Bradley Efron (BS '60), Statistician
As one of the key innovators in using bootstrapping methods in statistics, which is a method of assigning accuracy to an estimate. It is considered one of the revolutionary developments in modern statistics, lauded for its simplicity and ability to derive estimates for a broad range of data sets.
In the discussion below, Efron reflects on his undergraduate experience at Caltech, and the value that his mathematics education conferred for the span of his career. Efron went on to complete a PhD at Stanford 1964, and thereafter he joined the faculty and has been at Stanford ever since.
Efron's interests run the gamut from theory to application, and he brings his analytical skills to fields from biostatistics to astrophysics. One of the most eminent living statisticians and a member of the National Academy of Sciences, Efron has been the recipient of the MacArthur Prize, the National Medal of Science, and the International Prize in Statistics.
Interview Transcript
DAVID ZIERLER: This is David Zierler, Director of the Caltech Heritage Project. It is Monday, August 29, 2022. I am delighted to be here with Professor Bradley Efron. Brad, it's great to be with you. Thank you so much for joining me today.
BRADLEY EFRON: I'm glad to be here.
ZIERLER: To start, would you please tell me your title and institutional affiliation?
EFRON: I am Professor of Statistics, Emeritus at Stanford University. I guess I'm also emeritus from a department in the medical school, Biomedical Data Systems.
ZIERLER: What year did you go emeritus?
EFRON: Last year.
ZIERLER: Oh, brand new.
EFRON: My hearing was giving out. That was the main thing.
ZIERLER: Tell me a little bit about your affiliation with medical issues at Stanford.
EFRON: I got here in 1960 as a student, then assistant professor. While I was a student, I started participating in the medical school, consulting. My advisor, Rupert Miller, and senior fellow, Lincoln Moses, ran a weekly consulting workshop in the medical school, which had just moved down from San Francisco into the Stanford campus, and it was a wonderful experience working with them. It was much livelier, in a data sense, than the pure statistics I was getting over in the stat department. I was a half-time member of the medical school faculty for all those years, over 55 years. I'm not a doctor, and I'm not any good at medicine, but what I did and am doing is consult with people in the medical school. People run experiments, and they get a lot of data, and they're not trained to get the information out of the data. They're also not trained very well a lot of times to ask exactly the right questions. I work with medical researchers who are, by and large, smart, honest, hardworking people. Human medicine is a much more complicated subject than, say, quantum theory or something like that. It doesn't work that way, it just doesn't follow the rules the way it should. If complicated is a measure of how hard something is, medical research is about as hard as it comes. I worked on various kinds of leukemias and stuff like that early on, worked with very good researchers. 50 years ago, the experiments tended to be smaller, and the data less. It was harder work getting things done, but people were clever about it. One of the questions was, does it help cure leukemia, non-Hodgkin's kinds, to add radioactivity? They were going to put people at the end of a small linear accelerator, which sort of sounded crazy at that time, but that's the way they do it these days. I learned a lot, and it's always had a big effect on my research, and my general attitude towards statistics was always applications-oriented.
A Brief History of Statistics at Stanford
ZIERLER: Before we turn to your own research, you've been at Stanford so long, I wonder if you can talk a little bit about the development of statistics at the institutional level at Stanford over the decades.
EFRON: Sure. The last time they did a review, the Stanford Statistics Department was far and away the most successful department. Everybody in the country agreed it was the best department. And that wasn't an accident. The university, for some reason, has always been quite supportive of statistics, and it got going at Stanford after World War II. Maybe 10 years after that, it got really going. Herb Solomon was very well connected with the Naval Research Institute. It was during the time when the armed forces could still directly support research. They got quite a bit of money, and the University was forward-looking in having a statistics department. That wasn't so obvious. By the time I got there, it had a lot of momentum. It was probably the second-best department in the country when I got there, the best being Berkeley, where the famous Professor Neyman had collected wonderful researchers and sent some of them to Stanford. The trouble they had with the Loyalty Oath, which was a long time ago, sent Charles Stein to Stanford. Charles was the greatest mathematical statistics guy. When I got here, there were famous people here, and famous in that they really were good, not just that their names were in the paper. Chernoff, Stein, Manny Parzen, Rupert Miller. The department was a very exciting place to be. Subsequently, you can see from the point of view of a long time ago, it was the successful end of a long arc of mathematizing the field. And that was very good. It was really done at a high level. But it had its downside, which was that mathematized statistics wasn't what the world was calling for in terms of analyzing data. It was needed to put the field on a solid basis. But subsequently, for a long period of time now, there's been a movement to make the field more useful to people like the medical school, but everybody else, too. And that's been accelerated by computers and things like that. The field came up strongly, and the department didn't rest on its laurels. It's got new, good people who work in those areas.
ZIERLER: In what ways has statistics become more interdisciplinary throughout the years at Stanford? In other words, opportunities to collaborate with other departments or even schools.
EFRON: Statistics, by its nature, is interdisciplinary, though that's not exactly the right word. It's sort of super-disciplinary in that astronomers have stars, geologists have rocks, chemists have chemicals and stuff. There is no natural statistics. The raw material for statistics is what other scientists do, so it's naturally interdisciplinary. During all this time, more than half the members of the statistics department have had joint appointments with other departments. Math, computer science, electrical engineering, the medical school, things like that. It naturally mixes with other disciplines.
