Daniel Crichton, Data Scientist and Computational Investigator
The major trend-line in science and engineering over the past quarter century is the broadening relevance of computation. Across the space sciences in particular - astrophysics and astronomy, planetary exploration, and radar-based Earth observation - the increasing power of computers, and the technological innovations that produce staggering amounts of data every day, pose opportunities for discovery, and challenges as they relate to data management and targeting techniques to find signals amid the noise.
As in so many areas, JPL has been a global leader in the field of computer science, machine learning, and complex data management precisely because its core missions demand it. Central to these developments is Dan Crichton, whose work over a broad range of science and engineering endeavors has demonstrated that cutting-edge computation methods are both an engine for discovery and a "force-multiplier" in expanding the science objectives of any given mission both within and beyond space science.
In the discussion below, Crichton reflects on the historical context of these developments, as superimposed against his own career in computer science and artificial intelligence. He explains how these advances have influenced promising new directions in disciplines far afield from space science, such as early cancer detection, and he sees a bright future for JPL, in large part because of the data management and analytical groundwork already set for the next generation of missions in pursuit of discovery.
Interview Transcript
DAVID ZIERLER: This is David Zierler, Director of the Caltech Heritage Project. It is Friday, February 10, 2023. I am delighted to be here with Daniel Crichton of JPL. Dan, it's great to be with you. Thanks for joining me today.
DANIEL CRICHTON: Oh, pleasure to be here. Thank you so much.
ZIERLER: To start, would you please tell me your title and affiliation within JPL?
CRICHTON: Yeah, I am a Program Manager, Principal Investigator and Principal Computer Scientist in the Center for Data Science and Technology.
ZIERLER: To give a sense of where that sits–JPL is so large and so complicated administratively–where are you within the larger universe of JPL?
CRICHTON: I predominantly sit in the Missions, Systems, and Operations Division in our Engineering and Science Directorate, but I also have appointments to the Earth Science and Technology Program Office, and that is to support technology development in the whole area of computer science and data science for the Lab.
ZIERLER: With one of your titles being Principal Investigator, is any of your work mission-specific?
CRICHTON: I've worked across missions, cross-cutting, but I also work on technology projects for NASA, and I've been a Principal Investigator for the last 20 years for the National Cancer Institute in helping to actually do methodology transfer between what we do in Earth and space sciences and particularly cancer biomarker research. A lot of the fundamental methods that we've been able to use and that we've made breakthroughs in, in areas like astrophysics, we've been able to actually bring into cancer research and do an infusion there.
ZIERLER: One of the larger stories that's so fascinating to me about JPL is how it's diversified its portfolio over the last 20 or 30 years beyond planetary science, into astrophysics, into Earth science, climate studies, and even biomedicine. How do you see your tenure at the Lab as a sort of microcosm of these developments?
CRICHTON: I think what I realized early in my career, probably around 2000, I was giving a talk at the National Academy of Sciences, is just the fact that a lot of the work we were doing to unlock use of data and data-driven methods in areas like space science, so both planetary science and astrophysics, had a direct link to other science challenges, other science domains, that were really struggling with the same kinds of data-analysis needs that we were making progress on at JPL/NASA and Caltech. And so, I think we were really pioneers in thinking about how to approach and start to work across disciplines.
AI From Biology to the National Defense
ZIERLER: Just as a snapshot in time, what are some of the current projects you're working on?
CRICHTON: I have a large project with the NIH in cancer biomarker research. I was actually involved and led the whole redevelopment of the NASA Planetary Data system, so that has every single bit of data captured from solar system exploration of the universe since the 60s. I led that, standardized the data system and the architecture, not only for the US but internationally. Every space agency that collaborates with NASA and with JPL uses our infrastructure standards for actually capturing and organizing planetary data. That was one of the major things that I've been involved in. Now, what I'm doing at the Laboratory is, I've really led, the last seven or eight years, the Laboratory's efforts to build a data-science and data-driven discovery program at the Laboratory.
We've been really reaching across all the major engineering science mission and institutional areas to really drive what we call data science in the fabric of the organization. We're looking at, "How do we raise the bar so that we can begin to really apply these data techniques to a variety of challenges and areas we have at JPL?" Really thinking about, "What is our future?" Because my view is that, as data is growing, and our advancement is continuing in areas like data-driven methods, we're going to provide whole new paradigms for the way in which we actually do our engineering science and mission activities.
ZIERLER: Obviously without getting into any sensitive details, in the way that JPL contributes to our national security, is there a data-science element where JPL is contributing with intelligence and things like that?
CRICHTON: There is. And in fact, we've got partnerships with the Department of Defense and other agencies, in particular to look at opportunities to also do methodology transfer between what we do at JPL and what we've done on our NASA missions into areas of the DOD. The DOD has very similar kinds of needs. They've got sensors to take observational measurements. We want to be able to take those measurements, and interpret them, and analyze them, so we can leverage a lot of the same kinds of techniques that we've been able to develop with astrophysics, planetary science, and other areas.
ZIERLER: Looking at your research agenda at a broad level, is it useful to think about what you're doing in either a terrestrial or non-terrestrial aspect, where you have space-based data science and then Earth-based data science?
CRICHTON: It is. And in fact, one of the areas I really try to lead at JPL and with NASA–and I've been pushing this with NASA–is to have us look–we've often talked about the fact that the way we're going to scale the whole observing system, because we're getting more and more compute power capabilities on the platform. At the same time, we've got instrument capabilities that are increasing the amount of data that they can generate and capture, so we're trying to do data analysis as close to we can to the point of collection. Pushing data science onboard, into these embedded environments, and then looking at how we bring that data back to Earth and building the whole ground infrastructure for computing to be able to support the analysis. And of course, the goal is to be able to move to more data-driven approaches, drive automation, be able to drive scalability, integrate multiple sensors, and really be able to look at this end-to-end.
ZIERLER: In all of JPL's achievements in astrophysics, astronomy, planetary science, what jumps out at you in the course of your career, the things that we've discovered about our solar system, even the universe, that's really been only possible as a result of machine learning and data science?
CRICHTON: I think there are a number of things that we can now do. And in particular, things like pattern recognition and things we're able to do in terms of things like nightly sky surveys and to be able to find transient events and other things that are in those images. If we went back 20, 30 years, we would maybe find 14, 15, 20, couple dozen types of events in these images. You could probably have a grad student take and circle those, and that was kind of the practice. But now, looking forward, we're getting millions of these and our observations. We've been able to really dramatically increase our ability to find much, much more fine-grained analysis and observations. That's really changing the way in which we can more intricately extract science from the data that we couldn't ever see before. We're finding correlations, patterns, and insights in the data that we haven't seen. In my area of cancer biomarkers, we're finding patterns of cancer biomarkers, particularly in imaging, so that's a fantastic overlap area with what we do because we can start to see space-time progression in cancer.
And what we're doing at NASA, a lot of the recent success we've had in landing on Mars with Mars Perseverance and what's been occurring has all been because we can push machine learning, data science, and artificial intelligence onto the platform now in a new way that we couldn't do before. For example, we can detect dust storms on the surface of Mars because we've got machine-learning agents running there. Really, running the model output, but we're actually able to train a model on the ground on what those dust devils look like, and then we are able to track those right there on the platform versus bringing all that imaging data back to the earth, trying to do the analysis, and then going back and missing the whole dust storm. We can begin to react very, very quickly. And that reaction, that event-driven approach, is so important, not only to how we're going to run missions in the future, but as you mentioned, to other agencies and other needs that we have in terms of really being able to use the power of data discovery right there at the platform.
ZIERLER: One of the most exciting developments that JPL is certainly going to be at the center of, if we ever find signs of life, either in our solar system or with bio-signatures or techno-signatures on an exoplanet, in either scenario, how will data science and machine learning play a role?
