Gene Myers (BS '75), Computational Biologist and Leader in Genomics
One of the great trendlines in science over the past twenty five years is the increasing relevance, and even centrality, of computation. Indeed, computer science is not only advancing scientific capabilities within specific disciplines; it is serving as a hub that connects fields of research previously considered discrete, with outcomes that are proving fantastically exciting in both fundamental and translational research. Perhaps this transformation is no more dramatic than in the field of computational biology. Until the turn of the twenty-first century, most of biology was considered "small science," where experiments were done by relatively small research groups that managed datasets which rarely pushed the envelope for what computing capacity could offer. This has now all changed.
The career of Gene Myers can be understood as a microcosm of these changes, and the sequence of his educational and professional trajectory reads almost like a textbook narrative of how biology embraced computer science. After a highly mobile childhood, and despite the educational challenges this presented, Myers was accepted as an undergraduate at Caltech, where he took full advantage of the world-class education that the Institute offers. As he muses below, Caltech undergrads are thrown into a crucible, and for him, the less-intense research atmosphere offered by the University of Colorado provided just the right balance after Caltech. It was in Boulder that Myers became fully immersed in computer science, and it was at the University of Arizona where he began to think deeply about the potential applications of computer science in biology. It is not only the subsequent research achievements by Myers that makes him a pioneer in connecting these fields, it is the timing of his focus. In the 1980s, computational biology was in its infancy, if that, and there is no doubt that Caltech's quantitative approach to all of science was instrumental in the way Myers built the field's path to where it is today.
During his professorship, Myers made the decision to leave academia to join Celera, a biotechnology startup that, under Myers's leadership, made foundational contributions to mapping the human genome. As he explains below, this was not a project supported by the U.S. government; Celera was a competitor to behemoth agencies like the National Institutes of Health and the Department of Energy! Myers describes the massiveness in computational power that was required to map the human genome, and the satisfaction he felt in demonstrating the obvious value that basic science in genomics could offer in advancing human health. After an appointment at UC Berkeley and his desire in taking on a completely new research area, Myers became interested in microscopy, which led to his affiliation with Janelia Farms, a new intramural institute outside of Washington DC which supports cutting-edge research across the fields of biomedicine. In his most recent appointment, Myers accepted an offer to become Director of the Max Planck Institute of Molecular Cell Biology and Genetics in Dresden, an experience he enjoyed as much for its cultural aspects as for the unique and efficient way that science is funded in Germany.
In reflecting both on his career and his current interests, when Myers decided to go emeritus, he was motivated to be responsive to the characteristic that explains so much of his career: the desire to work at the frontier of knowledge, and to embrace knowing little about a particular research topic and to lean into being a novice as a secret weapon for success. In retirement, Myers muses that he can be a postdoc all over again: unencumbered by funding limitations or administrative burdens, and free to take on the next great challenge. This is undoubtedly the Caltech way, and it is with both a sense of excitement and an expert's appreciation for how much more there is to learn, that Myers looks to chart his future.
Interview Transcript
DAVID ZIERLER: This is David Zierler, Director of the Caltech Heritage Project. It is Monday, July 24th, 2023. I am delighted to be here with Dr. Eugene W. Myers. Gene, it is great to be with you. Thank you so much for joining me today.
EUGENE MYERS: Pleasure to be here.
ZIERLER: To start, would you please tell me—I know this is going to be a little complicated—but your current or most recent title and institutional affiliation?
MYERS: Most recently, my last full-time job was as a Director of the Max Planck Society, specifically at the Max Planck Institute of Molecular Cellular Biology and Genetics in Dresden, Germany. I stepped down as a Director, so I'm now Director Emeritus, as of April 2022. My current affiliations are: I'm an Adjunct Professor at the Okinawa Institute for Science and Technology in Okinawa, Japan; I'm an honorary faculty member at the Wellcome Trust Sanger Institute in Cambridge, England; and, I remain an Emeritus Director of the MPI, in Dresden.
ZIERLER: As Emeritus, in the German system, is it similar in the United States, where if you want, you can retain an office, a research agenda? Do you maintain an active research life at all in Germany?
MYERS: I do remain active there, and in the other places I mentioned. It's not the same in the sense that I actually—I believe that I get to keep the title for as long as I am alive, of Emeritus, but in terms of actually having budget, office, et cetera, that's all at the whim of my colleagues, as to whether they do that privately, at the Institute, and also, the Society itself. There's a central headquarters, and then there's 80 institutes that constitute the Max Planck network, spread around the various Bundesland, or states, of the German country. Basically, the MPG has a policy that I'm allowed three two-year renewable terms in terms of them financing me, and then it's over. So it's a little bit more definitive than in the U.S. Does that make sense? But if my colleagues—it's very interesting—the Institutes are in some sense fully independent. They report to the headquarters in Munich at the central site, and there are certain bylaws and rules that have to be followed. But how each Institute is constituted, and how it spends the money that is allocated to it, is entirely at the discretion of the board of directors of that Institute. Our Institute—I'm not going to bore you with the details of our structure, but our Institute, for example, has a completely different kind of internal structure and utilization of funding than many of the other institutes in Germany. Our Institute was actually a very international one. The directors—there was a British director, an Italian director, a Finnish director, a couple of German directors, and then me, the Yank, was there, so it was quite an international group, and we operated by consensus. But we had full discretion. In fact, it's the only job I've ever had where I took out insurance, because it's actually the case that the buck stops with the directors. It's not like being at a university, where the university is responsible legally and liably. No. We are actually the operative body, so basically it's up to the board of directors as to whether they continue to give me an office. It's generally very convivial there, and I imagine that I'll have an office there as long as I want it.
Going Emeritus and New Research Opportunities
ZIERLER: Where you are able to remain active, what kind of research are you involved in, and more broadly, what's interesting to you in the field?
MYERS: I decided to step down not because I really wanted to quit in any sense, but I wanted to go back to being an individual investigator and basically solving problems on my own. I'm not really a theoretician. I think because of my Caltech experience, I've got a lot of engineer in me. But I do do what many would consider to be theoretical computer science, which is where I basically develop algorithms or methods for solving complex computational problems. We think about the efficiency of those, and there's a whole theory of what makes an efficient algorithm. So, I've been in that area, but I have a penchant to want to make things, I think also because of Caltech, and the engineering. What I love about computer science is it's basically applied mathematics. It's applied mathematics, but then basically you can take your application, you can type away at the machine for a while, and then you have a program that actually does something. To me, that has always been beautiful, because you're actually making something in some sense. Yes, it's a program, but it's a program that does something interesting.
I've spent a lot of my career—I've had big groups, and then I've had small groups. In fact, I've struggled with that my whole career, because as my star rose, there was more money, more people, I got bigger and bigger and bigger. But actually what I noticed is that I got unhappier and unhappier, because I spent more and more of my time directing what other people were doing, and less and less of my time doing what I call having a good think, you know? [laughs] Because I like to sit and think and solve the problems and see the patterns. The whole reason for getting into the field was because of those eureka moments, where you see something. Those are very addictive, actually. They're very addictive. It's like you could say I'm an addict, [laughs] for an insight. I'm an addict for insights. So I love sitting there thinking about, deeply, the essence of things, and seeing their structure, and then having "Ahas" about things. That happens at all levels. It's not always running down the street naked [laughs]—"Eureka!" Although sometimes it's very powerful; sometimes it's just like lots of little connections are being made. That intellectual activity, to me that's the essence of being a computer scientist, slash engineer, slash applied engineer, slash technologist, I guess. I tell a lot of people that I'm a technologist, basically.
Like I said, when my groups got big and I had a lot of people, I tended to get frustrated, because yeah, I needed the people in order to execute the vision, sometimes—you just needed the manpower—but at the same time, I became more and more divorced from the thing that I loved, because my postdocs and my PhDs and my staff scientists, they were all doing that stuff. It's great, and it's fun to watch, and as I've gotten older, I love watching people develop, and I love watching the young people become facile in these things, and see their own careers go. I find that very satisfying, actually, also, to feel like I helped launch people. But at the same time, it's not the core of my love. I had several times where I got very big, and then I said, "To heck with it," and I basically just gradually shut down everything, [laughs] so I was back down to me, and then got big again—at Celera—and then I got small. Again, I was big, at the Max Planck. I was running the Institute for a while. So my concept of retirement is not that I'm not thinking and actively developing things—I am—but I'm not involved in raising money, I'm not involved in managing budgets, and I'm not involved in directing and managing and being responsible for other people. [laughs]
ZIERLER: So you just get to have fun, now.
MYERS: That's retirement! I'm like a postdoc again. I'm doing my own work, I'm writing my own programs, and I'm having a blast. What I'm interested in now, today, is I really want to go back—during those periods when I had big groups, there were a lot of insights that I had that I never got to act on, and there are a few problems in areas where I would like to finish and do a capstone piece. I'm thinking, I'm 70, I maybe have 20 more years if I'm lucky. Maybe only ten, you never know. But hopefully I have enough time to—and I thought, now is the time, maybe, to start, and to really do those capstone things, to finish some of the results. The thing that I'm really still in love with is—and it has dominated my entire career—is this problem of sequencing genomes, and I'm still very involved in that. There's now numerous initiatives to sequence basically all of the extent species on the planet, and that's because now we can do it almost perfectly. Back in 2000 when we announced the Human Genome Project, we sequenced the human genome, we had most of the information, but we really only had about 90% of it. Most of the useful stuff, we had most of the genes, everything, but we were missing bits of it. In terms of actually producing a beautiful—technologically producing an end-to-end sequence of the human genome, we had not done that. But we're able to do that now. Just—just barely. We can now produce basically out of the box almost perfect genomes.
There are still a number of beautiful algorithmic and computational problems on strings involved, and producing those assemblies and analyzing them and comparing them, and issues of also scale, of having codes that are efficient enough. Many of the codes that we use, they won't scale to what's coming. What are we going to do when we have 100,000 species of genomes sequenced and we want to compare them all? We don't have the software infrastructure in place to do that. These are kind of the things that I'm interested in now. In fact, those are the associations that I kept. I kept my association with the Sanger Center. It's one of the leading places in terms of doing this, in terms of pursuing this idea of sequencing everything that's out there.
Tracing the Computational Approach to Biology
ZIERLER: An overall question that will frame so much of our conversation, in all the ways that you've identified yourself—a mathematician, a computer scientist, a technologist—when and how did you decide to apply those skills to the world of biology, to genomics? How did that happen?
MYERS: That was a gradual walking. It wasn't an all-at-once kind of thing. There were pivotal moments. Towards the end of my grad school career—my PhD advisor was Andrzej Ehrenfeucht, a very eclectic, brilliant, Polish theoretician, at the University of Colorado. He was working with Gary Stormo, a PhD student at the time, on recognizing core promotors with a perceptron. They' built a perceptron—which is the first neural net; it's a very simple neural net—to recognize promoter sites in DNA sequences. This was at the end of the seventies. There was enough DNA sequence available—sequenced—there wasn't a lot, but there was enough that Gary was interested in, well, what are the molecules recognizing on the DNA to start the transcription of a gene, and so what was the core promoter. He was trying to build a neural net that would—well, he did build a neural net that recognized these promoters. He was doing that with Andrzej, because Andrzej was the guy in the Computer Science Department that was doing artificial intelligence and machine learning, such as it was at the time. Although I was doing discrete algorithmic theory. I was working on graph theory. But because he was working with Gary, and it was an interesting source of problems—how do you compare strings? We didn't even know how to approximately compare strings. There were problems, in what's the secondary structure of a tRNA, and there were some very interesting problems on strings. Just as a computer scientist looking at it over there, it's like, "Gee, those biologists have a lot of four-letter strings, DNA strings, or twenty-letter protein strings. I think that's an interesting variation of the problems that we think about."
We had, actually, a group seminar. We met once a week, and we all—it was David Haussler, myself, Manfred Varmuth, Gary Stormo, and a few others—forgive my failing memory. Haussler, Gary, and I all became quite prominent in the field of computational biology. We were some of the earliest people in, as a result of that seminar, basically. It wasn't really a seminar; it was basically a working group. We would get together, and we would talk about these problems, and people would present what they had been doing. That was the first thing [step towards becoming a computational biologist]. I had had this background. I then went to my first job at the University of Arizona in 1981. I was an assistant professor. I went from Colorado. I promptly forgot all about that [Ehrenfeucht's working group] and was focusing on compilers, and programming languages, and then concrete problems. But then about three years later, in 1984, computational biology was getting bigger, and biologists were getting interested. A quite well-known molecular biologist by the name of David Mount, at the University of Arizona, who is known for discovering the SOS response mechanism, basically wanted to have a computational biology center at Arizona. So he went to our department head and said, "Hey, I want to do this thing. Do you have anybody that could contribute to the NIH grant I'm going to write to have this center in computational biology?" He had written a popular book on basic things in computational biology.
Our department head, David Hansen, pointed at me and said, "Well, he thinks about strings"—because I had just done a piece on comparing computer files, so actually the diff algorithm for comparing two computer files, I had actually come up with a new method for that. Of course, that's the analogous problem to comparing strings. Basically, it's just instead of lines of code, it's the DNA alphabet. So he said, "Well, Gene's doing work that is probably relevant." So David approached me. I remember writing—I was an assistant professor—I remember writing a couple of sections for the grant, research sections, but I was very clear with David, I said, "Look, David, I'm an assistant professor. I'm worried about tenure. I don't want to be involved in the operation and administration of these things. I don't have the bandwidth for that, but I'm happy to work on these problems that are going to help me produce publications." [laughs] Sorry, is the story too long?
ZIERLER: Not at all. No.
MYERS: Because I do think it's a relevant story, actually. It was a very relevant event. What happened is the grant got to the point where it was site visited, so a site visiting team came out. It was quite an illustrious site visit. Temple Smith was on there. The Canadian—I've got to remember this name—well, there were quite a few luminaries—David Sankoff. What's interesting actually is the mathematicians were in first. The computer scientists were in second. The mathematicians had actually gotten involved in the late seventies. So, David, Michael Waterman, Temple Smith, these guys, had actually been working a little bit already on the comparison of strings. Sankoff was there. Sankoff is a very top of the line theoretician. He wrote a 1977 paper that was 10 years ahead of his time. It was a brilliant paper. He was there. So I was kind of like starstruck. I got up and gave my little pitch on a couple of things I was working on. I was working on a very complex way of doing string comparison incrementally. I had some ideas about pattern matching. I got up and I gave those talks.
In the meantime, the site visit just went horribly. David is not a computer scientist. He was basically ordering computers without disks [laughs]. And there were a lot of other problems, and I could see the whole thing going down. And it did go down. But what was interesting was that Sankoff actually wrote a minority report that said, "No to the center, but the kid's not bad." The kid—"You should fund the kid. He's doing interesting stuff." Of course he told this to Temple, and Temple is very socially gregarious, and Temple was putting together one of the first meetings, in Waterville Valley, where there was going to be a computational biology meeting. We didn't even call it that then, we didn't know what it was, but there was going to be a whole bunch of people that were interested in the fact that we were going to have a lot of DNA sequenced and we wanted to compare these things, and we wanted to be able to do a variety of analyses, and there were a lot of problems. Temple invited me. The consequence was that I got invited to the Waterville Valley meeting.
I went to that meeting, and that was transformative, in the sense that, to me, it was totally exciting, because it was like different cultures meeting. Because the scientific culture of molecular biology is completely different than the culture of mathematicians. Biologists work in a world where—molecular biologists—where they can't see or touch the things that they think about, and where intervening and trying to understand and getting any definitive information is incredibly difficult. Whereas as a mathematician, we live in kind of a crisp, neat world, where we have complete control. [laughs] So they are culturally quite different. Sometimes it's hard for me to wrap my head around molecular biologists'—at least at the time, it was. I think I've got the hang of it now. But that meeting was really incredibly exciting, and I decided that, yeah, I'm going to work on this. Because I had already been doing some stuff with Ehrenfeucht back at the University of Colorado, but like I said, that hadn't been a main thing. That was kind of a sideshow. But then after this meeting, I decided, no, I'm going to make that part of my portfolio. But it was part of my portfolio as a computer scientist. I still was doing computer science. And yeah, here's this cool stuff, it's kind of interesting, it's a really great source of problems, but I'm writing computer science papers. I'm writing codes.
I was actually trying to do theory. That's what led to BLAST, the search engine. BLAST was basically just a heuristic version of a theoretical result that I was producing, around 1990. But I still was thinking about it. It ended up being the search engine of choice because it was so fast, and it became—I think that paper is one of the top ten papers in Science, actually, in terms of citations. Because everybody uses that tool. I didn't even realize how popular that paper was, actually. [laughs] I was on it, and it wasn't until I think—that paper came out in 1990—it wasn't until like 1994 when there was the U.S. News Report about our department at Arizona. It was a very small department. There were just 13, 14 of us, really small. But we were suddenly number one in citations per faculty member. We beat everybody! [laughs] It was like, Berkeley, Cornell, everybody. It was like, Arizona, number 32 in the country, I think, or something like that. [laughs] What was funny was when we studied it, what we realized was it was that one paper was getting so many citations that it basically took the entire department average above the median for any other department. Then I realized, wow, that thing was really a home run.