ZIERLER: I want to turn to your own areas of expertise. At the broadest level, if someone asks what kind of statistician you are, what's the most digestible answer, one that the man on the street can understand?
EFRON: Well, I can tell you what kind I'm not. I'm not the kind who does high math theory. I've been somewhat in the middle. If you made a social diagram, my own work, I've tried to make it as useful as possible, but that doesn't mean going around and working specifically with people in the medical school or astronomy. I mean in a general sense of doing stuff that people could use. I worked a lot on confidence intervals, families of models people might use, things like that. I'm not as applied. My colleagues, Rob Tibshirani, Trevor Hastie, and Jerry Freeman have worked much more with making statistics take advantage of the computer age. They've written books that have had great effect. I think Rob is the most-quoted mathematical scientist right now. He's got something like 300,000 citations. I rely on them to help me be more with-it when it comes to doing stuff that people would want in the computer age. But what they want in the computer age isn't necessarily just computing. I still get small datasets, and I still have to work on them. I have one right now with 480 people, patients in some medical thing, and they have nine kinds of symptoms. I'm looking at the relation between the symptoms and the people. That's not an enormous dataset. It's hard to weasel out the right idea. I try and work on stuff that's at least sort of useful. I'm publishing a book with Cambridge on exponential families, which are parametric families of distributions. Parametric meaning some kind of model. Before that, I did a book with Trevor Hastie called Computer Age Statistical Inference, which reviewed what had happened to the field since computers came in around 1950 or so. I'm sort of a general worker. I'm not as mathematical as the more mathematical guys in the department, and I'm not as applied as the more applied guys in the department. I'm somewhere in the middle.
ZIERLER: What about another binary on the spectrum in which you lie, the difference in statistics between an experimentalist and a theoretician? How does that work in your discipline?
EFRON: I like to say, physicists have a third category called phenomenologist. Phenomenologists are people who are supposed to take the theory and bring it over to the experimentalists. They're connecting links. I like to say I'm a phenomenologist. It makes me feel good to have a nice, long name like that.
The Bootstrap Approach
ZIERLER: It's such a long and varied research career. What are some of the themes or research questions that have always remained close to what's important to you?
EFRON: There are questions of accuracy. There are two main kinds of statistical questions, maybe three nowadays. I wrote a paper called Prediction, Estimation, and Attribution. It's obvious what prediction means. These days, it's big in the news. And I've worked with some of those things, but I have to go to my colleagues to talk intelligently about stuff like that. Estimation, you get some data, and you're interested in some number, but you don't see that number, and you have to estimate it somehow. That's when you get to things like how accurate an estimate is, how to get a confidence interval for an estimate. Attribution, you have a bunch of possible causes. In that example I gave, there were nine different things that were important, and you wonder which are actually important. I've been working more in the accuracy field. What's gotten me the most credit is the bootstrap, which is a method of assigning accuracy to an estimate. You go and get a whole bunch of data, and you have a parameter you're interested in, like the mean of some quantity. You can estimate it from the data, but how accurately are you estimating it? That's what I've worked on a lot. The exponential families I talked about are a meta-method for getting at that problem, and I've tried to get the theory of that to work what I'd call automatically. Some statistical methods are much easier to use than others. If you want to estimate something, one way is to try and find the best estimate, the one with the smallest variability, that's unbiased. Another way is to use maximum likelihood, try and choose the value that maximizes the likelihood of what you've seen. The first of those unbiased estimations is hard. You have to think of a new idea each time. Maximum likelihood is automatic. You can write one program that does it for everything. And I've been trying to make a theory of confidence intervals that is automatic, and it's become possible because of massive computing. It's unbelievable how fast the computers are now. Literally ten-million times faster than when I started, maybe more than that. And they're more than just fast, they're so convenient. The engineers have done such a wonderful job. This little thing I'm talking to you on is so amazing. When I was a kid, there were all these predictions about what the world of the future would look like, autogyros in the sky and stuff. Nobody ever predicted this stuff. It's just incredible.
ZIERLER: Have you ever pursued a career as a public intellectual? In other words, communicating the technical aspects of statistics to popular audiences so they can appreciate the role of statistics in sports, literature, healthcare, you name it.
EFRON: Now that I've retired, one of my projects is to write such a book. And I'm writing one called To Think Like a Statistician, and it's about how statisticians think. And, boy, it's hard to do. I'm not a natural friend of the public or something like that. My friend, Persi Diaconis, a good mathematician and statistician, is a natural at that kind of thing. I'm not. I sure wish I could write better, I'll tell you that.
ZIERLER: But you're pushing yourself in retirement. That's great. What have been some of the big debates in statistics, and where have you come out in some of those debates?