CRICHTON: Certainly, the discovery of exoplanets has been accelerated by data science. Our imaging capabilities and our ability to start to find those patterns of an exoplanet is something that, in the last few years, has been accelerated because we could do data science. I think that's certainly helping. And then, I think the other thing we want to be able to look for is, really, how do we find the right conditions for being able to sustain life? And I think data science is going to help us look at some of those patterns and really be able to detect what that would mean, give us a likelihood of whether we should go, follow up, and continue to do more science. And that's one of the ways in which we use data science, and I see this in biomedicine and medical science as well. It's not meant to always replace, it's meant to assist and guide. Giving us guidance of where we should be able to pursue future missions is certainly one of the things we want to be able to do.
ZIERLER: On the terrestrial side, how is machine learning and data science going to help with the all-important issue of climate change and sustainability?
CRICHTON: That's a great question. We've got a large, large number of activities going on in climate science and Earth observation. We just launched, in the last two months, a new satellite called SWOT. SWOT is a mission that's looking at surface water and surface water observations, trying to look at questions around hydrology and understanding where the water is in terms of the water cycle. That mission largely is going to generate as much data as we've been capturing in Earth science over the last 20 years. Big data and data science are really going to help us in terms of being able to understand and extract some of the insight from those datasets that are generated, but in addition, we're seeing an opportunity for us to be able to improve the way in which we do physics-based modeling and analysis for climate models with things like machine learning. Areas where we can begin to introduce data-driven methods alongside traditional physics-based methods is an opportunity for us to continue to add to and understand systems science and what's going on with climate models, areas where we can begin to use machine learning to add value to improving and running those models.
ZIERLER: Just a fun thought experiment, if you can think back to when you started at JPL and the computational abilities of that time, if you compare those advances during the course of your career, how might you extrapolate? What might you be aware of in terms of current computation to go, "In another 20 or 30 years, just imagine how primitive our current tools will be?" What's really lacking for you in terms of what you want to do?
CRICHTON: That's another really great question. I've been very focused on the data-intensive challenges and seeing opportunities with data over my career. And just to give you an example, back in 2001, we were concerned about how to deal with about four terabytes of data from a mission. The total volume of planetary data at that point was probably about 10 terabytes, and we would put all that data on physical media. It wasn't sitting on a large scalable network, cloud storage, things like that. Today, planetary science is on the order of about three petabytes of data. You realize how much we've captured in terms of that data. It's just incredible. A lot of that's been driven by imaging data. But as our communications increase, we can capture more and more data, because today, we can return about 1% of that imaging data we get from a planetary orbiter. Earth science, we've got hundreds of petabytes of data that's emerging, same with astrophysics. I believe we're moving into an era of being an exabyte science organization at NASA.
That means we need to be able to process and compute all that data. One other thing we didn't have years ago is the computing power to be able to really support things like machine learning the way we can do it today, deep learning methods, all those things which are very compute-intensive. What's changing is that we can now embed those capabilities onto things like cell phones and other kinds of computational devices. I believe that we are entering an era where we've got so much data and compute power that that's going to actually unlock a lot of new opportunities for discovery. And as we look forward, the thing that I really believe is going to be a game-changer is the ability for us at JPL to be able to put that compute power into space, and that's been one of the limitations because we tend to fly processors and memory there are inferior to what we have on our mobile phones today due to the radiation environment.
As we're able to actually scale that out, we're going to be able to do a whole lot more in terms of doing and scaling science out in the solar system. We're looking at things like whether we can build network-based sensors in cloud-computing environments and things like that, and put those computational devices around Mars, and we begin to support ways in which we can have networked sensors that can look at all kinds of observations and create sensor nets around Earth. I think what you're going to see is a whole new era of computation, which isn't just sitting in large servers at a cloud vendor, but really distributed out there in terms of what we do in space.
ZIERLER: Overall, in what ways is Caltech, having that affiliation and those partnerships really an asset for your work?
CRICHTON: It's been tremendous to have Caltech as a partner for us. What JPL is really excellent at is large-scale systems engineering. It's what we know how to do, to figure out how to be able to build these large systems. Caltech's really excellent at research. Put the two together, and we can go from fundamental research ideas all the way to deploying those into scalable environments. It makes us second to none in the entire world to be able to do that, bring those two together. And the kinds of use cases and challenges we have from JPL and NASA are phenomenal because we have the kinds of problems that really drive future needs in computation. As we work with Caltech, we've got the science, the research, the computational expertise, and we bring that together with JPL's system-engineering expertise, and I think we can really build world-class teams between the two sides.
ChatGPT in Historical Context
ZIERLER: Just a ripped-from-the-headlines kind of question, I'm sure you're following all of the media coverage about ChatGPT and Open AI. From your perspective, what are some of the advances in AI and machine learning that excite you, what give you cause for concern, and what might be some of the confusion, either in public perception or media narratives, about what's going on right now?
CRICHTON: There's not a day that goes by where you're not seeing a headline now about ChatGPT. [Laugh] In fact, yesterday, we just had a conversation with some of our sister NASA centers about ChatGPT, so we're all, of course, tracking that and tracking what things like generative models mean for our future, particularly for making them useful. I'm excited about what it can do. I think the concern that is out there is that it's trained on a set of data, and it doesn't always get the answer right. The question around trust in AI has often been a really large issue. With us at JPL, part of what we want to do is to put more AI capabilities on our spacecraft. That means we have to be able to trust what we put there, and we need to often be able to build that trust through what we would call explainability. Some of the challenge we have with machine learning in other areas is that an answer comes out, and it's difficult sometimes to explain why we got that answer.
There have been large efforts in explainability to try to improve the efficacy around certain kinds of results that come from, say, a machine-learning algorithm. I think one of the challenges we have with all of these AI capabilities is building confidence and determining how much to trust the result. There's a level of uncertainty, and at JPL, we tend to want to assign an uncertainty quantification to certain kinds of answers, which comes along with some of our inferences from things like machine learning and AI capabilities. I think that's probably the biggest challenge we have, building that trust. I think from the public perception, there's a very big difference between what we would say as generalizable or general AI and narrow AI.
Much of what we're talking about with machine learning is mathematics, statistics, something that we can do in terms of writing algorithms. As we get to things like general AI, that really cross that boundary, we're not there yet. But I think the question is, can we get there, and is ChatGPT beginning to lean us to a place where we can start to do more inference and be able to make more assertions about the data that we couldn't ever do before, much like the human mind? I think that's the challenge we have, seeing whether or not we can make that leap from more of a mathematics-statistics-based approach to one where we're truly able to generate and expand our knowledge.
ZIERLER: Looking way out into the future to sci-fi scenarios, do you see if we're on any kind of a trajectory that AI achieves self-awareness, or is that all a bunch of nonsense as far as you're concerned?
CRICHTON: I think the risk people are feeling with ChatGPT is that as we begin to use those kinds of techniques, they, themselves, are going to introduce their own biases. Because there are going to be answers, and those answers might feed other answers, and so forth. I was just reading an interesting article talking about how what we deal with on the internet, false information–you've got things on Twitter, Facebook, or other social media platforms where you're trying to regulate or figure out how you manage the propagation of information that might not be true. ChatGPT could actually generate, in some cases, false information that could get out there and then be used and continue to propagate. I think, looking to the future, part of the challenge we have is that as we rely more and more on these AI methods, the risk is that these AI methods may make a different determination than a human would make, and that's where we've got, I think, some real questions around ethics and if there are threats to mankind that could come from having too much dependence on an AI algorithm.
ZIERLER: Let's go back now and establish some narrative context. When you were a kid, were you always interested in computers and engineering?
CRICHTON: It's funny, I was 12 years old in 1982, and my dad decided to buy us an IBM PC. It was one of these 8086 computers with a floppy drive, no hard drive yet, and I started to play on that computer. I was writing BASIC code, and I started reading as many of the manuals as I could. I was actually teaching myself assembly language and those types of things. At 14, I had some connections already to JPL. I remember there were some entrepreneurs who hired me to actually port some software for them from CPM to MS-DOS. I was doing some things there, and then by the time I turned 17, as a junior in high school, I was actually working for a computer company out in Van Nuys. I was originally helping them build these early client server networks–the company built software for escrow companies–then helping them install software.