Anyway, I continued in that vein, but I was trying very hard to—I was very interested in the assembly problem, involved in DNA sequencing, and so I continued to work on that, but I was having trouble having impact. I wasn't at a sequencing center. I couldn't get anybody to really use my stuff, because I wasn't there. Also what I was realizing was my stuff [software] wasn't quite tailored to what they needed in the lab, and so they tended to be using other people's stuff [software] that was more convenient to use, even though I had a lot of interesting ideas. It wasn't until the human genome thing came up, and I had been working on a method for doing whole-genome shotgun sequencing of the human genome. I maybe should tell you a little bit more about the ramp-up of that story. But right now, I'm still trying to answer the basic question of how did I learn more about molecular biology. The trend was basically, when I went to Celera, I had to solve a real problem. There was a real factory, real machines, and I had to deal with all of the caveats. That I think was a point at which I began to really be devoted to molecular biology, as opposed to computer science. I would say that I would have called myself a computer scientist up until that point, and then, I don't know, I became a technologist, I guess. But I was completely dedicated to producing things that actually solved the problem for the molecular biologists so they could do that.
It was also the point at which I was really exposed to a lot of molecular biology. Up until then, I had been kind of distant from it, but suddenly I was really beginning to understand what kinases, and phosphatases, and all the -ases, were, and beginning to actually have some understanding of cellular function, and became interested in cellular function and became interested in, how does the genome express itself in a way that creates a living cell. That trend just continued and continued, until eventually when I was at the Max Planck, for this last 10 years, I was embedded in a biological institute. So I went from being in a computer science department to being a director in a biological institute. In fact, I was in the biomedical section of the Max Planck Society, not the computer science section. So, really it wasn't, like I said, an all-in-one; it was just a gradual walking. I'm still not a molecular biologist. I call myself a technologist because what I do, and what I hope I do, is I produce the tools that allow molecular biologists to discover the things that they discover. Because having the best tools is really what the game is all about.
ZIERLER: Do you see your research career as a halfway point to where biology is going? In other words, is biology becoming, or is it already, so computational that basically everyone who wants to achieve success in biology really needs a strong computer science background?
MYERS: I don't think so much that they have to be computational. I think it's more they have to be physicists. I think physics is probably more important than computation.
ZIERLER: Hmm!
MYERS: Computation is going to be, I think, very important, because I think a great deal of the work is going to be simulation based. This is not the work that I have personally done myself, but watching it, understanding what a cell does is not just a question of biochemistry. It's a question of biochemistry and physics. In other words, these are biochemical reactions that are taking place in a physical context, and that physical context—in other words, the pressure, the density of the medium—processes are basically a result of condensation, of phase transitions —like the whole cell basically is poised at the point between being a very thick fluid and being a solid. And it's not by accident. By evolutionary design, that's how the cell very quickly forms complex and solid things and then dissolves them again. If you think about it, when a cell divides, the entire nuclear envelope has to disappear, dissolve, and then you have to reconstitute two nuclear envelopes. You have to recrystallize that thing. It's done by having things poised kind of right at their melting points, as it were. That's a physical concept.
There are just things like—the anterior and posterior axis, it's controlled by where the sperm enters the egg. Because the sperm enters the egg, it creates a wave, and it creates a wave in the cytoskeleton, which is basically a collection of actin fibers, which in aggregate basically act like a dense fluid, and they create ripples, and those ripples actually affect the stoichiometry of the Par-3, Par-5, biochemical relationships. I don't know if I'm doing a very good job of it, but the point of the matter is that I think that physics, and analytics, are going to be very important. Physicists have always had the most general training. I also think that's where Caltech has been very useful. They made us take a couple years of physics, even when we didn't like it. [laughs] I think that was very important. So, we've all got a little physicist in us. But I think it's going to be a combination of physics and computation. And it is the case that physicists do computation quite well -- but not as well as they often think they do.
ZIERLER: [laughs]
MYERS: Computer science does actually have something to instruct, and to contribute, in that regard. I think more that the modern molecular biologist is going to have much more analytic training. Molecular biology was always the place you went, when you didn't want to be quantitative. It didn't involve differential equations and complex things [mathematics]. It was mostly descriptive in the early days. It was mostly descriptive and mostly running kits. You had standardized kits that you could run that you knew had a given effect. I think those things are changing, and I think more and more, the sophistication is that—and there's still a tremendous amount of room for descriptive and observational work, but I think in terms of really mechanism, it's going to be that the training is going to have to involve a lot more analysis. In other words, you need to take calculus through multidimensions, and you need to take at least a year of physics, I think, to be truly, I think, a modern molecular biologist. That's what I think. It's not so much that you need to be a computer person, but that you need to be analytical and quantitative.
ZIERLER: A question we're all thinking about these days—you're uniquely positioned to comment on it, I think—where do you see the role today of machine learning and artificial intelligence on the development of bioinformatics? Where is this all headed? Where is the hype? Where are you really excited? Where are you concerned?
MYERS: The new thing with ChatGPT and the new level of functioning of AI technologies is very interesting. I've used deep nets in a number of very limited contexts, like resolution enhancement in microscopy. We did a piece on that. It worked very effectively. A deep neural net will learn any kind of continuous complex function, and that's very useful. [But] I think it's going to be very hard for it to penetrate medicine, because medicine demands an explanation, and the problem is you can't explain what these nets are doing. I think that the nets could potentially be—and AI in general—could potentially be very effective in predicting things, but I think the ultimate goal of molecular biology is to have some sense of intellectual understanding. In that sense, I don't see a really big strong role. Forgive me, David—the big advances—these completion systems like ChatGPT, the performance is really surprising. If you have a big enough corpus of data, you get something that looks like it's intelligent. But it's not intelligent like we're intelligent, okay? It's not. It's actually operating by completion. It basically knows everything that humanity has ever said and can complete it in a nice way. It looks like a very sophisticated individual. Which may be an interesting statement about human intelligence [laughs]; I don't know. But it's not a silicone consciousness, in the sense that we're an organic consciousness. I do think that will eventually happen. It hasn't happened yet. But in terms of helping us in terms of molecular biology, yeah, I can see lots of places where it will help, and enhance things, in terms of being able to predict things, operate machines, perform experiments, help with interpretation. But ultimately—at least I think—if we could get an AI to tell us exactly what would happen in a cell when you make a change, and the AI would get it perfectly but I have no idea what the AI's logic was for making that prediction, I don't consider that a win for science.
But it might be very useful for a pharmaceutical company. Like, "Yeah, this is a good candidate. Let's pursue this guy." It might, for example, by using AIs and being able to much better predict what the reaction is going to be to a subtle change. Most of what we're trying to do in terms of human medicine is we're trying to introduce perturbations, right? Or drugs or small molecules that are going to introduce perturbation in a set of molecular pathways of the cell. The reason that so many of them go down is because we don't know all the pathways, and so there are all these unintended reactions with the molecule we're introducing, and so you don't make it through third phase clinical trials. The animals die, or they get really sick, or they can't have children, or something goes drastically wrong, because we couldn't anticipate all of the effects. I do think that if we get to a point where we have a corpus of enough data—because these AIs, right now they are limited to the size of the corpus of the data. The question is, where is all the data going to come from, to learn what a cell does? That's the missing piece. But I wouldn't rule it out. I never rule anything out!
Longstanding Emphasis on Fundamental Research
ZIERLER: Gene, in surveying all of your contributions, to genomics, to molecular biology, if it's a meaningful distinction for you, where have you been motivated in basic science questions, just figuring out how the nature, how biology works, and where have you really been more applied, and thinking about clinical applications and therapies and drug discovery?
MYERS: I've actually never been interested in medicine, to be perfectly honest. I'm interested in science. In fact, I hold a view that we are spending too much money on medicine, and not enough on science.
ZIERLER: Hmm!
MYERS: Part of the reason I want to sequence everything is I think we're going to learn more about the genotype/phenotype relationship from studying all of the genotypes and phenotypes in nature than we are by studying human genomes and phenotypes to exhaustion. Because there isn't that much variation between people, but I can find two species of bats that are only five million years diverged but where the difference in the lifespan is 50. Not 10, 20 percent; 5,000 percent. We want to be able to improve our health by understanding our molecular function at some level, and it's not clear to me that trying to understand molecular function in human beings is the right way to go. We don't fit under microscopes. It's hard to experiment on us. That's usually not allowed. I think that operating and working and trying to understand how the cell works, in whatever kind of experimental system allows you to do that in a facile way, is what we should be investing our money in. I feel like a lot of biomedical, basic biomedical research, it's like, why are you doing that in mice, where it takes a year to breed a mouse? You can do the same thing in a fly and it's five days.
I've always been very interested in science. I'm very interested in the question of how the genome creates the creature, the shape. Honestly, I don't have the training, and/or the time, I think, at the point at which I became exceedingly interested in this question, to completely shift my career, so I continue to do what I'm good at, which is producing computational tools and analyses, and also building things that would help my science colleagues answer these questions. I started hanging out with biologists that were interested in development. I'm interested in evo-devo, and the question of evolution and development. How does a creature develop? How does that genetic program unfold to create us, and our five fingers? How exactly does that happen, and how is that programmed? I've spent a lot of time hanging out with scientists in that domain, and then doing things to help them. I've spent a lot of time thinking about sequence, but in the last 15 years, I've also spent a lot of time thinking about imagery, what happens in a microscope.
Because now that we have the genomes, we can light up anything of interest, and watch it, under a molecular microscope. And now that we have digital cameras, we can record that digitally. That all only happened around 2000. It has only been going on for the last 20 years. I've basically worked on a new area called bioimage informatics, which is really trying to interpret microscopy images, and I can give you a few examples of that. Producing digital information, and quantitating what's happening in these images, was exceedingly difficult. There was no tools or software for that. So I've spent a lot of the last 10 years developing that stuff, to help my evo-devo colleagues with their queries. So I'm a collaborator. I like team science. I think also that's kind of a Caltech thing, working in teams, interdisciplinary teams.
ZIERLER: A fun question, the one that brings us together, of course—in 2023, this year, you were named a Distinguished Alumnus. You received the Distinguished Alumni Award from Caltech. What was that like, and did that give you opportunity to reflect on what the Caltech education has meant for your career?
MYERS: Yeah, it did a bit. Actually I liked that. I've won other awards, but actually this one felt special, just because it [Caltech] was an important and pivotal experience for me, and I think to be recognized by the university that spawned me, in some sense, it felt very nice. I'm not sure how to say why, but it did. I was actually very happy to receive the award. It was quite—it gave me the warm fuzzies, and I'm not quite sure why.
ZIERLER: What has stayed with you from your Caltech days? How did Caltech teach you to think about the world, to think about science?
MYERS: I think there were several things. One was the idea of always approaching things with beginner's mind. We always were starting from scratch. I think it was part of the thing that the instruction was always at the level that—it was always slightly ahead of us, what we were being fed, so we were constantly struggling to understand things. I think one of the things it did is it made us really good at learning, and thinking about things deeply, because we're trying to understand what the hell the professor has been talking about, or what's in Feynman's book, for God's sakes [laughs]. What is he saying? Looks good, but how do I actually turn that into something? There was always this challenge to solve hard problems. Everything was hard, and you had to think hard. And there was a kind of can-do atmosphere. It was like, "We can do it. Always, we can do it. I can solve it. I'm going to be able to solve it. I'm going to be able to do it."
Then the thing also—it was a bit arrogant, but it was kind of this idea like, well, just screw it if somebody else has done anything. We're just going to start as if this has never been worked on before. I still tend to do that. Actually in some sense I'm a horrible scholar. I know a lot of what's happened in my field because I was actually there [laughs], exposed to it, but if you ask me to go back in time, or to go well outside my field, I don't read that much. And often times, when I get a new problem, I consciously ignore the literature. My method is to start working on a problem, develop some ideas, start to explore those ideas, and then once I've got those ideas in motion, then I go look to see what has been done. If I've rediscovered the wheel, then I just say, "Fine, I've rediscovered the wheel; I'm going to move on." If I haven't rediscovered the wheel, then I keep going. And, it's about a 50/50. I'd say about half the time, I've got a new angle on it, and about half the time I haven't. But what I find is if I really know something—and I don't want my brain to be filled with a lot of other people's thoughts. [laughs]
ZIERLER: Hmm!
MYERS: I want them to be filled with my thoughts, and whatever is being created inside my brain. I'm not saying that's the best thing in the world, but it's the most fun! [laughs]
ZIERLER: Let's go back and establish some family history. Let's start with your parents. Tell me about them.
MYERS: My mom is French. She was born of a French school teacher in France, and a father, Karl Fraenkel who was a polemic that hung out with Henry Miller and those guys in the French Quarter. She was half Jewish, and in World War 2, she was on one of the ships out of Marseilles to the U.S. and was left in the U.S. alone with her brother, without her mother, who worked for the government, the French government, and so she had the obligation to stay and be in the resistance. Although she did come later. My grandmother ended up teaching sailors French in Eastern Washington state, if you can believe that. I don't know why the sailors were up there, but that's where they were. My dad had just come back from the Merchant Marines. My dad was born in Idaho, grew up in Grangeville, a little town, in the Panhandle. Was in the Merchant Marine. Went into the Merchant Marine because they paid them more than sailors. But then he was really pissed when they passed the GI Bill, because he didn't get the GI Bill, so he had to pay for college.
They met in Eastern Washington, near Grangeville and that area. My mom was the lifeguard at the pool, and they met, one year, and they got married. They had me. My dad became a CPA, was the budget director for the state of Idaho the year I was born. I was born in Boise, Idaho, but I had my first birthday on a ship on the way to Pakistan, Karachi. My dad got the wanderlust from his time in the Merchant Marine—from a small boy in the Panhandle—and so he wanted to see the world. So, we basically spent my youth—he worked for Esso Eastern, which was a division of Exxon, and we went wherever they had energy or oil concerns. So we went from Karachi to Bombay—Mumbai, today—to Delhi, Indonesia—and then back to the States. We were in Connecticut for a couple years. Sometimes they'd bring him back to headquarters, which was in New York, so we lived in Connecticut. Then back to Japan, and then Hong Kong, and then I finished high school in Darien, Connecticut, before I went to Caltech.
ZIERLER: How did you manage to string together a decent education from all of those moves?
MYERS: I didn't. It wasn't a decent education. It didn't seem to matter. I think I'm very lucky. I think that genetically, I got a good roll of the dice. I guess—I'm named Eugene, which means "good genetics." Anyway. So I do think I was blessed with a good roll. Although my brother and sister were also highly intelligent, but they had different outcomes. But my mother was also—because my—my grandmother, my French grandmother, my mother's mother, was very academically oriented, and she really wanted my mom to become an intellectual, and get her doctorate. In fact, my mom was one of the first women admitted to the Sorbonne. But she didn't get to do that, because of World War 2. So my mom was interested in having a child that was an intellectual, so I think she did a lot to cultivate that in me, just in terms of making things available. Although, I don't know, what's interesting is that nobody noticed that there were a lot of subtle things, kind of going on, that I knew about, that people around me didn't seem to notice. Like I said, I think it was just—good luck, really, in the sense of—I was fascinated by numbers. I remember in Indonesia, once I understood what the numbers were, and how to count, I basically wrote all the numbers from one to a thousand. I don't think anybody knew that.
Then another thing was, for example, the teacher in first grade basically in passing mentioned that multiplication was repeated addition. I immediately got it. I went home, my dad's having a martini, and I tell him I know how to multiply. My dad starts giving me numbers to multiply, and I start giving him the answers. The thing I don't think he realized is that I was producing the answers by adding the numbers. Because I didn't have the table memorized. It's funny because when I told my dad about that later, as an adult, nothing—no memory of it. I can remember other things, like solving a two-variable problem in the fifth grade. I didn't know I was doing a two-variable problem, but I did it. And some other things that kind of indicated that something was going on. But you won't find any record of it, in anything about my education, and it didn't really show up until puberty. Then I found a Schaum's Outline on linear algebra. I'm 13 years old, and I look at this thing, and there's Cramer's rule, and how to solve a system of equations, and I was just totally sucked in. I don't think people knew that stuff was going on. It wasn't apparent until I got to Caltech, where of course I wasn't unusual at all, I was just part of the crowd, one of the crowd, that these things were going on. So, I basically am self-taught, all the way. I think that's also why Caltech resonated with me. I just had been interested in things and learning things. I think Caltech refined me. In fact, I really don't feel like my bucket of knowledge was filled until I went to grad school in Colorado, where they actually properly trained me in computer science and I learned [laughs] a concrete body of work, that I was doing anything other than kind of on-the-fly problem solving.
The Caltech Crucible
ZIERLER: When you arrived at Caltech, were you aware that it had just gone coed a year before? Did that register with you?
MYERS: Yeah, because there were like 10 girls, and that was it. It was a very unbalanced situation, actually. And, you know, it was a hard place, in some ways.
ZIERLER: It's a tough curriculum.
MYERS: It was a crucible. It was a crucible. But I'd do it again. Yeah, it wasn't all shits and giggles, no [laughs], at all. It was hard. But we bonded, we made relationships. We all got through in our own ways. And we learned a lot.
ZIERLER: Just to foreshadow, did you have any particular interest in biology as an undergrad at Caltech?