EFRON: There's a classical debate that's been going on for almost 200 years, which is between the Bayesians and the frequentists. It's basically a debate of whether, in approaching a new problem, like the one I told you about, you get to use past information or not. And it sounds like you should always use past information, but if you do, the question always comes up, which past information? People want to use as much information as possible, but they also want to not have their methods adulterated by opinion. The question of whether you can use opinion or not is important. The Bayesian theory, which goes back to 1763, is a very important way of using past information to update. The idea is, you start with some opinion, you get the data, and the Bayes theorem is a machine for updating your information. You had odds ten-to-one in favor of something before you started, then you got some contrary information, and now it's three-to-one. How do you do that? That's a wonderful theory, but in the 20th century, one person, R. A. Fisher in England showed that it was possible to have a non-Bayesian theory that was effective, and in some sense, optimal. The Frequentism works basically within the framework of any data you have, not bringing in what was past. The question I've always been very interested in is, what's the boundary between those two, and how can you get the advantage of both of them? And how can you make a Fisherian kind of answer mesh with the sometimes very reasonable Bayesian answers? The biggest back-and-forth over many years has been between Bayesians and frequentists. Frequentists, for the last 100 years, have been dominant, but not exclusively so. There are a lot of good reasons to do Bayesian research, especially in some areas where there is a lot of useful past information. If you're running a drug company, and you keep running tests on lots of similar drugs, each dataset doesn't have to stand by itself. That seems obvious. But it's also obvious that the Federal Drug Administration doesn't want you bringing your opinions into your data analysis. They don't believe them, or maybe they can't afford to. There's a lot of tension there. That's been the main back-and-forth. There's been another controversy–I don't want to say controversy…
ZIERLER: An academic controversy.
EFRON: It's between what these prediction algorithms, like deep learning and stuff like that, what they do and if it's enough. There was an article in the paper today, The New York Times, about a guy who works on that, and he said, "It's not enough. You don't understand anything. They're good at making point predictions, but you can't understand what the predictions are." Another controversy is, what are the roles of these machine-learning methods compared to classical statistics? How do they fit in? There's some controversy there about whether they're doing what you want to them to, if they've made classical statistics irrelevant or something like that. There's a lot of room for controversy, or at least uncertainty, in statistical arguments. Because as I said, there's no natural statistics in the universe. You can't compare your answer to something that's rock-solid from a measurement that you make. That makes the field difficult. After a while, you can see if something's working or not. One controversy has been about whether Bayesian answers tend to be biased. Biased in the sense that if I have 50 numbers I've gotten by looking at something, and I want their mean, taking their average is unbiased. If I have Bayesian information, it's not going to be unbiased in that sense. One question is, how much bias will people who work in the field stand? If you say, "In the long run, this is a great thing to do, but for your particular question, I'm estimating something with quite a bit of downward bias," people get nervous. That's been a question, what is it that we're doing? And needless to say, people work hard trying to get the answers there.
ZIERLER: As you alluded, there's been fantastical growth in computer power over the course of your career. In what way has that made your work more efficient, just as a tool, and in what way has it made your work different, simply that you can do things that otherwise would not be possible?
EFRON: When I started, there was almost no electronic computation. I had a desk calculator. I don't know if you've even seen one, but they're large, bulky, noisy things that take 15 seconds to multiply two numbers. And you were grateful to have them. When I started working over at the medical school, for example, I had Jerry Halpern, who worked here and was good with the computer. I'd give him an analysis to do, and he'd bring it back the next day, and we'd proceed like that because I couldn't do it. Now, I can do everything myself up to a point. I can go so much faster. I must be 100 times faster than I was before. Also, I can do much more ambitious analyses. The wonderful way the computer programs are available to do exotic things, but packaged in a way that I don't have to know the nuts and bolts of it. If I know what the idea is, I can use it. I go much faster now, and I'm much better. I don't have to rely on somebody else, I actually understand what's going on, and I can take it apart and do graphs. It was incredibly hard to do graphs and figures before. If you've ever tried to draw a curve doing the X's and Y's, it's not a natural thing. I'm a grade-B computer user, but that's a lot better than I used to be.
ZIERLER: Beyond academia, where have we seen the influence of your work out in the "real world," whether it's industry or government? What are some of the ideas you've had that have had an afterlife beyond your work on them?
EFRON: Well, I don't have 300,000 citations. I have a lot, but I've mainly worked inside statistics. If I was going to say what I do, I'd say it's connecting, and extending, somewhat, ideas inside statistics. But the bootstrap had a lot of influence in the sense that people use it a lot, and if I run into people in other fields, they're liable to say, "Oh, you're the bootstrap guy," or something like that. My colleagues I mentioned, Hastie, Tibshirani, and those guys, have had a big influence on how the machine learners do their work and stuff like that, and that's a really good thing. It's easy to overstate one's own influence on things. I like what I've done, but the man on the street is not going to benefit all that much, unless he lives on a very good street. [Laugh]
From Minnesota to Caltech
ZIERLER: Let's go back in history now to establish some context before you got to Caltech for your undergraduate. Growing up, were you always mathematically inclined? Did you naturally gravitate to that area?
EFRON: My dad, Miles, was a smart guy who didn't get to go to college much. He started back at one time, and he was a sales man, and he loved math, keeping score of baseball games. We'd sit around doing baseball scoring. I liked numbers when I was young, and I guess I thought I was a mathematician sort of. My high school St. Paul Central, a big high school, multiracial, as we'd say these days, and some pretty good teachers. I liked my geometry class and my trig class. There was no calculus at that point. I think I wanted to be a mathematician. I'd go to the St. Paul Public Library, check out math books, and not understand them but sort of hold them. That was pretty much how I got to it. I had an uncle when I moved to California, and he gave me a subscription to Time Magazine. At one point, on the cover, was Lee DuBridge, the president of Caltech. I followed one student, John Andelin, through his 80-hour-a-week schedule, trying to keep up with all the people at Caltech and stuff. I said, "That's for me." When you're 17, these things seem like a pretty good idea.
ZIERLER: I was going to ask you what your point of connection to Caltech was, how you even knew about it, it being so far from you.