But I started debugging their code for them, and by the time I was a senior in high school and going to college, they asked me to take over and be one of their lead programmers. I went to college, they bought me a computer, they set me up where I lived with all I needed to be able to dial up on a 300 baud modem, send software back to them, but provide that support. I got a degree in computer science, was working for these companies when I was very young, and really, it was both my hobby and my job all the time to do this. It's been something that's been a passion of mine for as long as I can remember.
ZIERLER: When you were thinking about colleges, were you focused on programs and schools that had good computer science programs?
CRICHTON: I was, yeah. Absolutely, applied computer science as an undergraduate. I went to UC Irvine for my undergraduate degree in computer science. At the time, UCI was the only school in the western United States that had an independent school of computer science, so that's one of the reasons I chose to go there. It had a program that really interested me.
ZIERLER: What were some of the really cutting-edge ideas in computer science that you remember as an undergraduate?
CRICHTON: It's funny because I was learning things like Ada and object-oriented programming. We were moving from functional models to object-oriented-programming models. That was a shift at the time. They were trying to move us from Fortran and Pascal into things like Ada, which today, you wouldn't even use. But there was a real push there, I remember, at that time. And Unix was becoming more and more popular as an operating system. I was starting to work on their large server network at UC Irvine, and I remember being able to get and receive email that was just coming out right on that computer back in the late 80s with a few others who were around. Of course, this was all pre web browsers and what we call the internet today.
ZIERLER: When you graduated, did you want to go on to a master's program, to go into industry? What were your prospects at that point?
CRICHTON: I actually did want to go on and ended up going on to USC to get my master's in computer science. But I was still working for that original software company, and they had me stay on as a consultant. And then, I got a job working for Hughes Aircraft in Fullerton. Hughes was building a large-scale air-defense system for the Air Force that was going to be delivered to the Kingdom of Saudi Arabia in Desert Storm. I went to work for Hughes in Fullerton and actually had a fantastic time. It was a great learning experience for me. I really enjoyed it. I was doing real-time software programming and working in areas of Unix, writing a lot of their software.
And one of the things I did there, which was sort of novel at the time, they had all these typical software-engineering methodologies of how much you should allocate software to people at certain levels, things like that. And I had a few other colleagues who had just joined, and we began to simulate the entire system. We actually set up a simulation of it that ended up being used as a test bed for validating a lot of the software. It became the approach that Hughes used going forward for how they built software. They showed the Air Force, they showed it off. It was a fun experience for me because they gave me a lot of freedom to go off, try and do these simulations, and see what we could do, and we were successful.
ZIERLER: Working at Hughes right out of college must've been pretty good. Did you ever think about not pursuing a graduate degree and going full-in on industry, or did you get the sense that getting the master's degree would really take your career to the next level?
CRICHTON: I think I always wanted to get a master's degree because I thought it would take me to the next level, and I wanted to continue to learn more. But I was balancing trying to work full-time and go to graduate school, too. Hughes had a very good program, they were one of the major Southern California engineering firms that supported some distance-learning opportunities. They really made it possible for me to be able to take classes remotely, they'd have a courier take my homework in for me, and things like that.
ZIERLER: I'm curious if you had any interface with the Information Sciences Institute, MOSIS, and all of those developments when you were at USC.
CRICHTON: My connection to ISI came around the mid-2000s. I started going down there, and we can talk about what led me there. But that didn't happen for me until a little later in my career.
ZIERLER: Did you ever think about staying on for the PhD or even going the academic track?
CRICHTON: I did. In fact, I took classes so that I could continue on with the PhD if I wanted to do so. It's always been a tension for me because I expected I was going to go into industry to be a software engineer. That was my goal. What I didn't realize was how much I would love technology, research, and science. It was a real eye-opening experience for me to be able to work at JPL in some of these areas because it's just not what I expected to do.
Background in Distributed Systems
ZIERLER: Did you have a master's thesis, or did you come out of USC with a real area of expertise?
CRICHTON: I came out largely in areas of distributed systems. I've had a lot of interest in being able to build these highly distributed, decentralized software systems. I could replicate that. Because there were problems I was seeing all over at NASA. They let me finish my master's degree, focus on doing some research projects around that, and I ended up taking a couple case studies that I ended up using. And that ended up being quite useful for me in the years to come.
ZIERLER: During this time, of course, we see the widespread adoption of the internet, both at home and in business. How did it change things for you, and how did it change computer science?
CRICHTON: This was about 1995, 1996, and we were starting to go to Yahoo!, and Yahoo! had this taxonomy of things you could look at, and we were starting to get insights. I used that model to look at what we should be doing at NASA in terms of how we'd organize and get to our data. That was really what my research thesis was around, that problem. It really drove my thinking.
ZIERLER: How did the opportunity at JPL come about? Did they recruit at USC? Did you have a point of connection there?
CRICHTON: I'm a second-generation JPL-er. I was at Hughes, and my dad worked at JPL. I should've probably brought this up earlier. He started working on the Deep Space Network in 1970, worked on some of the Mariner missions and Voyager, and wrote one of the first versions of SeqGen, which is still used today. It stands for sequencing uplink commands to the spacecraft. And I remember as a kid that he would have one of these computers where we'd take our phone, stick our phone on the computer, and he would use that to dial into JPL. There was no monitor, it would print out a dot-matrix result on the computer, so you could see that there. And I remember him bringing one of these old, old dot-matrix computers home. He had written a program for I think PL1 at the time, because he was learning PL1, a software game. I was probably 8 or 9 years old, and I remember playing that. Even my family really had that influence as well.
ZIERLER: Did your dad help secure a position, did he introduce you to the right people?
CRICHTON: Yeah, I think much to his disappointment, he would say that he didn't have a whole lot of influence in me getting my job at JPL. I was at Hughes, and Hughes had identified me as being a strong candidate to go off and deliver the software system to Saudi Arabia, but I was not in any kind of situation to want to go live there for about two years. I looked at going on to other projects, but they were really holding onto me. I ended up seeing an ad for a job at JPL, and I applied for it, and they called me for an interview. Before he knew it, I had a job offer.
ZIERLER: That's amazing. Growing up, did you pay attention to Voyager and the planetary encounters?
CRICHTON: I did, yeah. I was at JPL when they launched Voyager 1. I can remember that. I always had an interest in JPL. In fact, when I was in junior high, I took science classes on Saturdays at Caltech. Some of my friends would go down there and take biological science or science classes they offered to young students who had interest in certain areas. I was very familiar with the Caltech-JPL environment.
ZIERLER: What was your first project or job when you got to JPL?
CRICHTON: I worked on something called the Alaska SAR Facility at the University of Alaska. They're monitoring all the Earth-science satellite missions there are making measurements over the polar region of the Earth. We built the ground system for capturing all that data, and I built a lot of the software that actually processed the data that was coming from the satellites.
ZIERLER: When did you first connect with people like George Djorgovski and Ashish Mahabal, recognizing their interest in merging data science and astronomy?
CRICHTON: My career took an interesting shift. Early at JPL, I ended up being one of the first people in the entire Laboratory to learn and promote the use of Java as a programming language. I ended up writing a proposal that got funded to develop a new way in which we should work with our science data, and that software that was developed was with a team in which I was the principal investigator. I was still early in my career, but that ended up opening a lot of doors for me to present new ideas on how we should be building software systems and thinking about data, which led to a paper I wrote that went to the National Academy, it led to our work with the NIH, it opened up doors to a number of different projects with other sponsors in other research activities.
We probably launched something like 15 projects out of that, and it became the baseline for how we did Earth-science missions for science-processing for many, many years. The software, which is called OODT, Object-Oriented Data Technology, ended up being JPL, and then NASA's first contribution as an open-source project to the Apache Software Foundation. We broke the glass ceiling to bring NASA and JPL into the open-source world, to thinking about how we should be working, thinking about our data, and things like that. All that work I did had me connect with people like Rich Doyle, who was here at JPL, who was kind of leading some of our computer science activities.