MYERS: No, none. Not at all. I never studied biology, actually. My biology comes from reading Gray's Anatomy when I was 10 years old. There's a section in there on histology, and I learned what the endoplasmic reticulum was, and the mitochondria, and I kind of had an idea of the constitutive elements of the cell. Then the other part of it comes from chemistry. I never took biochemistry, but I immediately got the idea from what we knew of chemistry. Since then, I've been self-taught a large corpus of information. But it's really the physics that I think has been the most valuable, those couple years of Feynman. I don't know, this is an interview, it should be positive, but I would say that—my experience with my peer group is that half of us went on to have very successful academic careers. About 40% had good careers as engineers and scientists but did not kind of advance beyond that. Then I would say 10% were basically dropouts, because they were fried, they were burnt out, it was too much. Because it was—like I said, it was a lot. I still think that a place like Caltech, there's a place for it, because I do think that it has produced some really terrific people. But it's like a crash course, and not everybody swims to the finish line. That, I think, is the downside of it.
ZIERLER: What about computer science at Caltech? Was that available to undergraduates? Were you following what Carver Mead was doing in electrical engineering, for example?
MYERS: I knew about Carver. He was doing circuit design, primarily. There was a Computer Science Department. It was a fledgling department. There was a guy there, Giorgio Ingargiola, who was doing compiler work. I took a few courses in computer science, but it was a bit spotty. I had a job working on a compiler, but I was trying to write it in assembly language, which made it hard ! I learned a few things, but it really wasn't until I went to Colorado, and they had really a proper [Master's] program, and a faculty that could teach kind of the breadth, that I really would say learned computer science. But I had some interesting exposure in terms of theory and computer languages, and I certainly learned how to program in that time [at Caltech].
ZIERLER: What was the trajectory, going from a mathematics background as an undergraduate to a computer science program for graduate school? What was your thinking there?
MYERS: I fell in love with computers. My first semester, we took a programming course, and they had us write PL/1 programs for sorting, and we had to punch the cards and do the whole thing. I just thought that was the coolest thing, for exactly the reason I told you earlier, which is that to me it was fascinating that I could think about something, type it, a sequence of instructions, and then have something that actually did something—sorted an array of numbers, or—and I found that I just was totally lit up by that. So I already was very interested in computers. I did a lot of electrical engineering, actually. I did a lot of digital design work. In fact, I had built a simulator for digital circuits, and it had the RC delays and everything. I was very proud; I would walk around with my two boxes of cards, and would run my program on my little synthetic digital circuits. In fact, I think I was the outstanding Honeywell engineer in my junior year for that particular piece of work. So, I was working on it. It was a discrete event simulation. I didn't know that at the time, but it was. And it was one of the earlier ones.
Like I said, I worked for Ingargiola, and kind of learned how to program. I took the theory course from him. Took a lot of electrical engineering, too, though. I was one unit shy of getting an electrical engineering degree, actually. But I got a degree in mathematics. Although I could have had a double degree but—so here's another thing. There was a one-unit public speaking course that senior engineers had to take, to graduate, and there was a very old professor teaching this. I had an advisor, a much younger guy. I remember I liked him a lot. I can't remember his name. Maybe if I go back and look, I can figure it out. But he was encouraging me to stay in engineering, electrical engineering, and get a degree. I was a junior, and he said, "Well, that guy won't be here next year. Don't worry about it. You're not going to have to take that course. Keep going." So I did.
So, I was basically one unit shy. So I did a lot of electrical engineering, actually. We had to design circuits at one time. I've forgotten it all, but I did, at least at the time, know. I had been doing also computer science, which was part of electrical engineering, was part of it at the time. I never met Carver, in person; I just knew about him as this larger-than-life entity there. The obvious truth was that I was embarrassed to speak in public, and I was afraid, and I didn't want to do it. That was obviously what was going on. But in my infinite wisdom, I thought that that was okay, because I didn't think that public speaking was going to have anything to do with what my career in research was going to be about. I thought that I was going to sit there and think great thoughts and write code and do stuff. I was completely wrong! It turns out that giving a speech is an incredibly important thing. In fact, as an academician, it's one of the things that can kind of make or break you, if you can get up and give a speech that interests people or not.
The surprising thing is that I think I'm not too bad at it, although I think a lot of that was the result of the years of teaching I did at Arizona. I spent 18 years of my career in the classroom, teaching. I think there's nothing like trying to teach something to kids that don't want to learn it, to help you get good [laughs] at explaining things. I think that whole thing of teaching, of trying to figure out how to reduce things to their essence, to really get down to the crystal, and putting that forward, I think really helped. But like I said, to me it's just so amusing and satirical that—ironic—that I didn't want to take this course, and that I didn't get the degree because I didn't take this course, when it turns out it's one of the most important things, about having an academic career. Because I did not understand what a research career was. I was still quite naïve at that point. I was also very young. I came to Caltech when I was 16, so I started pretty young.
ZIERLER: You skipped a grade or two?
MYERS: I skipped a grade.
ZIERLER: Being a college student in the early 1970s at Caltech, I'm curious, was it political at all? Were people talking about Vietnam, about the Civil Rights Movement, those kinds of things?
MYERS: No, we weren't. We weren't political. We didn't have the bandwidth to be [laughs] political. We were just trying to keep up with our classes. I mean, we all wanted to avoid the draft. We did talk about the draft. There was the draft. I got a draft number my last year. It was pretty low. I think it was like 32 or something. But that was the year they only went to 10. It was the last year. So we didn't really have to worry about it. But we were worried about it. That was the one thing we did talk about. But political, no. At least I certainly wasn't. Some of my classmates might have been. But that—no. I'm still not political.
Thesis Research in Computational Language Analysis
ZIERLER: When it was time to think about graduate school and you were focused on computer science, why Colorado? What was the draw?
MYERS: I could have gone to MIT, worked with Liskov, or Cornell, or I had some offers from some top-tier schools. But, I don't know, I'll just tell you the truth, David, which I just felt like I needed to kind of back off. I had had enough pressure. I had had four years of pressure, and it was enough, and I wanted to go to a place where the sun shone, the girls were cute, and there were lots of them, but at the same time where I would get a good education. An enclave of my peers, of my upperclassmen, had actually gone to Boulder. Steve Bankus — I talked with Bankus and he said, "Look, the program here is very good at Colorado. You'll learn computer science." In a way it was kind of the opposite order, because Colorado is not the top school in the country, but I went there basically to decompress a bit, and at the same time, not to quit. I was going to keep going, and I was in a good program where I was learning. Like I said, I thought it was a really good program. They really trained me. They really gave us a solid and connected and coherent corpus of training in computer science at that time, and all the dimensions of it, and I think that was very valuable, that master's level training. It was also nice to actually for the first time be a big fish in a little pond, again. Because I had been basically nobody at Caltech, one of the struggling minions trying to get through, to be one of the top students, basically, and that was nice. That was helpful.
ZIERLER: Going to Colorado, did you know already who your thesis advisor was going to be, or you developed that in real time?
MYERS: No, I went and worked with Leon Osterweil and Lloyd Fosdick, who gave me a research assistantship. I was working on program language analysis, as I knew a lot about programming languages. I worked with them. But that was another pivotal thing. The first pivotal thing was in my freshman year at Caltech, actually programming and realizing how cool it was, and like, "Yeah, this is what I want to do. I want to figure out how to write codes and do cool things." Making my simulator and things. My first semester there [Colorado], I took a course from Hal Gabow in algorithm design, which was the whole mathematics of how do you solve problems, how do you sort strings, how do you compare strings, how do you sort numbers, how do you—there's a whole corpus of methods for solving basic [computational] problems, and it was just fascinating to me. It's a huge field, because there's an unlimited number of problems. But looking at these solutions and seeing this cleverness at how you can save time and space in these computations—and it was basically mathematics, it was basically discrete mathematics, which was wonderful.
Basically it's induction. I would say that I'm a master of induction. It's not continuous mathematics; it's discrete mathematics. It's combinatorial mathematics. You can do it all on your fingers. You can do it all by counting. I loved that about it. And yet it was really deep. The structures were fascinating. I became completely enthralled with that, even though I had gone to [Colorado to] do work in programming languages. I started working on problems in graph theory and developed my own thesis topic. By the end, even though I worked and continued to work for Lloyd and Leon, I ended up with Andrzej Ehrenfeucht as my advisor because I was doing algorithms, computer science. That was another shift, was that one, I would say. Then the later one was the Waterville Valley meeting, and then Ehrenfeucht seminar. It's interesting how it wound up. These things happened, and they shaped me.
ZIERLER: This will sound like a long time ago, but in the mid 1970s, what did the computers look like that you worked with, as a graduate student?
MYERS: I think it was a 360 mainframe, an IBM 360. It was a big box. It was maybe three refrigerators worth of box. It wasn't super high. It had a card reader. I would go in with my box [of cards], and drop my box off on the desk, and the guys would take it, walk back in the machine room, and put the cards on the card reader, and let it rip. I remember the computer room, they had a big room with punch card machines, so we would all go in with our programs, our little charts, and sit there and punch cards. The terminal didn't come in until just like the middle of the seventies. In 1978, 1979, there started to be computers that—actually Caltech had a DEC-10. I remember that. That was fascinating. In fact, I still have the programming manuals for those machines, actually, somewhere stashed away in my library. They're falling apart, but I've still got them, especially the DEC-10 ones. That was the point—it was very interesting—I was at that age where, if I had had to work on punch cards, I would have never got as good as I got, in terms of writing software.
The real transformation was when we could be at a terminal, and we could type at the terminal. That was happening right about 1974 or so; we could talk to the DEC-10, and it was an interactive computer. Prior to that, it was take your punch cards, and hand them in, and then you get a printout later. After some amount of time, you'd get a printout back. It was very slow, because you had to debug. So the cycle—you make a mistake, and you don't know you made a mistake for an hour, whereas on the terminal, you just said, "go," and the mistake was obvious, and you could fix it, and then find the next mistake. Because you have to debug programs. Nobody gets it perfect. The debug cycle was suddenly fast, and suddenly you could really start to produce codes. You could get things to work. And, you could start to create big codes. That persisted, and so it was kind of like just when I went to grad school, that that really became the method of talking to the machine. Compared to today it was clunky, but we could still do it. I think that's where—and like I said, even though I was a theoretician and I was designing algorithms, every algorithm that I designed—in other words, every kind of applied math problem that I solved and figured out a theoretically efficient way to do it, I would write a code for it, and test the code, make sure the code worked, and if the code is successful, I would deploy it in some way. So I think that experience—because many of my predecessors in the field, basically they were either electrical engineers like Carver Mead, or they were mathematicians, like Ingargiola, and they didn't really know how to write code. They would write papers about how to solve problems but they never wrote code for any of them. So it was the beginning of that generation of people that could really be both theoretical and practical.
ZIERLER: Tell me about developing your dissertation research. What were the big questions you were after?
MYERS: Actually it wasn't a very big question at all; it was a very small question. It was a very small question [laughs].
ZIERLER: Maybe a focused question, is a better way to think about it.
MYERS: Yeah, I was working on graph connectivity. I wanted to see if I could do four-connectivity in a linear time. That's what it was about. So it was an extension of some work by Robert Tarjan. In fact, my thesis was not very good. But what had happened was in the meantime I had written several other papers. I wrote a paper about a procedural data flow analysis. I ended up solving this problem for the "for all" version of the problem. So I could prove that however you executed this program, [a particular] something would always happen. This turned out to be a very important paper, actually. It was the one actually that I used for my interview talk, I talked about that result. My thesis was a thesis of 110 pages on my attempting to show how to do this [4-connectivity] problem in linear time. In fact, I never achieved it, actually. I got very close, but the end of the day I couldn't solve it. But I wrote it up and they gave me a degree! In the meantime I had produced three or four other papers. I had produced a paper on a macro-processing language for FORTRAN. I had solved this data flow problem. I had another successful graph theory paper on how to generate all the spanning trees of a graph, a theoretical result. Today, I would never get a job with that portfolio, frankly. In a way I feel a little bit bad. The number of publications that people are expected to have today, I think it has just gotten a little bit crazy, honestly. But I had some good papers, some very good papers, and that's what got me the next job, got me to the next station.
I didn't get the top offers, either. I was getting offers from schools of the same level as Colorado—Arizona, Illinois, Rice, a few others. In fact, what was interesting was, in a way, I did the career incorrectly, because the career should build. You should be going to the highest grad school, after being at the highest university, and that's one strategy. Whereas because of Caltech, I had taken a notch down [to Colorado], some would say. I don't think it was, not in terms of the education at least, but I went to a place that didn't have the same ranking, which was number 30 or so in the country. I was getting offers from other places in the top 35.
ZIERLER: Were postdocs not common during those days?
MYERS: No, no. You didn't have to postdoc. That was the other great thing. I was there at the right time. There was tremendous demand for computer science. There were these huge classes. There were these thousand-student classes, and they didn't have instructors. So I did not postdoc. I went directly from my PhD to an assistant professor position.
Algorithms and Biology at the University of Arizona
ZIERLER: Do you think that served you well? Was that a tall ladder to climb for you?
MYERS: No, I don't know. It was the ladder I took. It's hard to say. Because sometimes people say, "What was it like living overseas?" And I say, "Well, I don't know, it's all I knew. [laughs] What was it like living in the States your whole life?" Anyway, it's the step that I took. I do think that it was a pretty big step up. Suddenly I had to learn how to write grants. I had teaching responsibilities. I had to conduct my own research. I had to have my own [research] plan. Caltech really helped me in that regard. I didn't have any trouble coming up with a plan. I think Caltech really inculcated creativity, at least in me, so I had lots of ideas. The harder thing was writing the grants and learning how to do things. I'll tell you a little bit about that in a moment. What happened basically is that I was interviewing, and I was doing pretty well, and I interviewed at Arizona. Like I said, I wasn't getting interviews from the top places, the top five, but then I'm pretty sure what happened is one of the people at one of the places I was at kind of realized that actually the quality of my work was very high.
Because people were making decisions about who they were going to interview based on their pedigree, and I did not have a good pedigree. I mean, Caltech was good, but they were really looking at the grad school, and I didn't have the pedigree. But then they talked to somebody at Cornell, and the next thing I know, I have an invitation to Cornell, which is top five. It was simply because through the grapevine, somebody had said, "The kid's not bad. He's actually better than his origin would suggest." So I went there. And you know what? I decided not to take an offer from them. Because what I realized was that I would be going back into a pressure cooker, that I would be expected to perform at a very high level at Cornell, and I would feel like I would need to perform at a very high level, and I would constantly be wondering if I was good enough. I was already wondering that [laughs], as it was.
I decided to go to Arizona. Because it was like a little department; there were like 10 people, 10 faculty members. Ralph Griswold was the chair at the time. We went to a Chinese restaurant on Fridays and had our faculty meeting over Chinese food. It was very convivial. What I recognized is that there, I would be important, and there, I would be supported, and there, I would not feel so pressured. That there, I would do probably better work than at Cornell, because I wasn't really secure enough in myself to take that step. So I went to Arizona, as an assistant professor, and I was supported. I got a lot of help. I could go talk to people and ask them how to write a grant, and "How do I do this?" and "How do I do that?" and it was a very supportive place. And I felt important. I was the only one who did algorithm design, so I was the algorithm guy. Psychologically it was very important to me, actually. Because I already had a lot of doubt. At one level, I still can't believe I got tenure. I was kind of like, "Really?" I think one of the hardest things as people is for us to be able to see ourselves. We don't see ourselves. We don't really know who we are.
Although I think in this one sense, I actually was—I had a little bit of wisdom. Unlike my one unit shy from the speaking course, I at least I think made the right decision about Arizona in the sense of knowing myself enough at that point to know that I wasn't going to do well at Cornell, but that I would do well at Arizona, or at least that I had a chance of doing well at Arizona. I was there for 18 years, and I moved through the ranks very quickly. Like I said, then I ended up doing some very important work, with the BLAST thing that became a top ten paper in the field of science in general. I've 260,000 lifetime citations. [But] I still only feel as good as my last result, most days. I think that's another thing that I learned at Caltech, actually, was humility. I learned that there was always somebody who was smarter than me. Because up until then, I had always been the smartest one, wherever I was. I could kind of walk around—"Yeah. Hey." But, I got to Caltech, and there was always somebody better. That's a bit ego deflating but it's also a good thing to know. [laughs]
ZIERLER: Do you see your early work in algorithm design as really providing the foundation for your entrée into biology? Would it have happened regardless, do you think?
MYERS: Going into biology? I don't know.
ZIERLER: Is there something so specific about algorithms and their importance to biology that it was inevitable, almost, that you would have gone in this direction, because of your expertise?
MYERS: No, no. There are lots of algorithmicists, and they don't do computational biology. So, no. I just took algorithm design and applied it to computational biology and became an expert in that domain, and in the problems in that domain. I would say the first 10 years when I was at Arizona, especially after the Waterville Valley meeting—Webb Miller and I did a lot of the seminal work on how to compare sequences. There were no algorithms, and we developed a lot of that body of algorithms. There were other people involved as well, but we developed many of the seminal methods in that time. But that's not a function of being at—it's not a natural that an algorithmicist would have gone there. I think it was more because of my experience living in diverse cultures as a child that made me so interested in the diversity of working at cross-disciplinary boundaries. It is difficult. Still, a lot of people talk about working across disciplines, but people are either naïve about the cultural differences, and the fact that they have to be attuned to the cultural differences in the sciences. We're not talking in sociological terms; we're talking about the culture of different scientific fields. Because they have cultures. They really do. So, there was that. Another common thing is that people don't appreciate each other in terms of their relative contributions and what they're doing.
