Kanianthra M. (Mani) Chandy, Computer Scientist
October 12, 14, 18, and November 11, 2021
As he remembers it, Mani Chandy's graduate research in electrical engineering was really a function of the fact that his interests pre-existed computer science as a discrete academic discipline. Over the last fifty years, his career has matured alongside and propelled the field. Chandy is one of the world-leading experts on distributed computing, whereby computers located on different networks can communicate and coordinate their activities.
Born in Kerala and educated at the Indian Institute of Technology, Chandy came to the United States to pursue graduate research at NYU and then at MIT, where he developed very large optimization programs. His work at Honeywell provided real-world appreciation of the industrial needs of large-scale computer systems, and he taught in the first half of his career at UT Austin at a time when Austin was becoming a major technology hub.
Chandy joined Caltech's faculty in 1989 and was named the Simon Ramo Professor in 1997. He has served as Executive Officer of Computer Science and Deputy Chair of EAS. Chandy's research has been recognized by some of the most prestigious awards in the field, including the Koji Kobayashi Award for Computers, and the Communication and Charles Babbage Award from the IEEE. In 1995 Chandy was elected to the National Academy of Engineering, and in 2019 he was named a Fellow of the Association for Computing Machinery "for contributions to queueing networks, performance analysis, distributed and parallel programming, and distributed simulation."
Interview Transcript
DAVID ZIERLER: This is David Zierler, Director of the Caltech Heritage Project. It is Tuesday, October 12th, 2021. It is my great pleasure to be here with Professor Kanianthra M. Chandy. Mani, it's great to see you. Thank you so much for joining me.
KANIANTHRA M. (MANI) CHANDY: My pleasure.
ZIERLER: First things first, you're known universally as Mani. How far back does that nickname go? Did your family call you that, or that's more of a recent development later in life?
CHANDY: That's my name. That's the name I was baptized with. In the state of Kerala in India where I'm from, there's a large so-called Syrian Christian population. That's people who were converted to Christianity by Saint Thomas. Mani is a diminutive for Emanual, so that's where it comes from.
ZIERLER: Your first name, Kanianthra, what does that mean, and why is that not the name by which you go?
CHANDY: Kanianthra is actually the family name. We had a different nomenclature algorithm, and when the Brits came, the algorithm got Anglicized. Kanianthra is really the family name, and its literal meaning—"Kanian" is an astrologer, or possibly an astronomer. Probably astrologer is what it meant. "Thra" is house. So way, way back, I presume we got into an astrologer's house and that's our name.
ZIERLER: The only one remaining is Chandy. Where does that fit in?
CHANDY: It's an interesting story. Chandy in Kerala, also I think parts of Orthodox Christianity, is a short form for Alechander [pr] which is, again, Alexander. The literal translation into English would be "Astronomer's House Emanuel Alexander."
ZIERLER: A whole story, right there in a name.
CHANDY: A whole story right there, yes.
ZIERLER: Mani, on a more official level, what is your current title and institutional affiliation?
CHANDY: My title is Simon Ramo Emeritus Professor at Caltech. I've been that since 2014 when I retired.
ZIERLER: What were the circumstances of your retirement in 2014?
CHANDY: I got to the age of 70, and I think it's a good idea to retire—not to stop work, but to retire officially from Caltech. From my point of view, it's good to open up positions for younger people. You can keep working at Caltech and keep teaching. The institution derives from your work, from your history, but at the same time opens up slots for young people, which I think is very, very, very important.
ZIERLER: Mani, when you went Emeritus in 2014, in what way did not having as much administrative responsibility or some of the other responsibilities associated with being full-time faculty possibly expand your bandwidth to focus on the research that was most important to you?
CHANDY: First of all, it creates a lot of time. A professor's time is divided between teaching, research, and administration, all of which are important. But it's nice not to have the administrative burden, including things like attending committees, all of which are very important, and I'm not belittling those activities, but it's nice in the emeritus position to be able to pick and choose which committees you want to work on. I think that helps a lot.
Secondly, I think you can take a broader view, a longer term view, of teaching as well as research, because you aren't involved in day-to-day teaching. You can think more generally about what teaching is about in a more philosophical point of view, and likewise think longer-term about the research. You don't have to get something done right away.
In my case, there was another very practical issue, which is that as a professor, I rarely had time to code, to actually write programs, because I was so busy helping other people. As a computer scientist, I think it's important to actually get your hands—I was going to say "hands dirty"; of course nothing gets dirty—but actually to build things, build fairly large things and see how they work, and see where they maybe don't work, which takes time. That's not time typically available to most professors.
ZIERLER: You mentioned committees that were important to you since you had the opportunity to choose. In the administrative realm, what kind of service work has been important to you since you've retired?