Rich and I became very well-connected. And Rich had been working with George Djorgovski on some work doing a classification of features in a sky survey, things like SKICAT and so forth, in the late 90s and early 2000s. Rich, George, and I ended up creating a relationship. Then, Ashish was with George, and I got to know Ashish. He began to then work with me on some of our NIH-related work as well. We were looking at how to do methodology transfer between astronomy and cancer.
Connecting with Data Driven Astronomy
ZIERLER: Do you remember the earliest conversations? Was it George and Ashish who were aware of your work, and they approached you? Did you want to get involved in Caltech astronomy? How did that actually come about?
CRICHTON: We started doing some workshops and so forth together between our organizations, and that was, I think, an opportunity for us to start to try and look at ways in which JPL and Caltech could work together. We started having ongoing conversations–this was about 2012, 2013–in which we started to recognize, "Wait a second, we've all known each other, but we've got to really start to formalize a relationship." George, on the Caltech side, started the Center for Data-Driven Discovery, and on the JPL side, I started the Center for Data Science and Technology. Then, we wrote a joint MOU to connect the two and said, "We ought to start to look at how we can share people, share funding, create a more integrated joint center between Caltech and JPL, where we can go back and forth." And we thought this was innovative in a way because it supports that concept of going from research all the way to a scalable, usable system on these fantastic use cases that we have at JPL.
ZIERLER: I'm curious if you found yourself at this moment doing some level of evangelizing to the larger constellation of researchers at JPL just in terms of what these tools were capable of doing, that they weren't just niche products, but could be applied so widely.
CRICHTON: What I found as a technologist, and what I found as a skill I probably didn't realize I had, is that I'm pretty good at evangelism. [Laugh] And I had to be. I tend to think five years ahead of everybody else around me in my field, and so I'm often trying to influence the thinking of my senior leadership and others around me to really move us forward and push on trying to be more innovative and so forth. We had already been involved heavily in the data activities that were going on, and I could just see the trends and where things were moving. I should say, too, that prior to George and I meeting, we had some very parallel activities. George was heavily involved in the astrophysics community, standing up things like the National Virtual Observatory, the International Virtual Observatory Alliance. These were internationalizing things. And I was heavily involved in the planetary community, standing up the US Planetary Data System, and then the International Planetary Data Alliance, very similar kind of thing to IVOA. We started connecting because our intersection of computing and science in planetary and astronomy were starting to come together as well.
ZIERLER: Now, in 2000-2001, the transition from Ed Stone to Charles Elachi as director of JPL, did that change things for you, where you sat? Did that reverberate in terms of some new directions the Lab began to go in when Elachi started?
CRICHTON: Elachi was, I think, one of the best leaders we had at JPL. He was very strong in terms of trying to set and push the Laboratory forward. What accelerated my career in some ways was, back around 2005, I had been doing technology development, but there was a real crisis associated with how we were going to build the next generation of this thing called the Planetary Data System. There were some relationship issues that had emerged between JPL and NASA and some of our science community, and we needed a vision forward on how we were going to move from about 10 terabytes of data to what we have today. Through a series of events, I ended up being asked to step into this position, and Elachi had a lot to do with that, and he said, "I want to get this right, and I want to make sure we're committed to it."
I had strong support from him because he they wanted JPL to be a leader in what we were going to do with all this data from planetary science and to make sure we were leading how we were going to develop the next generation of computing capabilities for not just JPL or NASA, but for the world, really. It gave me a lot of opportunity to really push forward. I was organizationally moved out and up, and I worked for our director of planetary science, Chris Jones. And they gave me the latitude to rebuild it and really put that together. That launched my career in a lot of ways because I was really able to demonstrate the power of what we wanted to do with data within that program.
ZIERLER: To go back in the chronology, you said that your interface with the ISI really came in the early 2000s. What was going on at that point?
CRICHTON: As I mentioned, I started building this data science, open-source capability in the late 1990s, early 2000s that really provided a foundation for us to start working with our data. That was how I got into planetary and got into NIH. And there was a big push at the time for things like data grid technologies, computational grids, things like that, where you brought massive computers together to compute on them. I was building data grids. There were some folks like Carl Kesselman and others down at ISI who were sort of the fathers of grid computing and really pushing it. We ended up building a partnership and some collaborations with ISI, just getting to know some of the folks down there. And in fact, we've had really good follow-on conversations that continued with them and in particular, people like Yolanda Gil, who leads the data-science activities at USC now.
ZIERLER: At Caltech, with the rise of Astro-Informatics and the development of the National Virtual Observatory right around the turn of the century, what was your contribution, and institutionally, how was JPL helping make these developments possible?
CRICHTON: JPL was definitely part of the whole National Virtual Observatory, what was happening to internationalize and connect our astronomy centers together. I was involved at that point more as a technologist and software developer in the late 90s, early 2000s. And I think what JPL did in the late 90s, through people like Usama Fayyad and others who were here–who went on to Microsoft Research and then eventually became the chief data officer of Yahoo! and so fort–is that we demonstrated, even then, the power of things like machine-learning algorithms to do classification of some of the astronomical features. And I think that coupled with this need to start to build these national data infrastructures that would connect the data together really was groundbreaking. Because what you see 20 years later is, a lot of other science areas are now replicating what astronomy was doing back then. They realize, "We've got to look at building these data grids that connect our data together. We can start to bring in areas like machine learning for analysis," and so forth. Astronomy really, I think, provided a pathway forward for all of science.
ZIERLER: If we can get into some level of technical detail from your perspective, as I'm understanding and building out this story, these large-scale sky surveys are producing an inordinate amount of data, and the challenge, of course, is to find the signal in the noise. As they're coming up with all of this data, where do you get involved? Are you part of that process from the beginning? Does it come to you after the fact? What does that look like in real time?
CRICHTON: A lot of the work I've done has been focused on how to build the real-time systems that take the data in and then do the classification of that data. We get involved in building the whole system itself, and then integrating the computation that run those algorithms. My partnership with George and Ashish has been, I tend to bring a very strong architectural and systems element to how you build all these capabilities, then I work with them to build these multidisciplinary teams and integrate their algorithms. They're doing things like, "Can we take and identify bogus and non-bogus features in our images? We're trying to classify them. Are they an anomaly or real? Can we understand what this is?" Things like that. I tend to work with them to take and integrate their capabilities into the larger system, and that's been kind of the focus I've had all along with George, Ashish, and others.
ZIERLER: To clarify, when they're coming to you with this data, are you using existing programs, are these bespoke programs specific to the needs of the sky surveys?
CRICHTON: The breakthrough that my team made and that contribute I think I ended up making was building this capability called OODT. OODT was probably one of the first systems generic enough that we could take and deploy it across a number of different science areas, and we didn't have to rewrite the software every time. We could plug in the algorithms. The whole concept was, "Wait a second, we see the same kinds of problems over, and over, and over again. Can we create the framework, the car, and put in different engines, different components, without rebuilding the software system every time?" We've been able to standardize in a lot of ways, commoditize, how we do that, and that was really groundbreaking for ensuring we could actually build these scalable kinds of capabilities.
Computational Partnership From Lab to Campus
ZIERLER: This is only going to seem obvious after you answer the question, but why would JPL have these capabilities that Caltech would not have? Obviously, it's JPL and Caltech in partnership, but why would this not be a purely Caltech-campus, in-house kind of analytical endeavor?
CRICHTON: It goes back to that discussion that Caltech's phenomenal at research, and JPL's phenomenal at systems. You want to put the two together. The research side of the question is, how do we develop the methodologies and research capabilities to identify things we've never seen before? They're very, very good at building the algorithms and thinking about the question. The JPL side is, we're very, very good at building the systems that take the measurements and run those algorithms. We're putting the two pieces together and creating the solution by bringing in the research elements that are doing the detection with the systems that actually can execute, store, and work with all that data.
ZIERLER: Obviously, you're not an astronomer, but in building the algorithms, how do you know how to create them so that they find the interesting stuff in the data? What does that look like?