ZIERLER: What was it that caused your initial interest? Was it a graduate student, a paper? What caused you to jump in?
MYERS: To computational biology?
ZIERLER: Yes.
MYERS: Like I said, I think there was the seminar with Ehrenfeucht when I was a grad student, and I saw that there were very interesting problems there, in computational biology. I like interesting problems. I thought, "Oh, that's interesting." But then when I went to Arizona, I was like, "Okay, I've got six years, I've got to get tenure. Okay, programming languages, here we go." But then I still kept thinking about sequences. Then there was that thing with Mount, where we ended up doing the NIH submission, and I ended up getting invited to one of the first computational biology meetings. I think that was the point at which I said, "I'm going to really work on this." If you want a kind of what was the point where I said, "I'm going to take what I know how to do and I'm going to really work in computational biology and be focused on it," it was after that meeting [Waterville Valley]. I had so much fun at that meeting. It was like, "That was great, man. I want to go to more meetings like that." [laughs]
ZIERLER: This is a wide open world for you.
MYERS: It's just fun, and it's exciting, and you could feel the excitement. What I really like is—I like to do my own work and have a good think, but in terms of externalities—and I'm very much an introvert; I spend a lot of time living in my head—but on the outside what I want is I want to be in an exciting environment. I don't care if I'm the star or not. At least at this age, I don't. But what I want to do is I want to be where it's happening [laughs], where it's exciting, where thing are going on, where science is advancing. That meeting felt like that, so that was the point where I was like turned on. It was like, "Yeah, this is happening. I'm going to be in this." That was 1985, Waterville Valley. It was a three-day meeting. That was the turning point, if you want to kind of write it up that way.
ZIERLER: How brand new did it feel as a computer scientist, as an algorithmicist, to be in that world? Were there others, or did you really feel like a pioneer?
MYERS: No, there were not a lot of others. No, there weren't. There were a few people. You're never alone. And like I said, the mathematicians, there were a couple of mathematicians that had waded in before me. At Waterville Valley, I met Mike Waterman, who was a mathematician. Sankoff was there, I think. But otherwise there were very few—I was the only kind of tenure-track, tenured computer science professor at that meeting. I got up and I gave a talk that nobody understood. [laughs] Because they couldn't understand it. But I tried to make it comprehensible. I gave the talk that Temple invited me to give. The biologists got up and gave their talks, and I didn't understand 90% of what they were saying. But at the same time, there was excitement. There was like, "Yeah! This is new!" It was fun. Like I said, we didn't even speak the same language, we didn't know how to talk to each other, but we knew what it was going to be worth if we did.
ZIERLER: That begs the question of how the collaboration started. Did you identify problems in molecular biology for which a computational approach would be useful? Did people come to you and say, "We need help figuring this out"? How did that work in the early years?
MYERS: Mostly, like I said, there was this gradual transition from being a computer scientist to being a technologist, fully immersed in molecular biology. This was a 50-year career we're talking about. At the beginning it was basically there was a collection of problems. I knew what the problems were. I knew from the literature. I was extracting these, and improving on a lot of them, actually. I would say a lot of the initial work was taking some of the first wave work that the mathematicians had done, and doing my computer scientist thing, and actually making it better, more efficient, and faster—so optimization, I would say. But I was also getting some new things, and then I was talking to Mount, and started having conversations with people. I organized my own meeting in 1988, and that led to some new problems, and we were talking to each other.
But a lot of it was taking problems that were [already] extracted and then working on them to make them better and improve them. I would say that's the original work. In fact, I remember kind of a tough love moment. I was with Mike Waterman in Lyon. I forget what the meeting was, but we had both given a talk in Lyon. I was deathly ill, but we were on a taxi on the way back to the airport. This was around 1990. I had been in, and I had done some nice work. I said to Mike, "So, Mike, what else is cool to work on? What are the next problems?" I'm not sure why Mike did it—we're actually friends; we like each other—but he's an older guy—maybe it was because I was deathly ill in the back of the car and being a pain in the ass, but he said, "Why the hell should I tell you that? That's the most important thing!" [laughs]
ZIERLER: That's great.
MYERS: I felt like slapped in the face, you know? It's like, "My god, he's pissed at me." But I remember what he said! That was real wisdom, because it is about knowing what to work on. Actually, that represented kind of another turning point. So you can see it's not—I know as a writer, it's probably nice to find a—I mean, there were small moments. I would say that in terms of a portrait, it's not like there was one moment and it went 90 degrees. There were little things, linkages, that led to things. Because that comment, I took that to heart. I was like, "You know what? He's right." I recognized and knew that he was right. Anybody with a PhD can read, and [with] sufficient IQ points, given a textbook problem, in enough time can usually solve it. But the trick, as a researcher, is to know what the problem is, and whether or not it's even solvable. That's the other thing. Because you get a textbook problem; you know it has a solution. But if you're out there doing research, does it have a solution or not? That was the point at which I began to realize, I really have to pay attention, and I have to start looking and seeing, what are the problems, and start talking to people. That actually changed the trajectory. It didn't happen overnight, but I realized, yeah, knowing what to work on, and when to work on it. In fact, I think that later in my career, that was one of the biggest gifts I had, to give to my students, really, was, "Here's fertile soil. Here's the place where if you work, you're going to get something." But knowing where that is is the art, of the highest levels of research, I think.
Celera and the Origins of Genome Mapping
ZIERLER: Knowing those research problems, is that really most important in your decision to leave the faculty at Arizona, to start at Celera Genomics?
MYERS: Oh, no, that was [laughs]—that was the opportunity of a lifetime. We haven't really talked about the Celera story. Uch, I'm tired already. Can we do this in two parts?
ZIERLER: Sure! We can.
MYERS: Because I feel like there's still a lot of stuff that hasn't been done [talked about]. But let's do Celera, because I think that's probably an important story. Like I had said, I had been working on this problem of, there's this problem called the assembly problem, which is, given all of the DNA sequence data that's produced, basically tell me what the sequence is. It's a big kind of word jigsaw puzzle. I had been working on that for quite some time. It was a very interesting problem, fascinating combinatorial structure. I'm still fascinated by this problem, actually, believe it or not. And believe it or not, the problem is still pertinent, which I find amazing because even though the technology has advanced incredibly, it's still in some sense the core computational problem for the purposes of sequencing a genome, a genome you've never seen before. I had been working on this problem, and around 1985 or so—and there was increasing interest and momentum and organization towards sequencing the human genome, a three-billion-base-pair human genome.
This was a big deal. It was very controversial in the 1980s. Everybody was arguing about it. But in the 1990s they were beginning to go. Although some people were saying, "Should we really spend three billion [dollars] on this thing?" About 1995, I started to have some insights about the idea that—the plan had been to—the belief was that you couldn't actually sequence anything bigger than like 100,000 or 150,000 letters, that you couldn't run the sequencing machines and reconstruct something any bigger than that. So three billion was just out of the window. So what people were doing was they were cloning 150 kb pieces of DNA. They were called BAC clones. They could take 150 thousand base pairs of the human genome, they could put it in this cassette, and then they could propagate it and amplify it, and it would be a pure aliquot of that 150 kb, and then they would sequence it. The idea was that they were going to break the genome down into 50,000 of these 150 kb pieces, they would sequence them, and then they would have a big top-level puzzle, but they would [first] figure out where all these pieces came from. It was called physical mapping. The public sector was going ahead and doing that.
I instead proposed and started to think about the idea of whether or not, instead of doing all that complicated physical mapping, whether or not it would be possible to just run the sequencers on the human genome, the whole human genome, and then put the 50 million pieces together. In other words, instead of doing 50,000 1,000-piece puzzles, can I do a [single] 50-million-piece puzzle? Kind of cool, as a computer scientist. I started thinking about it, theoretically, in 1995, and I started having some insights. I won't describe them all, but I had insights that were saying, "Look, it's not as crazy as it sounds." Because people were saying, "That's crazy. That won't work." But I started thinking about it. Then I realized that if you collected the data in pairs, where you kind of knew how far apart the pairs were, then it really was looking possible. Jim Weber and I wrote a paper in 1996—because Jim Weber had actually approached me, because he also had the same idea, and said, "Can we do this?" He was thinking the same thing. In fact, it was interesting the way things worked out, because a friend of mine called me and said, "You're going to get this call from this guy Jim Weber, and he's going to want to talk to you about potentially shotgunning the whole human genome. You should not poopoo him. He's a serious guy, and he's a really good guy. You should talk to him and at least think about it."
He did call. I got off the phone, and I started to really think about it. Together, we put together a paper that proposed how we would do this. I did some preliminary simulation work that showed, actually, that if you knew all the parts of the genome that were repetitive, that were difficult, that you could then get a solution. There was a contingency—if you could tell whether something was repetitive or not. This was fun, because we submitted a paper to Science. We got rejected. We submitted it to Nature. We got rejected. We submitted it to Cell. We got rejected. We just kept getting rejected. Everybody was saying, "No." No, no, no, no. To our little paper. It was a really serious paper! Finally, what was it, I think it was Genome Research said, "We'll publish it, subject to the condition that it is followed by a critique by Phil Green"—who was kind of the notable computational guy in the public sector. He was associated with the labs. He was kind of my counterpart in the large public sequencing centers. It's the only paper I've ever had where the paper was followed immediately by another one that said, "It can't be done." Phil gave an excellent argument as to why his computer program would not work, because he didn't understand what I was saying. [laughs] It was really interesting. I thought, "For a smart guy, this is pretty stupid." He's stuck in his own view. He's not getting what I'm saying. Because he's continuing to think about the problem locally, and I had been thinking about the problem in terms of the global architecture.
That paper finally came out in 1997, and the other thing that was fascinating about it, to me, was that when I asked people why they didn't think it would work, I wasn't getting principled answers; I would get emotional answers. To me, that said, "I've got something." [laughs] I've really got something here, because I'm not getting rational answers from these people. I'm just getting, "I don't want to believe it's so" kinds of answers. I thought that was very exciting. And it was exciting, actually. I went, "Wow, okay. [laughs] Maybe I'm going to be on the wrong side of this thing, but this is fun." Then what happened is that nobody would do this. Everybody poopooed it. They had a big meeting in Bermuda Island, of all kind of the public guys. I knew all these guys, and they were friends. They basically blackballed me from the meeting. They wouldn't let me go. They only let Jim go, because they knew that Jim wasn't a mathematician, and when he got up to present it, basically Eric Lander got up and just smeared him across the floor, because he couldn't argue like I would have argued if I had been allowed to be there.
You can see it's already getting interesting, right? I thought it was all kind of funny, because usually computer science and mathematics is very dry, it's very genteel, we're all very polite with each other. But in biology, man, there are real pissing contests, and they really fight. And it gets down and ugly okay? [laughs] When these guys think there are Nobel Prizes at stake. I mean, it's interesting. In one sense it was a lot of fun. In another, it was kind of hair-raising. So, I thought it was dead. I was going to Europe, and I was saying, "Look, let's try it on something like a fruit fly. Let's do a pilot project. Let's do a 1/30th scale project and see if it works or not." I couldn't get anybody to do anything. I was trying the Europeans because the Europeans were behind the Americans, and I thought maybe somebody would buy it, because they knew they were lagging. But I didn't get anything to go.
Then in 1998, Celera was formed, and Celera was formed exactly to basically sequence the human genome using the new Applied Biosystems 370 DNA sequencer, and it was to do exactly what I was advocating. I called Craig immediately, once I saw the company was forming and he made the announcement, to find out what was going on. Indeed they had studied my paper, my simulation, in terms of deciding what to do and how to do it. So to me, it was like PerkinElmer had decided to spend $300 million, to see if the idea worked or not. How could I say no? How could I not go? It was like, this is the opportunity of a lifetime. I proposed this thing, and I had to see it through. I thought about not going, actually, at one point. I was in Europe. I was doing some tours. I was thinking, "Well"—but I realized that if I didn't go, I would always wonder what would have happened if I had not. So I just wrote—"I have to go. I have to go do this, and I have to see it through, and I have to see whether or not I could do it or not. I can't let somebody else do it."
ZIERLER: You couldn't do this from Arizona? You needed to leave academia to some degree?
MYERS: I left Arizona and went to the company. I was one of the first 10 people in the company, employee number eight or nine or something. I stood in front of a building that could house a thousand people, in early August, with Granger Sutton, and that was it. And we were a thousand people, two years later. We had built the sequencing factory, and we had sequenced the human genome, all in a three-year timespan. It was an incredible run. I aged like 10 years in that three years, but like I said, it's another one of these things I'd do it again. Because it was just so—it was so exciting. It was like—I will always think of Apocalypse Now, the napalm in the morning; it was like the smell of napalm in the morning. Duvall walking down the beach with the bombs going off—I mean, that's how it felt every morning. I would walk in, and they'd hit me with something that would kind of make my stomach go, sssss! I ended up being a VP, and it was a lot of fun. I ended up making decisions, like to go with DEC Alpha instead of IBM, which made DEC Alpha millions of dollars because we used their computers. Anyway, I was meeting the president of IBM, of DEC. Suddenly I was on a big playing field, a very big playing field, and it was just incredibly exciting.
ZIERLER: On that point, why don't we make this a two-parter? We'll pick up next time when you start at Celera and really go through what was so exhilarating, what was the plan of attack for you. Let's do that.
[End of Recording]
ZIERLER: It is Thursday, August 10th, 2023. It is great to be back with Dr. Eugene Myers. Gene, once again, great to be with you. Thanks again for joining.
MYERS: Yes, pleasure.
Genetics as a Massive Computational Challenge
ZIERLER: We're going to pick up where we left off last time. We're going to bring the story back a little bit. Just for some context, by the time you joined Celera, was the company already operational, or was your joining part of its origin story?
MYERS: I don't know exactly what was happening in terms of incorporation and all of those kinds of things. I basically heard about the announcement in May. Craig got up at the Cold Spring Harbor meeting, the annual genomics meeting, in May, and basically goaded everybody in the public project by saying, "We're going to sequence the human genome at this new company that I'm forming, Celera." That happened in May. I heard about that through the grapevine, so I called him up and said, "Hey, I want in. I want to do this." To me it was as if PerkinElmer had decided to spend $300 million to see if this whole-genome shotgun idea was going to work. I believe in the first part I told you about the background, the fact that people didn't think this was something that would work, and yet, through a series of ideas and meetings, I had come to actually realize that it could work, if the correct data was produced, and had been trying to pitch it.
So for me, this was very exciting. I immediately called him up. We negotiated things in late May. I had to figure out what stock options were and a few other things. I was pretty much just an academic; I knew nothing about the commercial sector. Once we got through all that stuff, basically I tore everything up, I got an extended leave of absence from Arizona, and I think I was there like at the beginning of August. I can't remember the exact day. I think there were like 10 people. They had a building rented. They had a sign out front. They were obviously incorporated. We hadn't issued stock yet. So it was very, very early days. Like I said, the business team was about three or four people, the top people from the VC and PerkinElmer, and then there were about 10 of us technically. It started there. It was basically this huge building, which was eventually filled with a thousand people and 300 sequencing machines. But on the day I arrived in August, there were 10 of us! [laughs] So, early, but I didn't start the company.
The idea of starting a company actually was quite interesting. I'm not sure that people really know this story. PerkinElmer has been a prime supplier of electronic and measurement and chemical measuring equipment, and they had diversified by buying Applied Biosystems, which was the company that was producing sequencing machines, was the prime sequencing machine company. Earlier that year, they had bought a company that was producing mass spectrometers. A company in Boston; I forget [it''s name]. But along with that Boston acquisition, they got a very smart young guy by the name of Nubar Afayan. They were sitting around, and what was happening was Applied Biosystems was losing market share, because there had been a shift in the sequencing machines. They had been what had been called slab-gel, where basically the DNA just flowed down this big slab of an acrylic gel and it would wave and you had this problem of figuring out where it was, to basically having the DNA flow through capillary tubes that were loaded with gel, so you knew exactly where the DNA was, because the DNA was in each of these tubes. So it got rid of what was called the lane-tracking problem.
This was a very big advance. There was also more throughput, and it was also a more highly automated format. This was the next gen or rev of the sequencing technology of the machines. Amersham was ahead of them, had actually come out first, and Amersham's machines were being sold and were gaining market share and were displacing ABI machines, Applied Biosystems machines. So they were all sitting around going, "What are we going to do about this?" Nubar came in and said, "Well, if we took 300 of the machines that we're late getting out, we could sequence the human genome to 10x in one year". They said, "Why don't we go ahead and do that, as a loss leader, and as a"—how should one say—it's a loss leader. Basically, if we do this, if we form a company that's going to sequence the human genome with our machines, then everybody else is going to feel like they have to buy our machines, because they're going to be threatened by the fact that—and we'll gain market share. This was brilliant, I thought, really. I think people don't really understand this, because a lot of people look at Celera and say, "Well, they lost a lot of money. They didn't work in the end."