EFRON: I got a really lucky break. The year I was graduating high school, they started the Merit Scholarship Program, and I won. At that point, it was a full four-year ride, and I applied to Caltech. And I'm sure they wouldn't have let me in, except for the scholarship. The guy came out. I forget his name. It was at a time when they sent a faculty member along to interview every prospective student. He came and told me I'd probably be a B- student, because they'd never had a student from St. Paul Central. This just encouraged me. "Oh, man, I can do it." [Laugh] I guess I used to be confident. That's how I got to Caltech. There was no other place I really wanted to go.
ZIERLER: What year did you arrive?
EFRON: 1956.
ZIERLER: What were your impressions when you first got to Pasadena?
EFRON: I took the train to Seattle because my older brother, Arthur, was there, and I took the train down. I was stunned. I'd never seen the ocean, I'd never seen a mountain. I woke up at 4 in the morning, and there was Mount Shasta going by. Then, we got to Oakland, actually, and at that point, you had to ferry across the Bay. I looked, and there was San Francisco across. I barely even knew about San Francisco. It was an incredible city. I thought, "Gee, if this is San Francisco, what's Los Angeles like?" We went down to Los Angeles, and I was picked up at Union Station by an upperclassman. His name was Brett. I wish I could remember his last name. I had never seen a freeway before. I got to Caltech, and it was pretty small. I liked that. I was horribly homesick for a few weeks, but then I acclimated. At Stanford these days, they tell the students how wonderful they are. That wasn't the attitude in those days. [Laugh] It was, "Look to the left. Look to the right. One of those students is going to be gone if you don't really work hard." Somehow, that didn't scare me. I don't know why. I started meeting smart people. Everybody was really smart. That was the first year, I think, that all the students admitted had 800s on both their math and verbal SAT scores. Everybody was a top student. And they really were smart people. The faculty was smart. But the students seemed smarter to me. Because I was closer to them probably. I joined Ricketts House, and I really liked having a bunch of friends around. And the people were so clever.
ZIERLER: Did you get involved in any of the pranks?
EFRON: Sort of as an aside. I'd see them doing the pranks. I remember Jim Sorenson was one of my classmates, he was a prank instigator. At one point, each of the four dorms had a standup piano in the lounge, and at one point, the pianos started disappearing, one-by-one. [Laugh] Eventually, they were all found in one student's room. I didn't see how he could sleep in the room, but somehow, he'd managed. He was doing it as a prank, of course. I thought that was about right.
ZIERLER: Was the plan from the beginning for you to pursue a degree in mathematics?
EFRON: I thought I was going to be a mathematician. It took me a few years to realize that even though I could get good grades, I wasn't a natural mathematician. I just didn't have the feeling for abstraction that modern mathematics has.
ZIERLER: And you mean pure mathematics.
EFRON: Yeah, that was the kind they taught. My co-student, Al Hales, wonderful mathematician who's had a wonderful career, I'd look at him and say, "No, I can't do that." Somehow, Galois fields seemed natural to Al. I began to get worried about it my junior year, that, "This doesn't seem to me like what I'm good at." One of my professors let me take a reading course in statistics. There were really no statistics courses. I got Harald Cramér's book, which is very old-fashioned by today's standards, but good book. Cramér was one of the leading guys. It was written while he was under house arrest, essentially, in Sweden during World War II. I read that book, and it made me want to be a statistician. It wasn't even clear there was such a field when I started on that.
ZIERLER: Why did this book speak to you in the way it did?
EFRON: The mathematics, by and large, seemed more realistic to me. There are sort of two main ways people convey mathematics, and one is the theorem-proof kind of approach, which is the way most textbooks present mathematics. If you're going to take a course in complex variables, that's how the book would go. But the trouble with the theorem-proof point of view, a theorem is a worst-case kind of entity. It says, "No matter how bad things are, what I say is still going to be true. No matter how bad you try and make them, given the restrictions I've put in the theorem." Worst case isn't great for applications. There's another way to teach or think about mathematics. Things like calculus or linear algebra are taught differently. You're sort of in the center of the field. You look at the things that work most of the time, like linear fit, or something like that. The mathematics of Cramér was more like that, and that appealed to my mind as the kind of thing I could do. I still have the book, it's sitting on the shelf behind me.
ZIERLER: Who were some of the professors at Caltech you became close with or were formative in your intellectual development?
EFRON: I hate to tell you this, but I was sort of a loner. I didn't get terribly close to most of them. I had people there who were very nice to me and stuff like that, but I didn't have intellectual heroes in the math department. If I had heroes, it would be guys like Linus Pauling, who taught the chemistry course. George Beadle. I'm sort of an introspective person when it comes to science. I don't think I took full advantage of what Caltech could've given me. Some of it was Caltech's fault. It was a small school, and they didn't have a statistics faculty, and they didn't really even have a computer science faculty at that point. It was just starting. And maybe that was good. I might've fallen into computer science otherwise. I liked some of my courses, but I didn't love them. Not long ago, I was going through some old stuff with my wife, and I found my transcript full of A's and A+'s of courses I couldn't even remember the names of. But I did learn something very valuable at Caltech, and that was the value of cleverness. They really taught that in the courses. The average test was an IQ test, and it wasn't a memorization test.
Caltech Cleverness
ZIERLER: What does cleverness mean to you?