CRICHTON: In a lot of ways, we need to have training sets. Those training sets are things that we use to train algorithms. When you look at things like machine learning, we want to be able to train our machine-learning algorithms on enough data that we can then go back and validate that they're working as we expect, and there are ways in which we can do that validation. What we end up doing with folks like George and Ashish is making sure that we can capture and build these training sets that train the algorithms, then we run those algorithms on the real data that's coming through. One of the great things about astronomy has been–pretty recently–that we've got a lot more data. We can really use data-driven methods. You go to areas like biology, that's tougher because it's fraught with regulation, what data you can share, we've got limited data samples, and so forth. Trying to use and apply machine learning in the same way we've done in astronomy is difficult. However, if we can take an astronomy algorithm that's worked for detecting certain features in astronomy and transfer it over, do more transferred learning, that's incredible because then, we can begin to train on data across disciplines.
ZIERLER: As you were getting involved in this, did that necessitate new capabilities for JPL, either in processing power or storage? What were some of those conversations like?
CRICHTON: Definitely. Part of what really occurred over the last 20 years is, areas like machine learning and data-driven discovery have moved from being research methods to really being operational methods. We had to look at, "How do we operationalize and bring those kinds of capabilities into mission systems operations, in how we actually run it and execute a mission?" What I see going forward is that we're going to continue to have to look at how we deploy these capabilities more and more into our mission systems, and that's going to continue to necessitate new computing technologies and capabilities, particularly onboard. What we've had to develop as a capability is a way in which we can integrate those kinds of methods into our traditional mission systems.
ZIERLER: Just to keep up with the sequencing, when you and George, JPL and Caltech, are finding something interesting, what happens then? What do you do with that interesting piece of data?
CRICHTON: What's really happening, and it's very similar to when you think about Google, or do a Google search, or whatnot, you want to be able to identify and index that interesting information, and then you want to be able to capture it. We capture it as metadata. Then, we want to be able to feed it to discovery methods. Somebody comes in, and they're searching for some feature, say, a landmark on Mars, you want to be able to find those features. We're running the algorithms over, and over, and over again, and we're expanding the knowledge base, the metadata we have that describes those features so that when you come back, you can find them and capture them. What's happening now is, we're using things like machine learning to really enhance and provide ancillary information about our data itself so that we can use that to search that data.
ZIERLER: Where do the big telescopes come into play? In other words, if we can liken the sky surveys to buckshot and big telescopes like Hubble to a sniper rifle, what is the mode of transfer from these huge surveys, sifting all the data, finding interesting signals, and then using those signals so that the high-powered telescopes can really focus in? What's your role in that sequence of events?
CRICHTON: As I was saying earlier, the organizational role that I'm in is really building all the ground software that would process all that science telemetry data that comes in. We deal with the petabytes of data that flows through all these systems and then run those algorithms. In a lot of cases, every bit of data that comes through in software systems that I either have helped develop or colleagues that followed similar techniques that we helped pioneer years ago are developing today, which take all that data, try to organize it, and then run the analysis. JPL ends up working with the science teams and scientists to figure out how to prepare and what analysis to do on the data when it comes through our ground systems, then how to work with the worldwide community so they can use all the data that's coming from these observatories. Then, of course, what George wanted to do was connect all of that data together, so you've got an observing-system telescope that is even more powerful because it's connected not only to one, but it's connected telescopes around the world together.
ZIERLER: In thinking about developing software systems, tell me about how the Enterprise Architect Project got started in the early 2000s.
CRICHTON: One of the big shifts at JPL is recognizing that our missions have become more and more dependent on software. As an organization, JPL probably looks more like Google than it may even realize, or maybe more like Apple because Apple has hardware. But our devices are all driven by software, so software is onboard our Mars rovers, we're flying basically computers when we build orbiters, it's in all of our ground systems, and so forth. There's been an increasing interest at JPL, even back in the early 2000s, in how to start to build better software and how to begin to raise the role of computer science at JPL, recognizing that we're more and more dependent on software that is going to define our future. Software is what controls our spacecraft, it's what's controlling our communications, it's what's helping us do our science, so it's very important that we organize and provide standardization and structure in terms of how we connect and integrate all of our software systems together.
ZIERLER: When commercial ventures like Blue Origin and SpaceX really got going, the challenges this posed for JPL, did that affect you, did that affect recruitment, did you ever think about leaving JPL?
CRICHTON: It's interesting, the world has shifted a lot, and if we were to start JPL today, there would probably be a lot of different decisions we would make as a startup versus 60, 70, even more years ago when JPL was founded. Areas like SpaceX, Blue Origin, Planet Labs, and so forth, much of what they've built from the ground connects well to the vision I feel like I've had, that what we do is all about the data, and computing and software defines a lot of that. We may have a science-driven focus, but it's the software and the data that really helps us enable and support that discovery. When I talk to colleagues at Planet, or at SpaceX, or at Blue Origin, they're really pursuing a lot of those core capabilities these days, and they've really built things from the ground up, whereas JPL has to turn its ship to be able to really adopt these things because we've got a lot of heritage and legacy that's in place, and that's why we've got a lot of these initiatives and things like that.
Sometimes, the opportunity to go off and jump into an environment where they've started that way is attractive versus trying to figure out, "How do we update JPL and move JPL in that direction?" But what keeps me here is my own personal interest and love for what we do, which is just unique. None of these other organizations have the mission we have at JPL, which is given to us by the US to be able to be this lead center, and really, this lead center in the world, to do this robotic inspiration and have the connection to Caltech and NASA. That's really what keeps me here, being in the middle of that relationship.
ZIERLER: In 2004, when you were named Principal Computer Scientist, how did that change your day-to-day?
CRICHTON: I think for me, it was a recognition of a couple things. I needed to be a laboratory leader in terms of my area of expertise, which was really distributed systems and data-intensive systems. As we've been pivoting this way, it's become more and more important that I had to step up and really help lead the way. Nobody else was going to lead in that. That was a recognition for me, that I needed to do that.
Institutional Support for AI Research
ZIERLER: Who were some higher-ups at JPL that shared this vision and gave you the resources you needed to get JPL where you saw it needed to go?
CRICHTON: Early in my career, I had a lot of support from my section manager at the time when I joined JPL, and then he became the CIO, Tom Renfrow. And Tom was a strong advocate of computer science and software, and he saw in me that I possessed a lot of skills he felt were important to the future of JPL. He was very supportive of meeting with me, talking with me about my career, and helping to discern questions about what we should do, and even providing resources for that support. When I took over the Planetary Data System activities for Engineering in 2005 to really build the next generation, Chris Jones, who's our director of planetary science, was a huge champion and really supportive to make sure I could move our vision forward and so forth.
And then, over the last about 10 years, our deputy director, Larry James, has just been a phenomenal champion of what we're doing. His motto is, "It's all about the data." And he recognizes that data and software really are the future of the Laboratory. He's really been a champion in providing support. And as we were trying to grow data science and machine learning at JPL, he really advocated to get us funding to run various pilots. We've done probably 80 pilots in these areas over the seven, eight years.
ZIERLER: In 2005, 2006, as you got more involved in planetary science, what was going on with the world of missions that may have necessitated a more intensive data-science approach?
CRICHTON: Mars Reconnaissance Orbiter, MRO, came on the scene, which had an instrument called HiRISE, which is a high-definition camera. And HiRISE was going to generate so much data, probably about 20 times the amount of data we'd already captured in the previous 40 years of exploration. It was a forcing function that required an architectural shift in how we were building missions, which meant we had to look at new ways, new software techniques and data-storage techniques to address the increasing volume of data that we were going to get. And so, MRO was really a game-changer that required us to rebuild the planetary data system for the next era.
ZIERLER: Now, is this where the Planetary Data System was born, or that's separate?
CRICHTON: The Planetary Data System was actually founded in the 80s, came out of a National Academy study that basically said that the US was spending hundreds of millions to billions of dollars collecting data all over our solar system, and we weren't doing a good enough job of making sure we were preserving and capturing that data. The requirement was to archive and keep all that data. What emerged out of that was not just an archive, but we had to be able to disseminate it to the science community for use. The Planetary Data System was born in the 80s and the 90s, but the problem was that by about the early 2000s, with some of the technology activities I was doing, they were reaching a technology barrier that was not going to let them continue on the same approaches. They had to shift the way the Planetary Data System was being implemented and operated, and that's where I came in.