But actually from a business perspective, Celera was a raging success, because what happened is they formed Celera, Craig gets up—he's this kind of magnet for controversy—and says, "We're going to sequence the human genome, using 300 Applied Biosystems machines." Right? I mean, it's the best advertising you could ever have. "And then we're forming this company, with 300 of their machines." The thing that was interesting is this company was a PerkinElmer company, and then Applied Biosystems was also a PerkinElmer company. So PerkinElmer basically owned the left hand and the right hand of this play. To them, it was gravy if we actually sequenced the human genome. It didn't really matter. What mattered was that by forming the company, and proceeding in this way, with this audacious approach to shotgun-sequence it—because the whole idea was we were going to do this shotgunning, this protocol that everybody said wouldn't work, but it was the way you could get 300 machines to produce all the data, in a year's time, in kind of a highly automated factory. The amazing thing is that what we did is we actually leased our 300 machines from the company. We leased them; we didn't buy them. In the meantime, everybody, every center in the public center, was buying these machines. Applied Biosystems sold a billion dollars' worth of machines in the first year, which more than recouped the 300 million that they had put in to form Celera.
ZIERLER: Wow.
MYERS: Do you see it?
ZIERLER: Yeah.
MYERS: It's just sneaky as all get out, but it was brilliant. Because it worked. Then if we actually sequenced the human genome and did manage to become the Bloomberg of genomics, which was kind of the play—because the idea was we were never going to make—there was a lot of people saying, "Oh, you're going to hold this thing, and nobody is going to see the human genome." No, that was never the plan. The plan was basically to make the genome available to everybody for a very modest subscription, and basically be the Bloomberg of genomics, have everybody come in and check out our data because we had a beautiful human genome and data thing around it. There were some problems. The company was very strong in terms of the actual execution of producing the data. Then they had me to put it all together and produce an assembly. We had very good people for doing the annotation and interpretation.
But we were not Bloomberg, and the people we had—mea culpa—but the people we had I don't think rose to the occasion, to basically produce a—the other problem, honestly, was that there was this perception that the public project had produced something of equal quality and it was free. It wasn't of the same quality for quite some time, but eventually became of the same quality. But I think there was a couple of years there where the product and the reconstruction of the genome we had was much superior. If you read the tea leaves, you can actually see evidence of that. But there was the public perception, like, "No, I don't need to buy this thing, because it's going to be free." So that part of the company never took off, unfortunately. But like I said, it still wasn't a loss. [laughs] It had already made a ton of money, before—and that's not even to mention the stock price, and the shares that were sold that also made a great deal of money at one point, although they—they didn't deliver to shareholders ultimately, which was very—but the other part, about selling the Applied Biosystems machines, that part was brilliant, and that was real money that was made in a real way.
The company was really—it was controversial. Nobody said we could do it. I remember this cartoon in The New York Times where they had a big pile of jigsaw puzzle pieces, and the guy was holding a box that said, "the human genome," and the idea was, like, what are they going to do with this puzzle? There were a couple of guys in lab coats with their book and the big, big pile of pieces, kind of implying that it couldn't be done. Because it was effectively a 50-million-piece jigsaw puzzle of sorts, and people just didn't think you could do that. It's really interesting that there's kind of this analogy to real jigsaw puzzle numbers, which is that the way the Human Genome Project, the public project, was working, was that they were going to manipulate pieces of DNA that were about 150,000 base pairs long, and sequencing those represented a 1,500-piece puzzle, exactly the kind of size that human beings do. We do the 1,000-, 2,000-piece puzzle. But there was this concept that the 50-million-piece puzzle, just you weren't going to get it right. But what people really missed was that a lot of the genome was unique.
There were lots of parts of the puzzle where the pieces were unambiguous, as it were; there was only place they could go. It was the little corner turret of the castle. Pick that one thing in the puzzle that looked like that. Fundamentally the problem was that if you could put together all of these little islands of unique stuff, the problem was how do you get them ordered. You have a puzzle, but you have 100,000 unique islands, and you have no idea what their order was. That was solved by this kind of paired-end whole-genome shotgun approach, so that the modification that Weber and I had proposed earlier, before Celera formed, was instead of collecting the data as individual sequencing reads, what you did is you produced a longer insert—you sized a piece of DNA about 10,000 base pairs—and you sequenced 700 letters from both ends. What we ended up with is data that wasn't just 700 base pair reads, all individual, but pairs of 700-base-pair reads that were at a known distance from each other. We called that paired-end whole-genome shotgun. It was that data that then allowed us to actually order and orient these things and actually solve the bits in between. I won't belabor it more, but it wasn't just a straight shotgun as it had been practiced. There was this very important modification of the protocol that we were producing paired-end data.
To me, like I said, it was a fascinating time. Oh, that's right, the last time I was talking about Robert Duvall and Apocalypse Now and the smell of napalm in the morning, which is exactly how that first year went. I'm really in some ways more proud of what happened at Celera than I am of the BLAST thing, because the BLAST thing was just me being an algorithmist doing my core thing, and while it was a very important algorithm and was very efficient for its time, it was kind of my thing, whereas at Celera, I had to do a lot of stuff that was way outside the comfort zone of a traditional academic. Suddenly, I'm in the corporate world, and there's nobody at the company that knows what computers to order. I'm an algorithmicist, I write code, but I don't—but at Caltech, I was one unit shy of an electrical engineering degree. I knew how computers worked. I knew what to order. So I ended up basically configuring like a $5-million-dollar data center, $10-million-dollar data center at the time. I was being courted by DEC Alpha. I was being courted by IBM. I was meeting the presidents of these places and being wined and dined, because they wanted my contract. I'm just this kid from Arizona. I'm an academic, associate professor. The next thing I know—so I made that decision. I actually picked DEC—Compaq at the time—and they made a ton of money on the basis of the Celera—the press they got.
That decision wasn't political; that decision was technical. We actually tested the machines. This was just at the time that computers were switching from 32-bit words to 64-bit words. Typically an integer was 32 [bits], which means you could have numbers up to four billion. But that's a bit problematic when I was going to produce basically 30 billion bases of data. How was I going to index 30 billion—so it would have been much harder if I had had to do it on a 32- bit machine. But it was just at the time when 64-bit architectures were coming in. Which now, you're into 16-billion-billion as the largest number, and there's no issue. The memories were getting big enough. You have to go back to 2000; it's amazing what the machine I'm talking through could do now. But back then, that was a big deal. So, we evaluated the DEC machines and the IBM machines, which were really the only ones that were available, for their 64-bit performance, and the Alpha won.
Although it was a very new chip. I remember at one point, it was—yeah—it was a year later. We had the center operating. We had all the new Compaq machines, the DEC Alphas there. We were trying to do the assembly of the fruit fly. The pilot project was the fruit fly. I knew this was going to happen. One of the problems I had was that I had no data, and I had to develop a very large computer program to do this, on a data set that had never been generated. I knew what was going to happen, which is that the factory, there was going to be nothing coming out of the factory. And nothing came out of the factory for 11 months. Mark Adams was in charge of building the factory, and he did a great job, but there were problems with the machines and things didn't work. So it went from basically zero to being a deluge of data. Once everything started to work, it's like suddenly the fruit fly was sequenced in three weeks. Literally I went from like no data on the fruit fly, to, "Here's the data set, for the fruit fly," three weeks later. I knew a year before that the day that that happened, they were going to come and say, "What's the assembly?" [laughs] So I had to have an assembler that could run on the data, practically the day the data came out. I wasn't going to get like six months after they produced the data to play with it. I knew this was going to be a problem.
There were a couple of interesting points there, potentially interesting points. One is that—the way I solved that problem was I used simulation. Remember I was walking around with my box of cards at Caltech? I guess there's a lot of concilience in all this. I worked on simulations back when I was an undergrad. Not the same kind of simulations, but we had sequenced like two, three percent of the human genome at that point, so we had a pretty good idea of the statistical properties, how many repeats there were, and things. I could make pretty good genomes and I could basically simulate the shotgun process. I could simulate the errors that occurred in the reads, and the rate at which things would get lost in the factory. The way I did it was I recruited my team, which didn't know anything about this particular problem, but I broke it down into a collection of phases, of steps, that had to be performed, in kind of a pipeline. Then what I did is because I had simulated the data, I knew what the answer was supposed to be, after each step. In other words, I had ground truth. So, everybody who got an assignment could basically write their code and then see if they were doing the right thing, see if they were producing the right answer, and to what degree they weren't producing the right answer. So, simulation really was a key method for developing the assembler, because literally we had—I think we didn't do it immediately, but we had an assembly within three weeks of the thing [delivery of the data], a good one.
There was a crisis where things weren't working at all, for a while. We couldn't figure it out. It was like everything had been looking good. It turned out that in one stage, somebody had forgotten the flip, that reads could be flipped. And that was it. Once we corrected that, then the thing worked. But I remember there was like a week where we didn't know what was wrong, and I'm pounding on the guys, like, "We've got to figure this out!" Everybody on the team was like trying to figure out what's going wrong. Because we knew something was going wrong. It just somehow wasn't finding all the ways that the pieces were sewed together. It was because the person had forgot to think about the fact that a piece of DNA can be in the forward direction or can come from the complement strand, and he hadn't put in the complementarity. It was a bit surprising, because it was somebody who was very good, actually, one of the better and more experienced people that I had, that they made that error. But we found it, and then it worked. But simulation was a key strategy, actually, to being able to deliver something that worked. The simulation was good. It pretty much—the data pretty much—there were a few things that we had to adjust for but nothing really significant. So, there was simulation as the way of basically doing it without having the data.
The other thing that was happening is we were trying to get the runs done, at one point, and the machines kept crashing. There was something wrong with the Alpha. I never did—still to this day I don't quite know exactly what was happening. It was some kind of race condition or overheating problem in the CPUs. But it was one thing when we did our test and evaluation; the machine ran for three hours. But when you're running 100 cores, 100 nodes, and there's some kind of low failure rate, then over the period of 24 hours, you're going to get failures, which meant the whole thing came crashing to the ground. Because we're trying to get this thing done, and we couldn't get it done, because the machines kept crapping out. So [laughs] it's the only time I think that I've ever used anger effectively. Almost every time in my life when I get angry about something, it's not appropriate and it's not effective. But I actually went down to the IT Department, which at this point had about 40 people. This is a year later . I got up on a table, and I yelled at the top of my lungs and said, "God dammit, these machines have gotta work! You've gotta make these machines work! These things are currently a piece of shit! Why isn't Compaq down here fixing this problem right now? We are gonna sink, if you don't fix this thing!" Something like that. I was like at the top of my lungs, I screamed at everybody in the room. Then I kind of woke up and walked out [laughs].
But, Compaq came, realized—basically the reason it was effective was because it made everybody realize how important it was, and that this wasn't the kind of a problem where they had weeks to figure it out. It had to be figured out now, because I've got the biologists breathing down my throat wanting an assembly. We're on the clock. Everybody is looking at us. There was lots of press. We had to get this thing done. What it did is it made Marshall, who's actually my friend, realize, "Wait a minute, this is important. Why aren't the guys from Compaq here?" They've already made a shitload of money off of the advertising that we're using their machines, but what if it gets out—I said, "What if it gets out that their machines aren't working?" [laughs] What will that do to them? So they were there the next day. A lot of [their] people were there the next day. And they had it figured out, within three or four days, and we got new processors, and by the next week, everything was okay. I never knew exactly what the problem was. I had enough things to worry about besides that. But that's the only time—I thought that might be a fun story for you. It's the only time I think I ever got angry and it actually did some good. [laughs]
ZIERLER: What aspects of the BLAST research were relevant when you joined Celera? What could you draw on from your previous work?
MYERS: The BLAST—in terms of the corporate experience and navigating the pressures and the deadlines and understanding when things were important, I don't really think it pertained. The BLAST experience was important because I understood in detail how to write exceedingly efficient codes for strings, and how to compare strings. You had to take all of these pieces and compare them against each other to see how they might go together, and we used a BLAST-like approach for that particular phase. But that was only one phase of the total problem. That is where my previous experience with BLAST was useful.
Competing With Government Agencies
ZIERLER: Gene, what about interface with government agencies—the DOE, the NIH? Were you working with them on a daily basis at Celera?
MYERS: No! It was a competition! We were competition for the public project.
ZIERLER: Wow.
MYERS: We were considered a competitor. There was a lot of talk going on about how Celera was going to sequence the genome and then lock it all up in patents, and it wouldn't be free. The public sector guys were saying, "Well, our stuff is going to be free." Which is really very self-serving, because it was government money anyway; I would expect that the taxpayers would expect [laughs] that a product of their money would be free. But they were putting it out there like it was some virtue on their part, and not [laughs] a government mandate. They were pitching that we weren't going to do that. We had said very clearly that we would look at—we expected that there would be about 30,000 genes in the human genome, and we said, provisionally, what we'll do is we'll look at the genome, we'll take 3,000 that look important, and we'll file provisionals on those 3,000, and then we'll follow up on those, and if we don't have anything within a year that portends a real use other than—because you can't really patent nature, anyway.
That was the decision, is it's a naturally occurring thing; you can't patent genes anyway. But you can patent a use of the gene. So we were going to put provisional patents on these genes that were kind of where the use was vague, then basically get it down to 400 or 500 that maybe were worth—where we had some idea of what they were actually doing in the cell and were potentially valuable. It was a bit of a compromise, right? The company couldn't say, "We're going to absolutely—" but the idea was that we were going to make the genome, we were going to make the initial human genome. We made it completely free. We gave it away. We didn't keep it. We gave the sequence away. We said we were going to do that, and we did that.
There were a lot of people that didn't trust what was being said. They didn't trust Craig. That's not my problem, but they didn't trust Craig. They didn't trust the statement about how many genes we were going to take. But it was kind of ridiculous, because there was this other company—I'm forgetting [it's name]—that was doing cDNA cloning, that was basically—what the hell was it called? Sorry, I can't remember what it was called, but there was a company that had been formed previously using cDNA technology, which was just another way of capturing genes, and they were capturing genes in the human genome, and they were patenting them left and right. They patented thousands and thousands of genes. Not one peep of noise about them, but they were making a big stink about Celera. Really, I was thinking that they should stop that company that was patenting those genes, because what they were doing really was a land grab.
There was this kind of contention between—I was basically a public sector guy. I was doing this for the science, and I was doing this because I wanted to do it, as a public endeavor. I had been assured that we were going to be allowed to publish, and that the human genome was going to be made freely available to everybody, and I believed that stuff. It was interesting; there were some compromises in terms of being in the private sector, but we pretty much did what we said. In fact I don't think Celera ended up at the end of the day holding any patents on any genes, after it was all over. And we did make the genome available. But at that time, there was a lot of fear that somehow the genome was going to get locked up in a private company, and the public sector guys were playing that up to the hilt. Because a lot of these guys thought they were just going to—they had all already lined up—and there was Lander, and there was a guy at Baylor, and Waterston, and Sulston—and these guys were all lined up, and they all thought that they were going to basically march to the end holding hands—"Kumbaya, we sequenced the human genome"—and they were going to pick up their Nobel Prizes.
So, it was very high stakes. It was really interesting to see what was going on. I felt like I was just a level below that. I was just kind of the guy in the boiler room. I was trying to keep my head low and just do this thing. I had to get this thing done. So it was very interesting. I'm glad I didn't have to engage in the politics. Craig was the one who was fighting the press wars with those guys. I didn't have to deal with that. I don't do well with that stuff, anyway. I'm an introvert, and it was a little bit too much for me. It was enough, the challenges of—like I said, the napalm in the morning, the decisions I had to make, and the magnitude of the decisions I had to make in those first six months, were gripping, for me, personally. I hope I gave a flavor of some of them. I aged a lot, actually, in that four years. It was nonstop.
ZIERLER: You mentioned it was initially a temporary leave that you took from Arizona. At what point did you formally leave Arizona?
MYERS: Not until actually I left Celera and went to Berkeley.
ZIERLER: They were willing to keep you on for the whole four years?
MYERS: Yeah. I negotiated that I could come back after it was over. I think there's a book by Jamie Reed where he made it dramatic, like there was no going back, I think because it made the book sound better. But no, I'm a more cautious cat than that. I had negotiated a return.
ZIERLER: What was achieved at year four where you felt like there was some completion and you could move back to academia?
MYERS: We were finished at year three, actually! We were finished at year three. We had done the human genome. We had the human genome paper out. The whole thing was done in three years. I stayed another year, because Craig asked me to stay on. I was ready to go. I was ready to start looking for an academic job and try to return. The good news was that we had been successful. There was a lot of jockeying for whether or not what we had done was successful. A lot of the public sector guys were trying to say that we couldn't have done what we did without their data in addition. I can tell you about that if you want. The waters got muddied in a way that made me very unhappy. But it was basically done after three years. We had sequenced the fruit fly, the human, the mouse, and the mosquito, in three years. Which to me says exactly how efficient the process was, right? After 10 years, they had sequenced 3% of the genome in 1998. In 2001, human, mouse, fly, mosquito—done. It was a real revolution.