EFRON: It means that you're given a problem, and it isn't a question of just slogging your way through it. You have to think of a way of finessing the difficulty of the problem by some insight that makes it easier. You took physics, the standard physics course at that time, Professor Strong taught it, and there were Strong problems. There were 100 problems that had levers and pulleys, and trains racing towards each other, and stuff like that. And each one had a trick in it. The idea that you don't make much progress by facing new problems head on by just slogging through, but you try to see what the weakness in the problem is and exploit it. That's a thing that's very Caltech. I've always felt I benefitted immensely being around people like that. Raised the game and definitely made me better at that sort of thing. I've been editors at magazines and journals, and an awful lot of the papers do slog through, and there's hardly ever a time you can just overwhelm something interesting. But there are a few people and papers that somehow manage to cleverly exploit a situation. And lo and behold, something happens. Ideas are the coin of the realm in science. Maybe I'm just saying it pays to have an idea, and an awful lot of papers are written with no real idea in mind.
ZIERLER: How did you come across the idea that statistics was a discipline that could be pursued in graduate school?
EFRON: A funny thing happened. I had my Cramér book, and that was obviously a statistics book. He said it was a statistics book, and I thought that was for me. It was time to apply to graduate schools, and I applied to Berkeley and to Stanford. I went up and made visits, and they both welcomed me. I needed, of course, a scholarship or fellowship. But Berkeley sent me a postcard saying I was in, and Stanford sent me a really nice letter, so I went to Stanford. And that determined half of my life. [Laugh] Incidentally, the two departments are still very close to each other. When I got to Stanford, I found that my recommendations from Caltech had said something like, "This guy is too smart to be a statistician. Put him in math," so I was admitted to the math department. It took me another year to actually get into the statistics department. I thought, "Oh, well. I'm a real good mathematician, and statistics should be easy." But it wasn't, it was hard. It's got a certain feeling to it. You can't mathematize the field. You have to have some feeling for where you're going. And that's what being trained as a statistician is about, learning the effects of ways to think about data analysis, how you might make tools that are good for data analysis.
ZIERLER: Was it always clear to you that you'd pursue graduate school, that you would not enter industry after undergraduate?
EFRON: I guess so. It was a time when a lot of the people who graduated from Caltech went to work in the defense industries. And there was money to be made, but somehow I never thought of it that way. When I look back, I'm surprised how young I was and how little I knew about the academic world, what that life would be like. Of course, I think I made the right choice, but maybe I'd be a billionaire now had I chosen differently. [Laugh] But yeah, somehow it seemed natural to go to graduate school. And maybe that was Caltech talking. Caltech really did have a wonderful intellectual atmosphere. By intellectual, I just mean smart. The people at Caltech are really smart. At least, that was my opinion when I was there, all up and down the line. Lee DuBridge was a very smart guy, a wonderful administrator. The various professors were obviously smart, the teaching assistants were smart, and the students were smarter still, I thought. I guess I felt I wouldn't be good at earning a living in the regular world. Maybe the academic world was right for me. [Laugh]
ZIERLER: When you got your bearings in graduate school at Stanford, what aspects of undergraduate training were really useful, and what were the areas you had to learn as a brand-new discipline?
EFRON: I had never taken a statistics course, where a lot of my fellow students had. I had to get that. But like I say, I had enough good mathematical training, so that wasn't going to scare me. I didn't have any real scientific background, and I didn't have a lot of good feeling for that, so that was difficult for me at first. You take a statistics course, and you learn about something like standard error. "Why did people choose that definition? What's so great about that?" Once again, I think what stood me in good stead was thinking more cleverly about things, and that was definitely Caltech stuff. I did feel at home in an academic environment, and I think I should give Caltech some credit for that, too. My actual home in Minnesota sort of disintegrated. My dad got sick and died. Caltech was my home, as far as I felt.
ZIERLER: In the way that, as an undergraduate, you never felt quite at home in mathematics, what is your distinct memory of feeling that statistics really was right for you, that intellectually, you did feel home in that discipline?
EFRON: I think it came more over at the medical school, where I was working on data-analysis problems with Rupert Miller and Lincoln Moses. When I'd feel like I really was being effective in analyzing data, and that my theoretical stuff–it's all sort of amazing to me that statistics actually does work. You think of these theories that seem maybe rather arbitrary, but you go out in the field and try them, and most of the time, something comes through. You actually do help doctors understand their data and stuff like that. I started feeling more of a mastery of the combination of what I was studying and how it might be used.
ZIERLER: Tell me about developing your thesis project. How did that process develop?
EFRON: Once again, it was me being sort of a contrarian. I just started putting together stuff on probability of geometric objects and things like that. There's a topic that goes back to the beginning of the 1900s called geometric probability. I got interested in that. It's random objects rather than points or numbers, things like circles and lines, stuff like that. I was very at home with that. I have a good geometric feeling for it. Maybe I should've listed that as the third way to do math. I started putting together things like that, and I worked one summer at SRI, working on the Perceptron, which was an early version of deep learning, and I proved a theorem about it. They wanted me to get my degree and get out of there, so I put together some four or five of these things, called Problems in Probability of a Geometric Nature. Some of them were published, and some weren't. It's nothing like I've ever done since then, but it did give me the feeling that I could do research and think of something that was new, not just finishing somebody else's idea.
ZIERLER: What was the process of determining who your thesis advisor would be?