ZIERLER: In your role as Principal Computer Scientist, do you have peers at other FFRDCs that are in similar roles that you're interfacing with?
CRICHTON: Yeah, absolutely. In fact, I've got peers at both other FFRDCs and at NASA that I tend to regularly interface with that really, I think–we know each other, we're in shared conferences with each other, we actually write proposals together, we push on some of our technology needs together, and so forth, and that's been absolutely fundamentally important because we all exist in these similar kinds of cultures, and we need to be able to determine a road map forward and what capabilities we need to bring into our institutions to make sure we stay at the forefront.
ZIERLER: You mentioned, of course, collaboration. The flip side of that is competition. In the way that Ames, Goddard, and JPL have their areas of strength, how does that work in competing for grants or resources in terms of really leaning on what JPL is good at?
CRICHTON: JPL largely operates on soft money. We have a number of our research, computing, technology staff that write proposals, write grants. And part of the role I was playing was, back in the early 2000s, a recognition that this whole area of data-intensive and data-driven computing was something that we had to make sure we were leading and driving forward. Because I felt that the paradigm shift was going to happen. In some ways, that means we're competing because we feel a need and a responsibility, as an FFRDC, to make sure we're leading the way where we can for NASA. We've made our own investments, we've gone off and pursued technology funding. One of the reasons we go to non-NASA sponsors is, sometimes we need more funding than NASA can provide us, and we need to provide areas that we need to increase our competency and capability, so we go off to those non-NASA areas as well to do that. And that's helped us quite a bit. And we end up also working with our NASA colleagues to make sure we're part of trying to collaborate where NASA is going, but we also certainly have a level of competition because there are NASA grants and NASA opportunities in my area that we can go after to advance our technology capabilities and computing.
ZIERLER: You mentioned working with NASA colleagues, having peers at NASA. What are the kinds of issues that might pull you to Washington D.C. where you really need a face-to-face meeting?
CRICHTON: I spent a lot of years on airplanes going to Washington. [Laugh] And part of the need is that you need the advocacy of your senior leaders and those that have the funding at NASA headquarters. Part of the evangelism requirement is that we need them to understand where we're going and the need to fund certain capabilities, and we need to lay the seeds for that so that that funding can be put in place. And sometimes the lead time for that is a couple years. We're often going out to Washington, doing some initial activities, but beginning to educate them, saying, "Here's where we're headed," and so forth, making sure they're aware what JPL is doing so that we can have an influence on the direction of NASA. We often see ourselves as NASA's FFRDC as one that needs to help define the road map. We do that in partnership. And part of what we want to do is make sure there's a shared road map around data, data science, computing that really is in place so that we can influence the future capabilities of our missions and how we set up our science analysis.
ZIERLER: Advocating in Washington D.C., what about the Department of Energy and all of its work with supercomputing, national labs, places like Oak Ridge? Are you working in that space as well?
CRICHTON: We are. Another project I've been involved in is, back around 2008, 2009, I picked up another role in helping define our programs and direction for technology and Earth science. It was the Earth Science Data Systems and Technology Office. And one of the areas we really wanted to look at was, "How do we take our observational data we get from Earth science, space-borne missions, airborne missions, and in situ sensors, and how do we integrate those observations with climate models so we begin to validate and improve our climate models?" We started working with Lawrence Livermore National Labs and others who are pretty strong in climate modeling. They set up an infrastructure called the Earth System Grid Federation. The whole idea was, very similar to astronomy, where they're sharing astronomy data, to begin to share climate modeling data and observational data. We worked on this NASA/DOE partnership to look at how we could bring all our observational data in with all the climate model activities they were doing to make sure that was available to the whole research community. And that's, I think, the de facto system for the entire world that we ended up working with. It goes into a lot of the IPCC reports, so when they create these various assessments, they use the Earth system grid as the baseline system for that modeling effort.
AI Inputs for Climate Change
ZIERLER: In climate science, there are so many debates, some political, some scientific, about what's cyclical and natural, what's human-caused. From your perspective, how does the data science, the machine-learning aspect, add clarity to some of those questions?
CRICHTON: That's a good question because we've actually had this discussion at JPL. Where's the line? What's the role of JPL? Because there certainly is also a whole political discussion and other kinds of discussions that go on. Our role is, really, in terms of providing information. It's providing the measurements and being able to provide interpretations of measurements. What we're not trying to do is make recommendations on policy. We provide information to the worldwide science community. We also provide it to what we call the applications or decision support community that may want to make policy. But JPL, as an institution, has been very focused on the delivery of science and information as our baseline charge of our institution.
ZIERLER: Without asking you to make a political statement, from looking at all of this data, what are you seeing? What are some of the obvious trend lines?
CRICHTON: I think what we see is a very interesting variability in trends and cycles. I think our models are getting better and better, which is good. One of the interesting things we saw in COVID, I was part of a project, and we began to realize that people aren't driving, we had less transportation going on, and that was shifting our climate again. We were trying to get aircraft up to be able to make measurements and analyze what things look like. We began to see that the human component really has a major impact on our climate. I think that was one of the most interesting things to me. We began to see certain things over certain regions of the Earth begin to shift in terms of increased water clarity, less pollution in places, things like that. I think that's interesting.
The other thing we're seeing is just a lot more advancement in us being able to do better and better predictive analytics, so we're trying to get better at looking at things, like we did a machine-learning project that was trying to look at hurricanes, rapid intensity and trajectory hurricanes, to see if we could predict them and so forth. And we found that machine learning was giving a better prediction than traditional statistical approaches that had been used in the past, and we've written papers on some of those results. What we're now seeing is the fact that we can put machine learning into aircraft and onto satellites and start to do more analysis of things around methane or other areas we want to measure.
We're seeing the ability to do more rapid response as a way forward. Another possible opportunity is, we've had some discussions and collaborations with the XPrize Foundation and others that want to be able to start to look at some technology advancements. Could we identify within, say, this 1,000-square-kilometer area whether or not there's going to be a fire? Is there smoke? Can we begin to react? Can we put it out? Things like that. We're seeing our whole monitoring activity really become connected to event detection and then response in terms of where we want to go.
ZIERLER: We've covered some important Lab leaders, from section manager all the way to director. Two positions I wanted to ask you about were chief scientist and chief technologist. Either by the individuals or the offices what their roles are within JPL, what's been most important for you over the years?
CRICHTON: Those have been key roles for us at JPL. I think what I've seen on both sides is an increasing recognition by both of those roles that the areas I represent, software, data, and computing, are becoming more and more critical to the future of JPL. On the technology side, our technology road maps released, certainly the last few years by our chief technologist and his office, have emphasized a recognition that areas like autonomy are increasing, recognition of data and data science, recognition of machine learning and software computing. They're all in the top five areas and technologies that JPL feels are important to the future. They very much have been advocates helping in this evangelism and the investments into where we need to go at JPL. On the chief scientist side, and certainly, Professor George Djorgovski represents this, there's really a growing recognition that data and data science are fundamental to the future of science analysis.
And you see a shift occurring, which is that not only are we publishing papers today, but we're required to publish the data that went into the paper. And now, we're required to publish the software that was used to analyze the data in the paper. My world and the science world have been coming closer together, and I almost routinely now have scientists embedded on my projects that support and provide the domain expertise we need for helping to ensure that we understand the science well enough to build the software in the right way.
ZIERLER: This emphasis on open access, on publishing everything, in what ways has this been good for the democratization of data science, that maybe you don't need to be at an elite place like a Caltech or a JPL to access this information and to make a contribution?