What I say is there was a lot of jockeying about whether or not it worked or not. There were papers in PNAS, deceptive papers actually. The problem was that the argument was occurring between biologists, who can't compute their way out of a paper bag. Forgive my insult, but why were a bunch of people who were not analytically trained trying to pass judgment on something that was intrinsically computer science, and was intrinsically computational? The people that should have been making this assessment were never asked. Because it was about the human genome, and it was about all the molecular biologists, and they were the ones who were all fighting and claiming for the greater glory. So whether it worked or not, they weren't the people to be saying, to have the skill set to even know [laughs], or to argue in a cogent way, which I thought was really funny. I kind of watched the whole thing. But there were PNAS articles that went back and forth. But look, the truth of the matter, David, is that basically a year later, the public project started doing it our way, and they did everything our way ever since. To me, that pretty much tells you whether or not it worked. [laughs]
We did the fruit fly. The happy part of the story, honestly, was when we did the fruit fly. We did the 1/30th scale model. The fruit fly is about 200 megabase pairs. It's about 1/30th the size of the human genome. What was nice about it is that it was cooperative. Gerry Rubin had been sequencing it. Instead of fighting us, he joined us. Sage move. Then it was kind of part of us, we basically had a wonderful paper about the fly. We got a little award for best paper in Science, and everybody saw that and said, "That's a beautiful genome." All the fly geneticists were happy. They had the DNA sequence of the fly. Years early; they weren't expecting it. It was a big celebration and we were all happy and everything, and there was no—but then in the next year, we continued to compete for the human, and we were sequencing the human, and it got really—to my mind, it got really ugly. At the top. Because these guys, like I said, they thought Nobel Prizes were in the offing. They went after each other, in a way.
One of the very interesting things is the sociology of science. Computer science and mathematics, it's very beautiful; when we review each other's papers, the reviews are objective. They're about whether or not the theorem is true or not. Is the proof correct? And a few helpful suggestions about this or that. When you get into biology, man, you get people that just—they don't like the paper, and they just rip you one. It's, "I don't like this. I don't like that. They didn't do this. They didn't do that." Duh-duh-duh-duh-duh. It's kind of like—they're savages, basically [laughs], from my perspective, in the way that it's done, because a lot of it, you get one guy who has a bad opinion but a substantial reputation, and you can have a hard time having your paper published. So it's very interesting how different the cultures are. The review process—I've never done that myself. I've always tried to adhere to the highest standard. If I review an article. I might make a comment about whether or not I think a piece is worthy or not, but I always tend to focus on whether or not it's correct, and whether or not it makes a point, and what's the value of that point, in as objective terms as possible. I've always felt that that's the way that it's done. But it doesn't seem that that's the culture in the biological sciences. I also think that physics is kind of more like mathematics—the more quantitative studies where there's more objective truth, and an analytic event, than in molecular biology. It was really something, to see the arguments, and the histrionics that took place at that time.
Yeah, so the sequencing of the human genome and the subsequent achievement was not really that happy for me, because there was so much arguing about whether or not we had done it, and negativity towards us. Mostly it was aimed at Craig, because they didn't want Craig to win the Nobel. But this was my work. I feel like it was the best work I ever did, in the sense that it had a broader scope than BLAST, and it really was a novel approach, and a surprising result. I did something that people didn't think could be done, and I wanted to be proud of it, yet I wasn't getting any kudos. I was a hero within the company, but in the public sector, nobody was saying anything good about it. It was very hard for me.
ZIERLER: What is the source of the argument about whether the genome was completed or not? How is that not an objectively—?
MYERS: Well, it wasn't completed. It was sequenced, and it was as complete as you could basically get it with the technology we had at the time. We produced about 90%, 95% of it. There were holes. It's interesting—today—today we can actually sequence the genome from telomere to telomere. We can produce a contiguous single sequence of every chromosome. But 23 years ago, we could not do that with the technology that was available. The idea was to basically get most of the euchromatic genome, the part of it that's not the centromere or the telomere, which are highly repetitive parts that don't contain many genes anyway, but they have basically kind of the sweet part of each chromosome arm sequence. So it was about 90% of the genome, and about 99.8% of all the genes that were interesting for medicine, and to have a good idea of what the construction was and where the genes are, and what order they are, and have all that stuff. So it wasn't a perfect genome. None of them were. That didn't happen until just recently, in fact. [laughs]
What happened is—so we've sequenced a fruit fly. Everybody's happy. It was a beautiful success. At that point, I knew that, look, the human genome is 30 times bigger, but it's going to work. No problem. So we're sequencing along—ch-ch-ch, ch-ch-ch, ch-ch-ch—it's the human genome. We produced about 5x data. For a variety of reasons, you need to oversample by about 10 in order to get a good reconstruction. You need at least seven or eight to get most of it. But the target, the level that I had always specified in my protocols, was 10, and I thought we were going for 10. This is the part where being in a corporation sucks. It's pretty expensive, right? We've done 5x; it has cost, I don't know, $25 million, and it's going to cost another $25 million to generate the other 5x, and about six, seven more months of sequencing. This, I can't tell you how upset I got about this—somebody in the business office gets the bright idea, like, "Look, the public project, all their data is freely available, and they produced about 5x of the human genome. Why don't we just use that and save ourselves $25 million?" [pause] Because I knew that that was going to completely muddy the waters about whether or not we actually put it together, independently, or not. That's what happened, basically. The corporate guys won. We couldn't fight them. Most of the people at the company didn't care that much, but I did. [laughs] Because I knew that it was going to create this problem, and I really wanted us to produce the data and produce the genome in a clean way.
We took all the public data, and what I did is I chopped it up into pieces. I took the assembled data that they had. I took their stuff. It was about 5x, and I chopped it up to create a kind of another 2x of data, and we assembled it, 7x, with that stuff. We did solve the jigsaw puzzle. But, see, it opened up all kinds of speculations. People were speculating that we cheated. That we actually used the fact that we knew the order, on how those pieces that we took from the public went together, and that we were using that. And that, yeah, without their data, we would not have been able to do the assembly. The truth of the matter is we needed their data to get a relatively complete genome, because as I said, we only produced 5x, and we really needed 10. But we did assemble it, legitimately. We took their data, we chopped it into 700 base pair reads, threw it in the machine. In fact, their data actually degraded the quality of our assembly, because of a very small problem, which is that the pieces that they had put together, their error rate was they had a misjoin every 35 to 50 kb, which is just horrible.
I mean, their initial genome was just awful. This will never get recorded, but it wasn't very good. In fact, we showed in our paper, when we compared our data to theirs, that their reconstruction basically—they had the genes kind of right, but they had pieces that were reoriented, and bad joins and stuff, and so they had like sections that were 50,000 letters long that were correct, but then they'd be joined to something not next to it, and then the next thing. So there were large sections that were coherent, but the order and orientation of all the pieces was bad. Then if you think about it, what I was doing was I was basically taking one of their things, which has one of these misassembly points in it, and just breaking it into 700 base pair reads. Well, that read that covers the break point, that's not a read that occurs in the genome. That's what would be called a chimeric read. I didn't realize this until later, but basically by doing what we did, we introduced thousands of chimeric reads into our dataset, which basically degraded our ability to put the puzzle together. We would have done better, in some ways—in fact, we did, internally, inside the company—we didn't get to publish it, but we did another assembly with just the 5x, in other words just the very light coverage data, without the top-up, and we had much better longer range coherent assembly. But it had more holes in it. It was coherent and it was long, but it had a lot of holes in it because we didn't have enough data. You need enough level of coverage.
So really, it was a crime, as far as I'm concerned. God, I'm reliving this whole thing now, and it's like [laughs]—sorry if I get emotional. But that was so hard on me, David. I mean, it was just so hard to have to deal with that. I understood it—to some business guy, it seems like a sage decision; let's save $25 million, and let's use their data, it's free. But, man, from a scientific perspective, it screwed up the whole thing. That's where the whole pissing match started. But we did the mouse without them, and that looked beautiful. We were actually able to sell the mouse for a long time. Because we had the mouse done like six months later, and we were doing subscriptions. People were buying those because the public sector mouse was nowhere in sight, and they were over a year and a half behind us when they finally got the mouse done. And guess how they did it? They got it done eventually because they used our method. They switched. They basically [laughs]—so it's like, did it work or not? Well, of course it worked, because everybody does it that way now. I don't know. But it was really hard for me emotionally, to be kind of accused of cheating, and kind of—whereas, I just—I've always just wanted to do the best thing that I could, and get a pat on the back, and have somebody say, "Well done." Which is kind of why I like the DAA Award; it's like a pat on the back. Maybe I'm not that secure. I guess I still need to be told I'm okay. [laughs] It was very hard for me, the human part. Like I said, up to the drosophila part where we did it and everybody loved us, it was great, but after that, the whole pissing match.
In fact, I basically left the field after that. I went to Berkeley. Craig asked me to stay for a fourth year. Because it was all done, and I was going to start looking for a job in academia. I looked at a bunch of places, and I ended up going to Berkeley at the end of the day. But I said, "Okay, I'll stay here, for you." Because it was such an intense thing, that there was this kind of—it's hard to describe—there was this bonding experience. Craig is kind of a bigger than life—he has his faults—a bit braggadocio, a bit—but he has a beautiful way of crystallizing the essence of something. He could talk to reporters. The thing watching him is he could talk to reporters in a way that they would understand, and he could state things in a way that was eminently quotable, and that you would be interested in, because they always had like a little—they were a little edgy always. I got to know Craig quite well, and he did have honor. He was very protective of his people. He was loyal to his people, the people that worked for him, and I think he treated us really well. He was kind of outrageous at times, but he was pretty much—basically he's the lion. The Bible talks about the lion and the snake; Craig is a lion, not a snake. There were a lot of snakes involved in this thing, but Craig was not one of them.
Genomics Success and Return to Academia
ZIERLER: You mentioned you basically left the field. Is that the explanation for why you went to Berkeley and not back to Arizona?
MYERS: No, I went to Berkeley because I had a chance to go from being in a number 35 department to being in a number one department. Literally it was just my star had risen, and I was able to go to—Berkeley is one of the top five. I thought, "I should go ahead."
ZIERLER: But when you say you left the field, what does that mean? What did you take up?
MYERS: No, I left genomics. I did not leave science, or I did not leave what I was doing, but I left the whole area of DNA sequencing and genomics. Not immediately, but in 2005, I switched to basically working on image analysis, and images, and what you could do with microscopes, and I completely switched out away from DNA sequencing and studying genomes and looking at genomes.
ZIERLER: How did you get interested in imagery and microscopy? What was the connection there?
MYERS: Actually I never finished the thing—Craig asked me to stay, and out of loyalty I said I would, and then they fired him, basically, three months later. The recruiting season was over, so I ended up spending a fourth year at Celera, basically keeping my head low, until the next recruiting season. So, I was there for four years. That's why I stayed. We were done after three. I was ready to go. I chose Berkeley because it's a great school, great people, great department. My star had risen. I was basically able to play at a higher level. I also think that I was also confident enough about myself and my abilities that I felt like I belonged at a place like Berkeley. Like I said, I had a lot of doubts at the beginning of my career about whether or not I had the right stuff or not.
Part of the reason I went to Arizona was because I knew it was a place that I would thrive. For me, the right strategy was to be a big fish in a little pond, and not the converse, so I did not go to Cornell or the other places that I could have done, because I knew that psychologically, I needed to be comfortable. I needed to feel like I was okay. I did not want to put myself under intense pressure to perform. But then of course Celera happened and I was under intense pressure to perform, and I made it through that, and I think that experience, and also the level at which I was playing the game, basically gave me the confidence to finally go to a place like Berkeley, a top-tier university, and say, "Yeah, I belong here. I'm taking my seat."
It's an interesting walk. I hope the story is interesting.
ZIERLER: It is!
MYERS: To me it's just amazing, the way it went. I could still be at Arizona! [laughs] But I went to Berkeley, and I didn't really have a specific plan. I was looking for—there's the book, The New New Thing—I was looking for the new, new thing. A lot of people were talking about comparative genomics, which is the idea that now that we can sequence these genomes, we start comparing them to try to get insight into what's common and what's different, and based on that, begin to divine things about the molecular function of cells. That was all very obvious. I knew how to pursue that. But somehow or other, it was like too obvious, and I just didn't feel like we had good enough data, like the genomes were sweet enough for that.
We had very good human and fly genome, but we didn't have many genomes. In fact, I tried to get—I got a dozen flies, species of flies sequenced, and they weren't done well, and it was kind of like—anyway, I had been pushing on that, and I worked at Berkeley for a few years, but I was basically kind of waiting, because I knew that HHMI was going to form this new intramural institute back in D.C. I had already talked to Gerry Rubin and knew that it was coming and that they were building it. I went to Berkeley in the meantime, and did some good work, wrote some capstone pieces on how to do the assembly and stuff, and wrote up some of the things I hadn't had time to do while I was at the company. It was a wonderful place, wonderful colleagues. I really enjoyed my time there. But in 2005, Howard Hughes Medical—you know what Howard Hughes is, right?
The Foundation for Medical Advances
ZIERLER: Sure.
MYERS: It's the largest private funder of biomedical research in the United States. They opened this $600 million intramural institute nestled in the hills by the Potomac River, as it flows through to Northern Virginia, just outside of Washington D.C. I joined. I was like the first seven investigators that they had there. We were called group leaders, a very subdued title. The mission was literally to decode the brain of flies, mice, and other things, by building models of them with electron microscopy and light microscopy. I became very interested in light microscopy. Now, I wasn't that interested in neurobiology, per se; I was more interested in what you could do with a microscope.
The reason I was interested in that was because the biggest outcome of having these genomes wasn't that we suddenly understood everything that was encoded in them, and we could cure cancer; more what happened is that once you have the sequence, now you have access to any part of the genome for genetic manipulation. In other words, to genetically manipulate a piece of a genome, you have to know the sequence there, so you can do kind of recombinant genetics there. We knew how to do recombinant genetics; we just didn't have the genomes, so we didn't know what to target. But now you could take any gene, or any part of the genome that you were interested in, and you could manipulate it. In particular, one of the most important, you could knock it out, you could knock it up so that you could get more of it, or you could do things like add a fluorescent molecule to the tail of it so that it glowed. Imagine there are 30,000 genes in the human genome, and suddenly I have the ability to say, That gene there, make it glow." In a human cell. And this has been—so this ability to basically say, "take it out," "bring it up high," or "make it glow," or modify it in some way, has allowed us to really, really change the rate at which we were able to discover things.
The Human Genome Project, it didn't get us really explainable code. We're going to be working on that for 40, 50 years. But it gave us exquisite access to the human genome, to the mouse genome, to the fly genome, to any critter that we had a genome of. That has allowed us to discover a lot of things. Because if you can light something up under a microscope, then you can watch it. You can see what it's doing. You can see where it is. You can see who it's hanging out with. Because you can light up a couple of things, one in red and one in green, and see if they're near each other. You could do a lot of things, suddenly, with the genome. That was the big thing. What I realized was that when I looked at all the papers, the primary mechanism of discovery involved a picture, a microscopic picture of one of these things glowing, and that there was no computer science for analyzing these images. There was no way to track particles, or identify cells and watch them move around. And there were things that were lacking in terms of the microscopes themselves.
Initially, I thought, well, what I want to do is I really want to focus on what we now call bioimage informatics, which is I wanted to look at electron microscope and light microscope imagery, and basically try to extract models and information from them. Much harder problem, different domain. I had to completely retrain myself. I went to Howard Hughes, where that's what I did. A lot of my time was spent working on building models of neurons and segmenting neurons, but it was basically the same problems, looking at microscope images, trying to understand and building models of what we were seeing in the images. I was also captivated—I also found that I wasn't that excited about academics anymore, academic institutions. I don't like teaching that much, and I didn't like all the committees. I didn't like raising money, writing grants, trying to raise money for this or for that. I guess I was spoiled by Celera. I had a $10 million budget my first year, and I got whatever I wanted, when I wanted it. I had a mission, so I could go about it.
Suddenly I'm back in this place where I have to beg for half a million dollars to do something, and I have to be questioned by [laughs] a bunch of people about whether I'm any good, or whether I can do it, and blah blah blah blah blah, and i just was like, "Nah." I hated chasing money. I don't mind teaching, but it's not my thing. I don't get fed by it. I enjoyed the research. Berkeley had tremendously talented postdocs and PhDs. That, I enjoyed very much. There were a lot of smart people to hang out with. But otherwise, it was kind of like—and I wanted to do something that was more exciting. There's no sense of urgency in academia, there's no timeline, whereas at Celera, it's like, "As soon as those machines are up and running, we want an assembly. We need a piece of code that solves this problem." There was a real objective, and an intense timeline, and we really had to go after it. Everybody was on mission, working furiously, and that was exciting. Academics just seemed too sleepy after that. Nothing against academics—great—it just felt too sleepy for me, after my experience. I wanted something more exciting. I wanted another crucible event.
ZIERLER: Did HHMI do that for you? Did it provide that sense of urgency?