EFRON: I'm beginning to realize that I've taken a rather strange path to sitting here today. When I finally got into the stat department, I found that the person who'd been at Caltech, whose name I'd heard, Sam Karlin, a major figure of the mathematical kind, was having a big fight with the rest of the stat department, and he was leaving to go to pure math. I'd started as his student, and I'd written a good problem for him, but he said that he wouldn't keep on with me unless I switched back to mathematics, and I wasn't going to do that. Rupert Miller was a young assistant professor at that point, wonderful man who died early. He was a very clear thinker. After a talk, whether I'd gone or not, I'd look at Rupert's notes, and they'd be very simple. A line here, a line there. But it would say just what the guy had done. Clear thinking is a wonderful thing. I wish I had more of it. I got to be Rupert's student, partly because of the connection with the medical school and working over there. Then, Herb Solomon, the guy who'd gotten all the money from the Naval Research Institute, was also a wonderful man. He helped support me and talked to me about things like geometric probability. I drifted over to Rupert, and he let me put together the thesis out of several things, including one part with my roommate at the time, Tom Cover, who'd come from MIT. I met him when I first came to Stanford. Tom was also a wonderful guy. We were roommates, and part of our theses was a joint topic. One of the reasons I felt more comfortable when I got to Stanford after a while was, I met some people in my dorm who turned out to be lifelong friends, Tom being one of them. Lou Padulo was another one. The dorm was a horrible place, but the friends were very good. That made me happy. I sort of got Rupert to be my advisor, and he was always helpful. Now, I've got my degree. Where am I going to go teach? A position opened up at Stanford, and I applied. Stanford has had a longstanding rule against hiring their own. I was listed as the third choice, and the first two turned it down. The first one was my Caltech colleague, Larry Brown, a very good statistician. The second one was a guy, John Bather, from England, who was also excellent. Thank God they turned it down, I didn't have to go back to the University of Minnesota, which was my next choice. There I was at Stanford still.
ZIERLER: What were the central conclusions of your thesis? What did you see as your contributions to the field at that point?
EFRON: I had no coherent view of it. I was still more of a mathematician than a statistician. I was still not really at my full natural strength that eventually I showed, long after that. However, I've always loved geometric probability. Nobody else does. It's a field that's almost nonexistent. But every once in a while, I'll take out my old book and look longingly at it. Here's a typical question. You have a normal distribution and a plane, X, Y. You draw several hundred points from that, and you try and draw the smallest polygon that will contain all the points. What's it's expected area? That was the kind of thing I worked on. That wasn't very useful, and I don't think about it much anymore, but I really liked doing it. Tom and I had fun working together on things. Tom went on to become the leading information theory guy in the world. Much missed. Everybody agreed, he was a fun guy. Fun guys are not so common in the statistics world. [Laugh] He was an engineer. He was really a fun guy.
ZIERLER: From being the third choice to joining the faculty, what did you feel were your prospects of tenure as an assistant professor?
EFRON: I never worried about it. After the thesis, I started getting my feet on the ground about statistics. I started writing pretty good stuff. I just assumed I was going to get tenure. I always worry about things, but never about the right things. [Laugh] I seemed to not worry about getting tenure when I should've. As a matter of fact, I got it pretty quick. Somehow, it seemed like my right. [Laugh] I don't know why I thought that. But I started doing good work, and I did have ideas. My friend, Carl Morris, who'd been my Caltech friend, then my Stanford PhD friend, then he went to Rand, we started working on stuff together. And it was good stuff. I started getting offers to go to other places and things like that. But I didn't want to go anywhere else.
ZIERLER: You were happy at Stanford.
EFRON: Yeah. Later, I was on some program where they gave me some support to do things for the University in general, and one thing I did was, I hired a very good-looking woman to ask a lot of faculty members why they were at Stanford. That worked well, they answered a lot. [Laugh] And I tried to analyze the data. "What do people like about Stanford?" I finally decided it was the absence of bad things. The weather's good, the department pays okay, housing was okay. They weren't the best at anything, but we had the least bad things. I've always liked being at Stanford. It's a well-run university, and like Caltech, it's wealthy. And, boy, that makes a difference in the United States.
ZIERLER: What was the research or project that really thrust you into the national spotlight, that resonated even beyond your immediate field?
EFRON: The only thing I've ever done that's really had outside resonance of any real size is the bootstrap. Before that, everything I did was connecting ideas within the field. R. A. Fisher, in his initial papers, had wonderful formulas that weren't well-understood, and I started understanding them in a geometric sense of curvatures and things like that. And that was the first thing I did that seemed to get attention. I started working with Carl Morris on what's called empirical Bayes. If you have a large dataset, the same kind of problem again and again, you can use Bayes's theorem without having to have prior opinions because the dataset itself can give you an idea of what the prior opinions should be. That's empirical Bayes. I'd done stuff like that. I guess people might've paid attention to it. But statistics is not one of those fields, or at least it wasn't one of those fields, that electrifies the population. It's hard to see what the effects are, even though it's very widely used. I would say about the only thing I've ever done that's put me in any kind of public spotlight was the bootstrap. I did one thing, however, that made me locally famous. I got kicked out of Stanford when I was a graduate student. I became editor of the local humor magazine, The Chaparral, and we had a Playboy parody. My Caltech background did not make it clear to me that some people took religion seriously. [Laugh] That was a mistake on my part. I got kicked out of Stanford, and I protested. They were always kicking out people. I protested. I was in the newspapers, I was denounced from the pulpit in San Francisco. For a couple weeks, I was really well-known. That's the only time I think I was ever well-known to the newspaper-reading public.