CRICHTON: From my perspective, it's really been a journey I've been on as well to say, "Okay, how do we capture all this data and provide all this capability as part of our charge as an FFRDC for the nation, and really, the world?" I think that NASA and JPL's investment in things like the National Virtual Observatory, the Planetary Data System, the International Planetary Data Alliance, all the various pieces, really has helped drive a new future. There's been an increasing focus in the US, particularly over the last 20 years, but even the last 10 years. There have been directives out of OSTP and other initiatives saying, "We need to provide free and open access to data, and it needs to be publicly accessible." We carry that as a requirement for all missions, that data has to be released and made available for worldwide access. And I think the fact that you don't have to be part of the inner circle to be able to use that data and so forth is really opening things up and allowing academia, nonprofits, even for-profits to be able to use the data in different ways. You see NASA data in a wide variety of different applications now.
ZIERLER: You alluded earlier to the value of going to a highly distributed system as you're developing informatics at JPL. First of all, what are the technological challenges, and then what's the end point? How distributed should these systems be? Is the ultimate goal to get to something that resembles blockchain? What does that look like?
CRICHTON: As I mentioned, earlier in my career, and certainly as part of my master's degree, distributed systems is one of my focus areas. It brings a lot of really interesting and unique challenges in terms of how to implement and govern such systems. Part of what led to the work we're doing with the National Cancer Institute is that they wanted to be able to share a bunch of data across their cancer centers in a secure way. We had implemented a distributed-system concept for them that demonstrated that. The flip side is that it also exposed some of the real challenges between centralization and full distribution. As a computer scientist, I love the technology challenges. From a governing standpoint, federated systems are really tough to govern. It's easier to lay out rules for centralization and so forth.
What we found in distributed systems is that sometimes the weakest link can take down the system. We had groups that didn't keep servers running, so you couldn't access their data, things like that, so we had to look at caching systems, replication, ways we could begin to look at uptime issues, all those things. I think part of the challenge that we have going forward is defining how much distribution and federation we want, and how much centralization we want, and that tension is always there. It puts responsibility on those who want to have the data to make sure that follow through and satisfy their end of the job. I think as we look at this question going forward, we need to have a finite number of groups that are really focused on the responsibility they have to actually curate, store, and open up access to that data well.
ZIERLER: Was there a period when you worked with George and Ashish in a really intensive way? Or has it been sort of constant throughout the years once that collaboration started?
CRICHTON: In the last about 10 years, we've really worked closely together more and more. And we actually did a summer school early on in our relationship, really to open up and start to educate around data science because data science was increasing rapidly in popularity. I think back in 2012, 2013, it was declared to be the sexiest job in the world by someone. [Laugh] We ran a Caltech-JPL summer school on data science, and we did a lot of work to put that together, get that out, and really coordinate between our two centers as a capability. That was a lot of fun and a pretty intentional time for us. Beyond that, it's been working together to write proposals, working on research projects, and really pursuing ways in which to get funded, work out new ideas, and so forth. We've seen a number or projects flow through during our relationship.
Sky Surveys and Data Density
ZIERLER: As the technology in astronomy improves, in the way that the Sloan Sky Survey supplanted some of the technology coming out of Palomar, how does that change things for you on your end?
CRICHTON: On our end, we used to be very, very challenged with how to begin to even move data. It's a race condition between the amount of data, the amount of communication bandwidth capabilities we've got, and the computational methods we have in place. As a computer scientist, I often think of myself as an architect. It's looking at how we take all those variables and make the right decisions about how to set up this distributed systems environment. And it's changed over time, figuring out whether or not we can move that data over the network, whether we have to reduce the data, how much we can capture, how much we can process, all those kinds of things. Working with Palomar and others, it's really being able to capture that data and then make sure that we can process it and run it through some of our machine-learning algorithms.
ZIERLER: When you got involved with cancer research, to go back to this very interesting question about how astronomy was first-in on big data, talking with people like George and others, were you part of those broader conversations in realizing that what big data was doing for astronomy, it could do for all of science?
CRICHTON: I was. And in fact, George and I were both working in parallel. We were having this very interesting journey where both of us were seeing this, and then we began to join forces. George sort of coined it, he called it informatics-X, where X is fill-in-the-blank. We had bioinformatics. But I would go to AGU, and they began to call the sessions I was going to Earth and Space Science Informatics. I started seeing that word over and over again, and then data science came up, same kinds of ideas and so forth. I think what we had started to realize is, the software work I was doing, we were starting to see the applicability of that to all these different science areas, and we started hearing in this context the same kinds of concerns around the limitations in science in many different areas. I would go to a cancer research meeting, and they'd be talking about the challenges they had with all the data they were getting in cancer biomarkers. I'd go to a planetary science, meeting, it was the same thing. Astronomy meetings, we heard the same kinds of concerns. "We have concerns about where we're going to store that data, how we're going to access it, how we're going to analyze it. Whether or not it's well enough labeled to do machine learning." Very much, the exact kinds of conversations were occurring over, and over, and over again.
ZIERLER: As these developments were happening, and the realization that the value was absolutely there, what role did you see for industry, for the Googles and Amazons of the world?
CRICHTON: I would go back 10 years, and I would say the decisions we made–even 20 years ago–and today have changed greatly. Some of the software capabilities that we developed–as I mentioned, we developed this data-science software framework we called Apache OODT, which was runner-up for NASA software of the year, we would not build that today. We wouldn't have to build that today. We were pioneering things that just did not exist. What we've seen with Google, Amazon, and others is, they've actually adopted a lot of our practices in these organizations, then they turned around and delivered them as services we can use. What's interesting today about Amazon, and Microsoft is doing the same thing, is Amazon is starting to deploy things like ground station as a service. We've got a small satellite, we've got CubeSats that are orbing Earth. We can downlink those to Amazon, they can move our data into the cloud, and then we can begin to process that data, so it bypasses all this infrastructure that we've had for ground stations that we can begin to just acquire from cloud computing. I think what we're seeing is that the infrastructure in a lot of cases is being picked up more and more by the cloud computing environments, which means that our job is focused more and more around supporting the analysis and use of the data.
ZIERLER: We're talking about a process that's very much ongoing. In the way that astronomy was first-in, now biology is fully onboard, as you survey the fundamental sciences, are there still low-hanging fruit? Are there still areas of scientific inquiry that have not fully bought into what machine learning and AI can do? Or is it saturated at this point?
CRICHTON: I think there are a lot of pockets. I think the challenge is that we tend to do something the same way until we hit a fundamental block, it becomes a paradigm in which we can no longer operate. What happened when I was working in planetary science was, we hit a limitation of the approach in terms of how we were managing and working with the data that allowed me to come in and say, "Let's make this shift." What happened in cancer biomarkers is the same thing. I find that individual researchers and others who are doing science analysis, their toolsets and approaches are beginning to no longer scale to support the needs they have to do their analysis. I think that's the next low-hanging fruit. We did the large observing systems, we've done a lot of those capabilities. Now, the individual researchers are becoming more and more interested in, "What tools can I have that help with doing the data analysis?" Which means that we now have a need to train and educate our scientists so they have at least some level of knowledge around things like machine learning and data science.
ZIERLER: Moving our conversation closer to the present, something we've all dealt with, when the pandemic hit, what did that mean for you, not being around your colleagues in person? And in what ways, because so much of your work is computational, was it a pretty easy shift?
CRICHTON: I remember talking to our deputy director at the time, and they were trying to figure out, "What should JPL do? How do we continue to make progress as a laboratory and advance our projects?" Of course, we had a launch, we were going to go to Mars. I remember going to our deputy director and saying, "We're good. The way in which we build the software and deploy these systems, the way we're running them on the cloud, we already have a mode of working." A lot of the teams I work with tend to not be centralized at JPL or Caltech. We work a lot with groups at other NASA centers and academia. We were very much used to having these very distributed project and team meetings and the ability to work from different places. I traveled a lot, so I was used to working in different places.
We pivoted pretty easily to being a team working online. I think the thing I probably missed the most is, I didn't realize the value we had in terms of getting together. The fact that we weren't traveling and connecting, at least at certain times, I think did have an impact on our team, and its cohesion, and our ability to address questions. Sometimes when we're remote, it's harder to solve things, versus when we get together, we can solve a lot of things pretty quickly. We're at the place now of trying to find that right balance.