MYERS: No, no, but I was hoping it was going to be more project-oriented, more interdisciplinary. The other thing that I really liked is I liked working with people. Celera was also the place where I really worked with other people. I worked with my collaborators. It was really an interdisciplinary project. It was not me, in my office, sending grad students out to talk to people around the campus. It was me literally engaged in doing something that was intrinsically interdisciplinary. I liked that. I went to HHMI because I'd be hanging out with neurobiologists, with physicists, electronic engineers. The idea was to really interact. It was partially successful. They got some interactions. I don't think it's worth, for this history, to go at it. I was there for seven years, and it was a very good opportunity for me to be a postdoc and retrain myself in the methods of image analysis, because image analysis is quite a bit different than sequence analysis. Sequence analysis is all discrete mathematics, whereas in image analysis, it's all the underlying methodologies, continuous mathematics, and so most of the concepts come from the continuum. Really I had to retrain myself substantially in a completely new repertoire of techniques and methods. I also had—oh, come on—sorry, it's just old age—who won the Nobel Prize?
ZIERLER: What year?
MYERS: It was a few years back. He won it for super-resolution microscopy. Eric Betzig. Thank you. Eric Betzig, yes. You connected—the right connections got established. Eric Betzig was like in the next pod over in this beautiful laboratory. He was developing STED, for which he won the Nobel, arguably. I worked with him on some of that. I wrote some of the software for him for the interpretation of the imagery. Actually there were a number of microscopists there, and I just learned enough microscopy to be kind of dangerous as an engineer. While I was there, I also built a microscope. We engineered a microscope. I'm not really a physicist, but we built a microscope that could basically image an entire mouse brain at micron resolution in a matter of days.
Basically it was a big robot. It was a microscope that was a robot that was scanning at an incredible rate. It was scanning at 16 million hertz, which is incredible. We were using an oscillating galvo. It was an engineering piece, but I had a super-fast scanning thing, so I could do volumes, and then we had this robot—you would put a brain on there, in fluid, and it would be sectioned, and we would be finding it, imaging it. In three days, we would have basically the entire volume of a mouse brain. I think it was eight trillion voxels. I built that and realized that I could engineer a microscope. That was an interesting time. I learned a lot of things. I think for the first time in my life, I understood the Fourier transform, really understood it actually. So, HHMI was—I would consider—I hope it's not too pejorative, but Berkeley was kind of a resting place to recuperate, and get my bearings, and understand where I was, and it was very good that way. I'm very grateful for my time there. I was hoping at HHMI, we were going to do some big things. We were going to actually build a complete model using light, of a fly's brain. I spent a lot of time working on that. But they were very top-down projects, and they weren't happening with much urgency, and I didn't feel like I had any control over what was happening. I was fine as an individual investigator. I won't go into why or what, or whatever, but—
Microscopy and Janelia Farms
ZIERLER: What's the organizational structure? Who is setting the research agenda at HHMI?
MYERS: At the intramural institute, at this facility, Janelia Farm Research Center, the director was the one who controlled the large resources that decided where projects went. He had a lot of control over the projects, and it was not democratic. I had influence, but no direct control, per se, in what happened, other than what I do with my research group, where I was free. And we had fun! We did some things, some reasonably good things. I was actually hoping that this image analysis stuff was going to become a big thing. To be honest, I was looking for my third home run, which I didn't find, unfortunately. I consider BLAST to be a home run. The human genome was a home run. I was hoping for one more. It just never quite panned out. I wasn't able to find a—we did some nice things, but nothing—some base hits, and some second base hits, to use the baseball analogy, but not a home run. Which is too bad.
ZIERLER: What would that have looked like, Gene? What would a home run have looked like in microscopy at HHMI?
MYERS: Something that everybody uses and that has thousands of citations like BLAST. I was hoping basically to develop a method, a microscope, a result, of an image, of something, that basically had that kind of purview. If we had been able to build a complete model of the fly's brain with light, in a reasonable amount of time, that could have been it. That would have, I think, had that kind of play. But it was very difficult to do, and it has taken years. It has produced results, but it's still not finished. So it was too hard. It was a little bit too hard, to actually—that would have been a good result. Another one would have been just a piece of software that solved a critical problem, but it turns out there there's no kind of generic problem like there is in BLAST, like "Find something that's like this sequence." It's all unfortunately very customized, very dependent on the instrument, and the way that you find the data.
At one point we had a method based on deep neural nets to do deconvolution to actually crispen up images. We did that about three or four years ago. How many citations with that? Has that reached a thousand citations? I don't know. I don't think it has gotten over a thousand. To me, you have to be over a thousand to be—well, cell detection with star-convex polygons, that got up to 676. That's pretty good. Our viewer got to 735. The BLAST paper has over 100,000 citations, and the human genome paper was 20,000 citations, so those are home runs. A thousand is a double or a triple. So, yeah, I was hoping for something with that kind of impact, where there would be thousands of citations. That's pretty much it, right?
I think I'm mostly known for those two papers. I think you could take my top 10 papers, remove all the rest, and it wouldn't make any difference in whether somebody knows me or not. It's kind of interesting that way. It's not a game of massive numbers; it's the home runs are important, because those are what—when I go someplace, everybody knows, "Oh, you're the BLAST guy," or "OH, you're the human genome guy." That's what I'm known for. "Oh, you're the star-convex polygon guy"? No. [laughs] No. Anyway—although that was a good paper! I got to a level, I think, with image analysis where I was pretty good. I spent 15 years at it. I spent 25 years on sequences. Maybe if I had had another 10 years. But I'm back to genomics now, actually. So, I went to HHMI, I learned how to do that, but like I said, I personally just felt like I didn't have enough control of the agenda.
Around 2011, I got an opportunity to be a Max Planck director. I had been going to Dresden, Germany, for many years. I had actually found Dresden in 2002, after the Human Genome Project, when I was looking for the new, new thing, I was kind of going around looking to see what people were doing, and I was told that they were doing very interesting things in Dresden with microscopy and cell—I went specifically to that Max Planck because I really liked the way they were approaching molecular biology. Because most of the mantra is that it's molecules that are interacting with each other, so the view is kind of biochemical. That it's about molecules coming together and affecting some small reaction, or one molecule inhibits another, or activates another, or this kind of stuff. But the thing that's critically missing is that to really understand how a cell works, it's not just biochemistry; it's biochemistry occurring in a physical context. For example, a huge number of experiments, they're done in water, but that's completely unrealistic, because a cell is like molasses. It's like a hundred times more viscous than water, which completely changes the reaction rates of things. The reaction of rate of things is not the same. Diffusion is not the same, how fast things move. It's not just that.
It's also the fact that the cell is a collection of compartments, and those compartments are filled with molecules. So the bigger guys aren't really subject to diffusion; they're much more constrained. There's also pressure. There's changes in temperature. There's annealing and unannealing. For example, when cells divide, the entire nuclear envelope dissolves—just ptoo!—goes away. The whole membrane, everything that's studded in it, the pores, they all just kind of disappear. The chromosomes duplicate, they go to the two opposing spindles, the cell starts to cleave in the middle into two cells, and the nuclear envelopes recrystallize. If you think about it, that's a phase transition. It's literally melted and then reannealed. That's a physical process. That has nothing to do with molecules liking each other or not, or interacting biochemically.
That is entirely with a state transition. So there's that. There's pressure. There's movement. For example, when a sperm enters the egg, that actually determines the polarity, determines where the tail is going to be and where the head is going to be. The reason that happens is because the sperm sets up a ripple that creates a disequilibrium that allows two molecules that were in equilibrium to separate from each other. As they separate, the Par-3 goes to the front, and Par-5 stays in the back. It's happening because it's a ripple in the actomyosin cortex, which is a collection of fibers, all around the outside of the cell. So you can see, it's not as easy as A inhibits B which activates C, which is what a lot of modern molecular biology papers are about. The interaction networks—those are called interaction networks—those are all very important. It's a prerequisite. You need to know the interaction networks. But knowing the interaction networks doesn't tell you how the thing functions, doesn't give you an explanation necessarily. In order to understand the explanation, you need to understand the cell and its physical context.
What was happening in Dresden is that they literally had incredible theoretical physicist modelers working with the molecular biologists, cell biologists there, and I wanted to join them as a computer scientist to help them accelerate their studies. Because what was limiting them was their ability to digitize their experiments. In other words, the physicists could make a model but they had to have data, they had to have a digital representation of the model, of what was happening, in order to try to fit a model to it. But they had to get that out of the images, and the physicist wasn't good at image analysis. I went there basically to work with folks there on this agenda, because I thought this was the most powerful way to do molecular biology. I still think it's an incredibly powerful way. I enjoyed my time there. I went there, and I built a few interesting microscopes, and built a lot of software to interpret things and work with people to get to results.
We were very interested in the question of what controls the size of something. We still don't understand that question very well. Why does it stop? Why does it become this big and not bigger? How do you get a given shape and form? These are fascinating questions. We continued to work on it there, and I brought some very talented physicists in. We continued to work there. In the meantime, I actually ended up getting interested in genome sequencing again, around 2013, 2014. I did Berkeley for three years, I did HHMI as a postdoc for seven years learning image analysis, and then I went, applied it, in Dresden, for 10 years as a director. Then during that 10 years, I had an opportunity to basically get back into genome sequencing.
What changed it for me is there was the third generation machines. What had happened is that we went from—the machines that I was using at Celera were the quote unquote first-generation Sanger machines. After that, there was this second generation of machines, next generation of machines, that produced prodigious amounts of data, but it was all very short. The reads were like 150 base pairs. And they're still 150 base pairs, due to the nature of the way the machines work, and I won't belabor that. But in 2012, a new wave of technology came in, for sequencing, that gave you very long reads. It was a single-molecule technology, so it was literally watching incorporation of labeled nucleotides into a growing chain, and you could get reads that were like 10,000 to 20,000 base pairs long. Previously, Sanger, it was 700; the short read machines was 150; and now suddenly it was 10,000 to 20,000. Except they were incredibly noisy. The error rate was like 15%. Everybody thought that that was really bad, because the error rate was so high, but it turned out that the error was completely random.
In fact, because it was single-molecule technology, and the errors were due to thermodynamic noise, which is basically a perfect random number generator, the errors in the reads were truly random. What this means then is that you get enough reads covering the same region, and you vote, with the deeper you can cover that region, the more accurately you can reconstruct it because there's no bias in the way that the errors are occurring, so you could potentially get a perfect sequence. As a mathematician, I saw this immediately, that it was just not bad data, it was great data, because for the first time, that the source of noise was truly random and not biased. I won't talk about how it's biased in the other ones, but it was.
I got excited about this. I was like, "Wow, and they're 20,000 base pairs long. That means that if we do a whole-genome shotgun, we don't even need to read pairs anymore, we just need reads, and we should be able to produce incredible genomes." In fact, we have. So I got back into it in about 2014, after I had been in Dresden for a couple years, to the point where now I'm—and then I joined—I talked a few other people into the idea that we could go out and sequence some other things. Now, the thing that I'm currently involved in is these projects to basically go out there and sequence everything, because we can now basically almost perfectly reconstruct a genome. I was talking about back in 2000 we got 90%, 95% of it; I mean, we're talking about going from telomere to telomere [now], all the way across the molecule, reconstructing the centrosome, everything. I won't belabor the story.
There were some steps where this technology has slowly gotten better over the last 10 years, but we're now at the point where, out of a box, if I get enough DNA out of a creature, I can basically almost perfectly reconstruct its genome. So there's a lot of interest now in what's called biodiversity genomics, which is to go out there and sequence everything. The other thing that's not being talked about is that suddenly, with these incredible genomes that are highly accurate and highly complete, this idea of comparing genomes becomes incredibly powerful. It wasn't powerful back in 2005 because the genomes just weren't good enough. There were too many caveats in the conclusions that you could make. But now, you've got nearly perfect genomes.
So, we're looking at things like—I've been sequencing bats for the last four or five years, before I left Dresden. We sequenced 42 bats, new species of bats. There are about 1,400 species of bats. Bats are incredible. There are bats that live 50 times longer than they're supposed to as a function of their body weight. There are bats that eat sugar all day long and don't get diabetes. There are bats that carry Ebola, SARS, and every nasty thing you can think of, but they don't get sick. In addition, bats fly, they sing, they do a lot of interesting things. In particular, with the questions of infection and disease resistance and longevity, I think bats are incredible. We have been sequencing bats that live exceedingly long, and bats that don't live so long, bats that never get sick, bats that get sick, and we're basically now just collecting that genomic information. Hopefully my colleagues now will be able to take these genomes and begin to divine things about what's happening in bats that gives them such great immune protections. We're [already] discovering interesting things. It turns out that their secondary immune system is actually not that strong, not as strong as ours, but their primary immune system seems to have found a way to basically tolerate a certain level of viral load. It seems like what has happened is they actually just get infected, but the infection doesn't do anything to them. Interesting, right? Kind of like they're symbiotes with the virus.
We'll see where it all goes, but I've gotten very interested in the technology, and so I'm back to—and I decided to retire—I'm just going to be 70 at the end of this year, and you could say that's a bit young. I don't really want to stop, but I was—I've had periods of my life where I have had very small operations, and I've been just kind of thinking and being an intellectual, and coming up with ideas and methods. Then I've had periods where I've had very big teams. At Celera, I had 40 people, PhDs. At the Max Planck, I was up to 20. But then there have been other times where it's me and a student or two. I go kind of big and small. I basically get big, and then I get frustrated, basically having to raise all the money and deal with all the people issues, so I kind of go, "Ah, screw it," and I just pare the whole thing down, and get back to basics.
Then I do it again for [laughs] some reason. You need a big team to actually execute on something, so that makes sense. I needed a big team to produce the image analysis software that I wanted to produce. I needed a big team at Celera, to write all the code that we needed. But for me—I don't know if I told you this the last time, but I live for the eureka moments. Those moments are like an addiction. There's nothing greater in my mind, in my world, than seeing a new pattern, or a new structure. That aha—there's nothing more satisfying, more elevating, than that moment. It's not always at the level where you want to run down the street naked, yelling "Eureka!" but it occurs at a variety of levels. You can have little ones. You can have big ones. It's that aspect of this career, this life, this avocation, that calls to me, and that is the essence of what has been driving me the whole career. It has been the achievement of making something out of cool ideas. Which is maybe the essence of Caltech, too. Engineering.
Dresden and the Lure of Max Planck
ZIERLER: Did you go to Dresden knowing that this was an opportunity, a way back into genomics, or that happened sort of as a surprise?
MYERS: Nope, serendipity. Serendipity.
ZIERLER: Culturally, what was it like living in Dresden?
MYERS: Oh, it was wonderful. It's the former GDR. It's East Germany, so it's a little different than the West. People were quite friendly, I found, for the most part. It was very hard learning German. It was culturally more different than I thought. I thought it would be easy. German culture is quite different, but I liked it a lot. I made a lot of good friends there. Germans take friendship very seriously [laughs]. I don't know how to say it. In America, we're more casual—"Hey, we'll see you"—but there, if somebody is your friend, they're really your friend. You can count on them, and they show up, and they make a point of showing up, and there's a certain depth to it that I really liked. It may be though that my experience wasn't that I was kind of the normal German society; I was in a very international institute with Italians, and British, and Finns, along with the Germans. It was a very international institute. We had kids from all over Europe and the world at the Institute.
In terms of the society, Dresden is about a half a million people, but about 50,000 of them are imports. What's very interesting is that there were some of us that came in internationally, but there were also a lot of West Germans that were looking for opportunity and more freedom than in the west, that came to the east to basically start things. So I've become friends with lawyers and other people. What I realized is a lot of my German friends are actually westies, western people that have come that are kind of very entrepreneurial. It's like we're like a little enclave. It reminds me of when I was a kid, living in an expat community. It almost felt like that, except the fellow expats are west Germans, if that makes any sense. But, very interesting, very lively.
It's a wonderful place because it's a small city. It has got a river flowing through it. This is not very historical, but anyway, it's an advertisement for Dresden—it has the Elbe river flowing through it, and it's one of the only cities in Germany where they didn't build right up to the bank; they actually left the flood plain about a half mile on each side. So you have this beautiful flood plain going right through the middle of town, and then old bridges. There's a lot of old stone in Dresden. Dresden was bombed severely. There was the firebombing at the end of the War, where they destroyed 45% of the city. That sounds awful, but you have to understand that they only got bombed once. Stuttgart, for example, got bombed a 100 times, so there was nothing of Stuttgart left. But Dresden, 55% of Dresden, of the old city, is there. So there's this beautiful old stone center. Because it was the site of the Kings Augustus, the Polish kings. Actually that was their capital, and so there's a beautiful summer palace, and churches. Some very beautiful old stone. A world-class symphony. The amazing thing is like, you can live kind of in a house, right along the river, ten minutes from the center of town, and you can go walk with your dogs or your loved ones out on the flood plain—spazierengehen, as they say in German—but you can get in your car, and in 10 minutes, you can be at a world-class opera. It has museums. This was also—their beautiful jewels, the Grünes Gewölbe—there was a serious theft that occurred while I was there [laughs],. So I liked it a lot.
It was good things and bad things. There's no perfect place. They're very procedural. There's a lot of paper. It's hard to get things done. They have a bazillion rules. But then, it's a very orderly society. Everything is orderly. Most things work. There's great civil transport, public transport. Things operate. During COVID, nobody lost their job. Socialist paradise. Nobody lost their job. Think about that. I mean, it appeared America was devastated by COVID. it's also got great medicine and universal healthcare. It's a very organized society. It's clean. Streets are clean, beautiful. Beautiful, organized. It's completely safe. Your kids can take the Straßenbahn to school. They do, in fact. Nobody takes their kids to school or anywhere; the kids get on the Straßenbahn and go where they go. Because there's nothing to worry about. Think about that. Pluses and minuses.