ZIERLER: What did you write that was so offensive to certain people?
EFRON: Quite a bit. It was actually a Playboy parody. They'd have what they'd call a Ribald Classic, where they would take some bit of classical literature that had something sort of sexy in it, and they'd write it up in a way that was more so, a few hundred words or something like that. I did a parody of that, which somehow unfortunately involved the bible. And I really wasn't trying to be sacrilegious, I was trying to be against Playboy. But that showed my lack of knowledge of the outside world, and I'll blame Caltech for that.
ZIERLER: For the last part of our talk, I want to ask a few questions retrospective of your career, then we'll end looking to the future. Obviously, you switched from math to statistics. What has stayed with you ever since, just in terms of your approach to numbers or thinking about scholarship, that you learned at Caltech?
EFRON: Again, I'll tell you that cleverness, having an idea and not just going bowling into the problem, is the only thing that ever works for me. Sometimes, I don't have an idea, and then it's hopeless. A lot of people in science and statistics don't really look carefully at what they're doing. They apply big methods. These days, it's easier to do with a computer, and out comes an answer. What happens if you change the methods a little? What happens if you change the data a little? I've gotten better at thinking about the connections between things. "I'm doing this method. Didn't I do something similar to that in another area not that long ago?" I'm better at connecting things than I used to be. I have a mental picture of fields. I was the dean for a while. I imagine a picture of a plane with an origin, lots of dots where things are known in the field. With physics, there's a big, strong cluster of stuff, a lot of dots near the center, where people really know things. With statistics, things are more diffuse. There's a center of the field that's well-known stuff, but there are points far away that haven't been connected. Right now, for example, those prediction algorithms are points far away that aren't well connected to the main field. I've always worked by trying to get connections between the things that might apply to any such problem. Sometimes that works, sometimes it doesn't. But I try and write papers that have some surprise in them. An awful lot of papers when I was the editor were surprise-free. They say what the problem is, say what they're going to do, and there's no surprise to them. You hear a lot of talks that way, too. I try and always have something people wouldn't have thought of easily. I've gotten older, and it's harder to do research now. Research is energy-intensive. I can't walk as fast or as far as I used to, and I can't think as well. Or I can still think, but I can't pursue the ideas as well as I used to. That makes me think there was a lot of energy involved, and maybe there was a time when I really could apply the force and push something through, not by brute force, but by knowing where to push.
ZIERLER: I wonder if you've ever thought about the role of luck in your career, the professional opportunities you had, being in the right place at the right time, and how you might interpret that through the eyes of statistics.
EFRON: Yeah, that's a meta question. [Laugh] I already told you about the Merit Scholarship. It was a lucky choice to go to Caltech. What I should've said about Caltech is that it did raise my game. I realized what it took to be a frontline thinker. It's sort of like playing in the NFL instead of at the college level. that was lucky. The bootstrap came out of Rupert Miller's work on the jackknife. Tukey was a famous character in statistics, wonderful researcher, and he co-invented something called the jackknife. A question of how well it worked was a way of getting plus or minus quantities for estimates. Rupert Miller worked on it and wrote a good paper called A Trustworthy Jackknife, and the bootstrap came right out of that. That was a lucky break, to be told what was an interesting problem. Going into statistics was a good choice for me. Maybe I would've been a good computer scientist, but I don't think so. I'm not really good at technical stuff that much. But I'm really glad I chose that field. That was lucky, too.
ZIERLER: Have you been an engaged alum of Caltech? Have you kept up with Caltech over the years?
EFRON: No. Stanford absorbed all my interests. Since I went to school there and stayed here, in some sense, that made a block between me and Caltech. I did go to the 50th reunion of my class, and that was really nice to do. Caltech seemed just the same as ever to me. Went to my old dorm, Ricketts House. Now that they admitted women, I thought to myself, "It's going to be a lot more civilized." No, it went the other way. [Laugh] I was grateful for that. It was fun walking around the campus again, remembering how much I liked that campus. It's at least twice as big now as it was then, maybe more. I used to walk around it all the time, into the areas around Altadena and stuff like that. I just love that area. One thing I like about the Big Bang theory is, it's at Caltech, and they actually pay attention to this. I'm very fond of that. But I haven't participated much. It sounds like maybe I do a lot for Stanford. I don't. I pretty much stay working. I don't contribute money or anything like that. But I'm very fond of Stanford, too.
ZIERLER: In reflecting on all of your contributions, what is it about the bootstrap method that allowed your research to break out of that academic mold and have influence in wider society? What's unique about that one research area?
EFRON: First of all, the problem's important. It's, how do you assign accuracy to a statistical estimate? The older theories require mathematical analysis, making models, and stuff like that. Bootstrap's almost automatic. In that sense, it's like the maximum likelihood. You can make one program that does it all the time. It's not hard to do. Once you do it and see how it works, it gives you a different feeling about what accuracy means, more down-to-earth, less theoretical. When you say that the percentage of Democrats is 54% plus or minus 3%, you get some feeling what that plus or minus 3% means. People like using it because it doesn't require elaborate statistical or mathematical calculations. It was originally a computationally intensive statistical method. I wrote a paper with Persi Diaconis for Scientific American on computationally intensive methods, and it was the first of those.