ZIERLER: Just in terms of your current work and looking to the future, for the last part of our talk, I'd like to ask a few retrospective questions, and then we'll end seeing where things are going. First, in the way that you got involved with the NIH and cancer research, it's hard to imagine something more impactful in terms of applied research and human betterment. Do you see opportunities for collaborations in the healthcare space beyond NIH? Or is there more than enough with the National Cancer Institute to keep you busy for some time to come?
CRICHTON: I think what we're seeing in the overall space itself is a need to inject these technology advancements in the way in which we do the healthcare. Data, AI, improved access to information and computation fundamentally is going to shift healthcare in the future, I think. We're already seeing examples. I had one of my team members who was at JPL, a Caltech post-doc, was in the area of imaging, and left JPL because he had a background in computational pathology. He went to Sloan Kettering Cancer Center, ended up starting an AI company called Paige AI, which has been very successful. The idea is being able to use AI algorithms to do analysis of pathology imaging with massive computation. In fact, some of those algorithms have already become FDA-approved.
We're seeing the results of these things not only happen within our relationship with the NIH, but it's going from NASA, to the NIH, and then out to drive change in the way healthcare is being delivered and performed. I think we still have a lot of work to do. Part of my experience working in the healthcare community is, in a lot of areas, they're still pretty far behind in terms of being able to leverage data-driven technologies. But I think that is going to change. In the next decade, I think there are huge opportunities for startups.
ZIERLER: What about the in the established biotech sector, the Genentechs of the world? What role do you see them playing in these developments?
CRICHTON: Areas like genetics–in fact, I've had some conversations with some of our pharmaceutical companies and some of the various other organizations, I know they've grown out of some of the NIH spaces I've worked in, so I know some of those folks, and there's a big push to be able to do more computational genetics, analysis, be able to bring that in for doing things like personalized medicine, to be able to bring in other data. I think imaging is the area we're going to see more and more advancement in as well. That's going to come into play, besides genetics. Obviously, George has got this spinoff with Virtualitics. We've had discussions about how to bring in immersive VR technology and other capabilities to really start to do more analysis of imaging data. That's what we've seen really the last 10 years in the cancer biomarker space as well. They've expanded from genetic markers to really now recognizing that imaging, and imaging markers themselves, is a whole other area of biomarkers we need to track.
AI for the Next Generation
ZIERLER: One aspect of your career we haven't touched on is mentorship, either with your team members or maybe even SURF students coming in from Caltech. What opportunities have you had to help train the next generation of computer scientists, and to flip that question around, for young adults, people in their 20s who grew up with far more powerful computers than you did, what might that upcoming generation have to teach you?
CRICHTON: One of the things I really have enjoyed in my career is bringing in students and being able to bring in folks who just have this excitement about being able to jump into these kinds of problems, to work at JPL, to be excited about the possibility of doing things. We routinely bring in students. Part of the relationship I have with George and Ashish is, we bring in SURF students through the Caltech side, and we've also done that on the JPL side as well. I think one of the benefits for them is, they've been able to write papers, get papers published, go to conferences, be part of that whole research academic space that we participate in, and just really work with a leading-edge team on some really, really cutting-edge use cases and problems. I think that's been a very important thing for me. And part of what I've been trying to do at JPL is to really work to create a career path for people in my area, really seeing that in some ways, as these areas have grown to become important, making sure there's a pathway for people to build a career at JPL. That includes meeting with some of these folks to mentor them, to help them make career decisions, and to also make sure we can build these teams and promote these folks up through the chain to have more and more responsibility.
ZIERLER: As you've risen in seniority at the Lab, taken on more administrative responsibilities, how have you managed to stay close to the research, not to get inundated by meetings, reviews, and all the things that are not really at the core of what computer science is?
CRICHTON: I sometimes view myself as an anomaly at JPL because JPL generally puts you on one of two tracks. You're either on a technical or science track, or you're on a management track. In the beginning, I said, "I'm a program manager and a principal investigator/principal computer scientist." I used that on purpose, basically to keep myself in these various lanes and areas, so that my work with the NIH and as a principal computer scientist has been so that I could keep my technical skills sharpened, and I can be embedded and work with the strong technical and science community itself, not just be a program manager. At the same time, I've got leadership I can apply, so I'm working to try and push forward as this evangelist to where we need to go and lead as well as having enough of the technical know-how and keeping my finger on the pulse of what's happening to make sure I can make strong decisions and help advocate with the level of respect for my background.
ZIERLER: Finally, some questions to close out, looking to the future. In terms of that evangelism, and looking at your own career trajectory, are there positions in your future where you might be a stronger advocate for machine learning and data science that simply might not be possible there now? Or is the leadership and your own role, are you basically happy with this current state of affairs and where things are headed?
CRICHTON: JPL is currently in the process of raising and elevating data science, software, and computing to a higher level at the Laboratory. Our deputy director, Larry James, has really been a strong champion of that. The Laboratory is actually looking to hire rather than a chief information officer, a chief data and information officer, and the intention is not to hire them to run the classic IT, but to really look at the broad range of, "What does it mean, as an FFRDC, to think from a strategic standpoint of software, data, computing?" To position us to have the right capabilities we can integrate into our mission science, flight software, and institutional activities and make sure that we are continuing to have a road map forward that raises machine learning and helps the Lab shift to become more data-driven overall, that lets us use our data for decision-making in many areas, and so forth. I think over the next 10 years, it's a shift we need to embrace to become more like SpaceX or Blue Origins, which we talked about earlier, where that might've been part of the DNA from the beginning. We've got to build it.
ZIERLER: Last question. Looking at those next 10 years and the key areas in which you operate, for astronomy and planetary science, for climate change, and for healthcare, what are you most excited about in terms of making an impact?
CRICHTON: I think I'm excited that we're going to open up new mission and science opportunities that we haven't been able to do before. One of the things I really want to be able to see happen at JPL is a lot more computing power on the spacecraft. Imagine us having internet in space. Imagine what we could begin to do. Imagine being able to put more autonomy so that we're not so much part of the loop of what's happening, so we can begin to let missions do more. Imagine rovers that can do more autonomous driving. Imagine sensors that can be more event-driven because they see events occurring that return data. I think all of that observing system is going to shift. That means on the ground, there are going to be immense opportunities for us to leverage more and more data. We need machine learning to figure out how to build better models to work with this data. One of the things we need to do is, we need to operationalize the use of AI and machine learning into our whole way of operating as a laboratory so it's just fundamental in the fabric of what we do. That means models are shared, models are used, we can trust models, and they become part of how we actually do science and execute our missions.
ZIERLER: And this is a truism across the board, astronomy, planetary science, biology, sustainability. It doesn't matter what the end use is, these are the methodologies that can be applied universally is what you're saying?
CRICHTON: I think what we're beginning to realize is that we're going to produce new models that are part of the whole scientific-discovery process. Not only are we going to do hypothesis-driven research, but we're going to build models that represent those hypotheses and can actually be used for validating science results and helping us gain science insight.
ZIERLER: Dan, this has been an excellent conversation, super interesting and important as we develop this DDA project. I want to thank you so much for your time.
CRICHTON: My absolute pleasure.
[END]
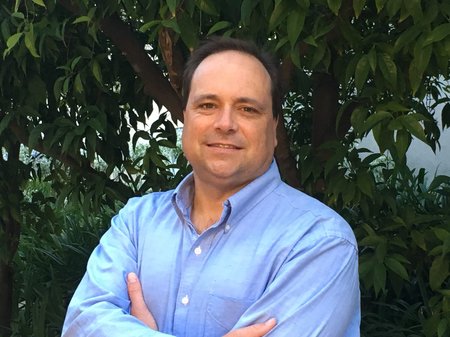
Interview Highlights
- AI From Biology to the National Defense
- ChatGPT in Historical Context
- Background in Distributed Systems
- Connecting with Data Driven Astronomy
- Computational Partnership From Lab to Campus
- Institutional Support for AI Research
- AI Inputs for Climate Change
- Sky Surveys and Data Density
- AI for the Next Generation