On the other hand, like I said, very procedural. Anytime you've got to register, do some paperwork, it's a nightmare. But, it was great. I loved it. We still have a foothold there, actually. We still have a place there. We've got a lot of friends there. It's a beautiful place. I went there because of the Institute, because I really believe in the science, but I also went there because it's one of those special places that I—at least to me, it was a special place, and I feel a certain heart connection to the place. Anyway, but we're back. We're just in transition, actually. We just moved to Austin, Texas. I like it here, too. I actually think I haven't been to a place that I didn't like. Probably not [laughs] much of a connoisseur of places, because I like them all. Every place has something.
Back to Being a Postdoc
ZIERLER: Moving our conversation right up to today, because you want to remain active, what institution will you be stuck to, to do the research?
MYERS: I'm trying to figure that out. To be honest, I think I pulled out a little bit too much. I was basically burned out from too much administrative overload. I put together a center there. I didn't talk about any of that, but I built a building, and had to do all that stuff, and then I created the organization for the building, and I did a tremendous amount of administrative work. I can do that stuff, and I kind of find it tactically interesting to put all that stuff together, but basically I'm an introvert, and that stuff just sucks the lifeblood out of me over a period of time. Doesn't mean I don't love people. Doesn't mean that I can't be social or have no social skills. [laughs] Although sometimes they fail me! But I was just burned out. I think it's a function of age. I'm in my sixties, and while at Celera I could work all day long, and go to sleep, and get up and do it again, when you get over 60 you're not quite as robust. I just got kind of burnt out. I got to the point where I noticed it was getting hard to get things done. So I looked at that. And to be perfectly honest, I had a little incident where I ended up in the hospital. They don't know what was wrong with me, but it was not good. I was under three days of observation. They think it was a temporary ischemia, but it kind of made me realize that—in today's society we think we're going to live forever. I'm hoping I make it to 90. But what I realized laying in that hospital bed is I may not. I don't know what my number is. And I am getting close enough to that number, whatever it is, at 70, that it was like, is this really how I ought to be spending my time?
Just I made the decision that what I want to do is—I've given enough, I think. Maybe that's selfish, but I feel like I've given enough in terms of building organizations and mentoring people and taking care of things, and raising money and all that stuff, that what I would like to do for whatever time is left for me is I would like to do the part of this that I love, which is the discovery, the eureka, that I talked about. So, I'm trying to figure out how to do that. I'm actually currently looking at a couple places that I might call home. I have some affiliations—I'm an honorary professor at the Sanger Center in England, and I work with those guys. I'm an adjunct faculty at the Okinawa Institute of Science and Technology. I'm an Emeritus director at my Institute in Dresden. So I haven't like left things. But I have basically no people. Basically I'm an individual and I'm doing my own work. I feel like somehow or another, that's right, that that's what I want to do with the time that's left for me. It limits my impact because it's just the code that I can write and the papers that I can write myself. But that's what I want to do. I want to write some of my own papers, and produce a few programs and some ideas that I've had, and finish up some things, maybe give some master lectures. I am discovering that I actually like teaching. I've been asked to teach a few times, for a few hours at a time, and—not so bad, actually! I think in limited doses, teaching, giving lectures, is fun. So, I'm trying to figure that out. I'm trying to figure out exactly what I want to do with the next thing. I'll take suggestions! [laughs]
ZIERLER: When you land as a postdoc—it sounds like you want to be a postdoc again—what would you want to focus on? What might that next area of inquiry be for you?
MYERS: I'm already working on it. I'm back to genomics and I'm looking at comparative genomics. I'm literally thinking about how do I compare genomes, how do I analyze the differences between them. In the last year since I've been on my own, I've written an exceedingly fast program to compare genomes. It's on the order of 30 to 50 times faster than anything else out there. And I realize that that's what's needed. Some colleagues of mine tried to basically compare 600 genomes, and it took them 1.9 million CPU hours. That's not scalable, because we're going to have tens of thousands of genomes. I want to work on the algorithms and software for comparing genomes and analyzing genomes. Very focused on that. There's a lot of problems. That's what I've been working on. I hope to have a couple of papers out soon.
ZIERLER: Now that we've worked right up to the present, for the last part of our talk, a few retrospective questions about your career, then we'll end looking to the future. As you survey the field of genomics, and your contributions, both in the fundamental science perspective, and the translational side, what do we know about nature as a result of genomics, and how have we translated that to biotechnology and medicine and therapies?
MYERS: Good question. I apologize, because I haven't thought about it deeply, to be honest. [pause] I think we're beginning to learn a lot about the—I think we're going to learn a lot about how genomes evolve, how they change over time, how they adapt. We're already beginning to see things, and I think we'll see more of those. Like I said, I think just having the genomes has greatly accelerated biomedical research simply because we can intervene and modify and instrument anything within the genome that we want. I think that that has been very powerful, and there's thousands of examples [laughs] of individual discoveries of that kind. I wouldn't know how to cast them in a single characterization, but I think that genomics has contributed in that way.
I think that as we go forward, we're going to learn a lot more, like I said, about how genomes evolve, and adapt, and also about how nature adapted the genome. What's really amazing is that all levels of life, we all have similar machinery, yet look at the range of forms and functions. A lot of it is scale, and just specialization and dosage. But the elements of the genome—the nature of the proteins that you have—every creature seems to have roughly an equivalent set. What's different is the number of each kind of gene they have, and then the functional role. What we're seeing is that the same molecule can end up having different functional roles in different creatures at different stages in the development of the creature. So there's this incredible flexibility that is going on. I think we're going to continue to understand that more and more and more deeply.
In terms of translation, I think the translation is still going to be hit and miss for a long time yet to come, in the sense that what will happen is, it'll be like penicillin. We'll find something that does something. I think our ability to do like—I call it the Star Trek medicine model, where you get on your tricorder, and it computes for a while, then it comes up with a thing—we're a long ways away from that. Because we just don't understand enough about how life functions at the cellular level to intervene and understand exactly what the impact of the intervention is going to have. What's going to happen is we're going to continue to make mud pies. We're going to intervene, and sometimes it's going to be good, and sometimes it's going to be bad. We're going to have to do clinical trials.
I think we'll get better at it. It will be like forecasting the weather—we'll do better and better and better, but it will be a long time before we can forecast the weather accurately. I do think that biomedicine is going to be vastly accelerated because the number of leads and the number of clues that we have about things that might be important and what they do is increasing all the time, because of the fact that we have these genomes and that we're able to intervene, but I think it's going to be a long time before medicine becomes completely predictive. A long time. In the meantime, I think incredible things are going to happen. I think we'll be able to figure out ways to extend people's lives without necessarily understanding the mechanism in detail. But I think that's coming along. The real change that needs to happen is medicine is we need to stop putting band-aids on things, and we need to start figuring out how to fix things, molecularly. I think we may be getting near that, where we can actually do interventions that improve a situation. Like right now, I take something to prevent blood fats from accumulating in my arteries. Well, how about something that I take that basically gets rid of all of the blood fat that's lining my arteries, okay? That would be [laughs] much better, and I would love that. And I think we're going to get there. And I think it will just be—my experience is—a continuum. My experience is that things move faster than you think. Usually when I try to put a timeframe on something, I'm wrong [too conservative].
Nothing Beats the Learning Opportunities in Academia
ZIERLER: You've worked in universities, you've worked at non-profit research institutes, you've worked in industry. What does each do best, and what are the shortcomings of each, to get to those eureka moments?
MYERS: The academy is still the best place for unfettered learning, undirected research, basically people working on whatever the hell they're interested in. Some of it is pretty arcane [laughs] and of questionable utility but, doesn't matter! That's the role of the university, of the academy. What were the other choices?
ZIERLER: Non-profit research institute, and industry.
MYERS: What's great about companies is that they are under pressure to produce, financially. That tends to have them be more focused, and mission oriented, and they push harder. Timeframes are shorter. Things move better. When there's a specific mission, and a halfway decent plan for how to get there, a company is a good way to go, if you want to get it done fast. Companies are good at execution and manipulation. When you want to execute, when you've got the plan, you've got the idea, and you want to go—company.
Not for profits—I think that the most interesting thing about not-for-profits is that they can experiment with models of intellectual cooperation or models for research. They can try funding things in different ways. They can create different incentive systems, and experiment with how to get people to create and work interdisciplinarily. Interdisciplinarity is a very difficult thing, I think for several reasons. One is that people are typically trained narrowly, when they get a PhD, and they're not prepared to think about that. I think that was one of the values of Caltech, because I think that PhDs should be pretty narrowly focused, but an undergraduate technical education should be broad, and I thought Caltech was very broad. So the fact that I know a little physics, I know a little chemistry, I know a little bit of this and that, has actually given me a kind of perspective that has allowed me to acquire the things. But many people are quite narrowly interested in this. I think that's one thing that a not-for-profit can do, is create a system—because academics tends to get very narrow. You get in a chemistry department, and people are going to evaluate you as a chemist.
But if you're doing this weird thing and it's kind of semimolecular and stuff, then they're not quite sure how to evaluate it. What I find is that universities tend to create siloed funnels. They tend to create siloes. I think that not-for-profits can break the siloes. That should be the role of not-for-profits is to create truly interdisciplinary—I mean, it's hard to figure out how to do interdisciplinarity. I think the correct formula is that when you have several people with different skill sets come together, there's always the question of who's going to be the lead. You've got a molecular biologist, a computer scientist; who's the lead? Well, the way I like to think about it is if you've got the three people and each are known entities in their fields, then it doesn't matter who the lead author is, because you just put all three authors on the paper, and everybody knows who did the physics, everybody knows who did the computer science, and everybody knows who did the molecular biology. You don't need to worry about it. But a lot of people do, and they still haven't figured that out.
I don't know if it's just because of my own experience, but my belief system is that the work is more interesting and the results are more interesting the more different kinds of minds you apply to the problem, and that's good. I'm not so worried about what I'm going to get credit for. What I want is I want the absolute best and most compelling result. Sometimes you just want the result, period, which can't be obtained any other way than having all three skill sets working together. It has been frustrating for me, kind of, as a computational molecular biologist, an interdisciplinary person, to basically watch what's happening. Academics doesn't tend to do that very well. Not for profits could potentially do it very well. And then companies are the place to execute. And there it works no matter what because the goal is to get to the product.
ZIERLER: Gene, returning to your Caltech education, being honored as a Distinguished Alumnus, a moment of reflection for you, what does that external validation mean, as you reflect on your career?
MYERS: I've struggled with a sense of self-worth, I think, throughout my career, in terms of my ability to do science. In fact, I only feel as good as my last result, sometimes, if you know what I mean. So, just to be honored by the place where I started, probably where I was the least confident and probably the most overwhelmed, it's just—it's warming, actually. It makes me feel like maybe I was worthy of the place. And that I succeeded, at some level.
ZIERLER: Finally, Gene, last question, looking to the future. In your capacity as a mentor, to postdocs, graduate students, you've led such a long and varied career. What are the most exciting initiatives in bioinformatics, in genomics, in microscopy? If you would be able to give advice to up and coming scientists, where would you expect them to dedicate their efforts?
MYERS: I'm very bad at that, because I'm only excited about what I'm excited about. [laughs] So, sorry. I look around, and I get excited about things, and I usually pick one thing. Right now I think the most exciting thing is this biodiversity genomics. I think down the road, anybody that does sequence based work, this is the way we're going to do it. It's not for my generation but the next generation. What are you going to do when you have the genomic sequence of every extant creature of the planet, and you can do experiments on any creature you want? It's going to be a whole new ballgame. I think that's incredibly fascinating. Just getting out of my own zone a little bit, I think that quantum computing is starting to look very exciting. We've got enough q-bits on the planet at this point that you can do some pretty interesting things. And to me, it's rather fascinating. It seemed like science fiction that we were going to be able to do quantum computing, but, damn, I mean, they're expensive, but we've got q-bits. We've got, I don't know, 30, 40, 50 q-bits on the planet, maybe more. Forgive me; the physicists will forgive me if I'm way off. You can program them. I think it's gonna be pretty damn interesting. I think that's going to be incredible. Because basically, crypto completely changes when you do that. For people that are into that kind of stuff, that's going to be incredible.
AI is going to be obviously another fascinating thing. I'm just amazed at how a completion system like ChatGPT does so well at mimicking human performance, given a sufficiently large corpus of data. So it looks like some of these things, in terms of AI, are really just questions of scale. It's like, have enough data, have enough computing nodes, something is going to emerge. It's kind of like human intelligence. The difference between us and a chimpanzee is a factor of 10, in terms of number of neurons. The basic structure is exactly the same, but—so that factor of 10 has allowed a qualitative shift in the level of cognitive performance and mental ability. I think the same thing is true [if AU[. When we have AIs that have the equivalent of 100 billion neurons; it should be pretty interesting. That's all computer stuff. Superconductivity I think is hot. Energy generation. Another fascinating set of problems. I wish we would develop thorium reactors, honestly.
I'm not quite sure why we aren't going that way. They're clean. They don't explode. Pretty good! Yeah, I don't know—it's interesting—actually I was looking at the agenda for the alumni week, and the kids are going to be giving talks. It's the part I'm planning to attend, actually! [laughs] I want to see what the kids are up to! I think materials science is really incredible, the materials and the composites that we can make. If I could start over again, I'd be interested in surgery. I'd be interested in medicine. Surgical procedures. Our ability to build artificial materials that aren't rejected by our bodies is incredible. Yeah! I would probably really be interested in trying to understand human health, I think, as a fantastic problem. To understand ourselves molecularly would be pretty cool.
ZIERLER: There's no shortage, is what you're saying.
MYERS: Yeah. My fantasy is that I could live a thousand years and have ten careers.
ZIERLER: [laughs]
MYERS: It's really too bad, you know. But I don't feel like I have enough time to start over again. But if I had time, I'd be an architect, I'd be a surgeon, I'd probably try a hundred years of music, and athletics. It'd be fun!
ZIERLER: Maybe it happens and we just don't know it.
MYERS: Yeah. [laughs] Maybe!
ZIERLER: On that note, it has been a great pleasure spending this time with you. It has been a terrific pair of conversations. I want to thank you so much for doing this.
[END]
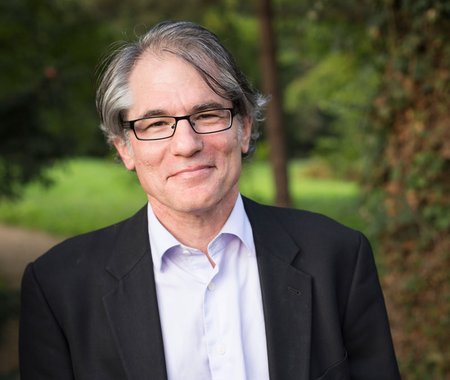
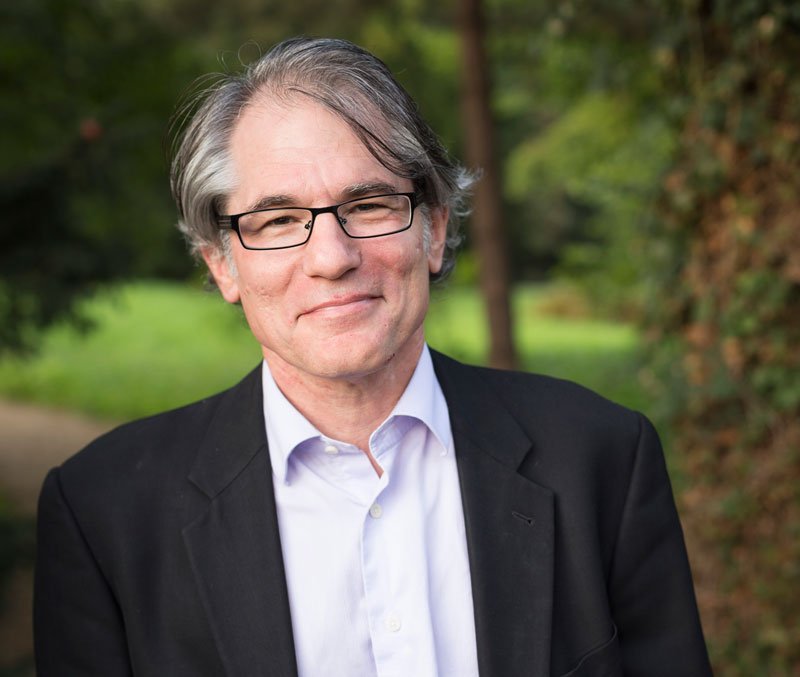
Interview Highlights
- Going Emeritus and New Research Opportunities
- Tracing the Computational Approach to Biology
- Longstanding Emphasis on Fundamental Research
- The Caltech Crucible
- Thesis Research in Computational Language Analysis
- Algorithms and Biology at the University of Arizona
- Celera and the Origins of Genome Mapping
- Genetics as a Massive Computational Challenge
- Competing With Government Agencies
- Genomics Success and Return to Academia
- The Foundation for Medical Advances
- Microscopy and Janelia Farms
- Dresden and the Lure of Max Planck
- Back to Being a Postdoc
- Nothing Beats the Learning Opportunities in Academia