ZIERLER: Finally, last question, looking to the future, when you're writing your book, what is it that you want the audience to take away? What will an audience truly appreciate through the eyes of a statistician if your book does what you hope it does?
EFRON: Well, the book starts by talking about the fog of noise that lies between us and perceiving things as they really are in the world, how randomness and noise is such a problem in science. Why wasn't there science in the year 0? The Greeks didn't really have science, they had math. It's because it's hard to see the real world, and the statistical point of view on the world is that there's a smooth, nice truth lying under a very noisy surface. If you are able to use probability theory and other theories in an intelligent way, you can penetrate the fog and get at what's underneath there. Trying to understand just how random a lot of the things we see are. It came out during COVID, when people suddenly really wondered, "What percentage of people are dying from COVID?" It was a very noisy thing to try and figure out. It turned out, about 1% of the older people who got COVID died. If it had been 10%, it would've been a much different kind of time for the last two years. Trying to get people to understand something like Bayes' theorem–what does it mean? It's a wonderful way to approach a problem. "I have some opinion. How does my opinion change when I get new evidence?" Well, the math isn't hard, but you've got to know something about probability, and most people don't know anything about probability. Trying to just say that without writing a lot of equations out–there are no equations in the book. Trying to say, "You have a funny-shaped coin in your hand. What's its probability of heads? You flip it 10, 20, 100 times, it comes up maybe 65% of the time heads. Is there a real probability of heads hidden in that coin? What does that mean? How long would I have to go on before I knew that?" That kind of thing, how and if information is accruing. Can you get better answers if you get more and more data? Not always. Sometimes the noise has an irretrievable lower bound on it. Trying to see questions of accuracy. What does accuracy mean? What does plus or minus mean? How do you know if a new drug's better than the old drug? You run a clinical trial. How do you interpret the data in a clinical trial? That kind of thing. There are big philosophical questions like, "What is noise?" But it's hopeless to try and compete with the philosophers. The next level of questions is, "How can we practically use ideas to get a good picture of what's under the noise?" That's how statisticians think. "What's the Bell-shaped curve mean? Why is there such a thing?"
Randomness and Truth
ZIERLER: Is that to say, when you talk about the underlying truth and all of the randomness, are those compatible statements? In other words, is the randomness part of the underlying truth, or is it something to get through in order to see the underlying truth?
EFRON: People have answered that both ways. There are theories that say there is no underlying truth, there are only objective things you can do. One of the theories due to di Finetti says, "I don't know what the probability of that coin is. But having flipped it 100 times, I have a set of possibilities that are more or less reasonable, and that's the only real thing. You can make that set of possibilities smaller and smaller, but there's no real probability of heads of the coin." The more Plato kind of theory is, "No, there really is one, you just can't see it." It's sort of like there really is a circle, you just don't have a perfect circle in real life. As far as working with statistics in the real world, those questions are less important because you don't get down to that for most things. You'll be pretty happy if I can tell you approximately what your answer is and approximately how wrong you are. But you want that to be a good answer, not just one I made up. It's not just my authority that says that, there's a model. People have thought of very clever ways of stating the thing you just asked that make it possible to give reasonable answers. There's nothing like Einstein's theory of gravitation or something. You're not going to ever get that kind of perfection with statistical arguments. But strangely enough, you can make the statements realistic enough that a rational person will agree, "Yeah, that's pretty much what we have to decide from this set of data."
ZIERLER: And that's a pretty good standard to go by.
EFRON: Yeah.
ZIERLER: Brad, this has been an excellent conversation. I'm so glad we connected. I'd like to thank you so much.
[END]
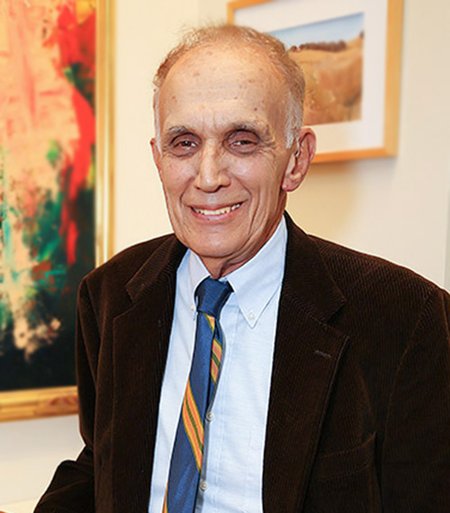
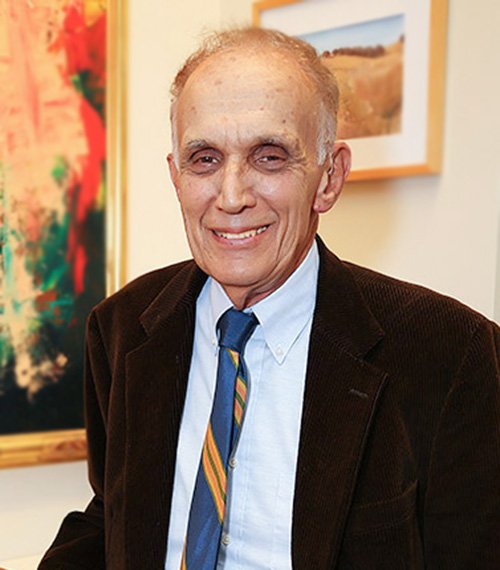