CHANDY: I pay a lot of attention to what's going on in teaching. It's not so much attending committees as listening to what the committees say, and listening to what students and faculty say. It's more a question of hearing than talking. It's a tricky issue at Caltech because the requirement for students is very broad in science. They've got to take physics, chemistry, biology, all of which are very important. But increasingly as students go into industry in computer science—it's a shift over time; this isn't the way it used to be. Everybody would go to graduate school, but now I think the majority go into industry, and many of them start their own companies.
So there's a different kind of pressure now, in addition to understanding the fundamentals and be really good at it, to know what it takes to get something going now, today, with the latest technologies. There's this pressure, which I think Caltech is handling well—the fundamentals of what's going to support somebody over the long term, a 20-year period or 30-year period. This is what's going to make them a provider to society, people who come up with new ideas. However for the short term, like the next year, they need a collection of tools of how to deal with this issue, and it has become more of a question as more people go into industry, as more people go to startups. You go to a startup or go to a potential donor of funds, you've got to give a so-called elevator pitch, so you've got to know something about what's going on technically as well. I think again the department and the university do a good job of dealing with this tension.
ZIERLER: Mani, more recently, were you involved in discussions as Caltech, like so many institutes of higher learning, had to pivot to a remote teaching platform during the COVID-19 pandemic?
CHANDY: I wasn't involved with discussions. However, I have changed completely the way that I teach. I teach a course every second year. It's a course oriented at juniors and some seniors, but also some graduate students. One question is, for Caltech, if a student cannot come to class because they've got COVID or some symptoms or whatever, what's the best way of reaching them? More generally, if we come up with a methodology that helps Caltech students, then can we use the same methodology for anybody anywhere in the world? Could material we are preparing here apply to somebody in India or Africa, who has access to the internet but not much else? This is a larger question for education across academia. MIT and Harvard are both looking at this issue. What can we at research institutions, privileged institutions, do to help the universal community of learners, especially as people continue learning? It's not just finishing a course and being done with it. But to the extent that we want to keep learning, every five years come back to it, so what can we do? What can we do? What should we do? It's a big question, and many people at Caltech are studying it. I'm trying to do my own little part with that.
ZIERLER: Mani, on the question of the pandemic, for your own research and the mandates of social isolation and essentially working on your own, physically at least, has the past year and a half been more productive for you as a result?
CHANDY: I don't know that it's more productive. I think that it's helpful to go in to the Institute and meet people and just talk. It has saved time, in the sense of not having to drive back and forth. It's a two-edged sword. I think there's benefit from personal interaction. It's easier to go downstairs to a seminar, I think, and to listen, than to remember to dial into a Zoom. There's a different quality of interaction person to person. There's a tradeoff, and I think the tradeoff could be best managed by eventually having certain days on which everybody shows up, and certain days in which people can stay at home and work. It could be two and a half days at work on campus, and two and a half days working on your own, wherever.
ZIERLER: Just as a snapshot in time, circa October 2021, what are you working on currently?
CHANDY: A couple of things. Getting back to teaching, I'm spending a lot of time on my course. Let me talk about that first, and I'll go on to other research issues. One idea, which is an experiment—we don't know if it's going to work—is to come up with a website that's ongoing, free of charge and all that, for teaching, [on] distributed algorithms. These are algorithms dealing with multiple agents collaborating to achieve some goal. There are many such algorithms, and many of them are very, very tricky. But one of them, for example, is the one underlying cryptocurrency, bitcoin for example. There's lots of interest in these algorithms. So suppose a Caltech student two years from now… "Gosh I remember—I wonder what happened with the bitcoin algorithm." Could they come back to this website and in 10 or 15 minutes, access the information that they wanted?
That requires overcoming a couple of challenges. One is that you don't want a very long description, however you want enough that they can pick up where they left off and understand what's going on. So I'm trying to make these very short videos, typically about four minutes for each algorithm, and a short module for each algorithm, to essentially curate the algorithms into—what shall I call it?—a compendium that Caltech students and possibly others can use. This is proving to be quite a challenge because you've got to find the essence of each algorithm, make it into a four-minute video, then have self-tests so students can test themselves and so on. So that's taking time. It's a challenge. But it could, over some period of time, be a vehicle for learning. Not just for a course, but for learning in general. That's one thing I'm doing.
The other thing that I did, and I stopped doing now, is I worked with the geologists at Caltech—you may know about this. The Community Seismic Network is something that Caltech put out, where we have a very cheap sensor, about that big, which we have been deploying across the Los Angeles basin. The computer science part of it is that there's a research component and an ongoing implementation/administration component. The research component was to get the algorithms by which all these thousands of sensors would send data into the cloud. I'll get into a little bit of detail and then I'll back off. This includes looking at how a building shakes. To understand how two adjacent floors move to understand the tensions between them, you have to get the timing very accurately on the two sensors on each floor, because if the timing were off, and when they went like this, we think this went at one time, and this lower one went at a different time. There are algorithms to ensure that the timing of these sensors is very, very accurate.
All of that research, which was essentially doing new things, we finished fairly early on. And it succeeded, so we have this system going on for a long time. Now it has transitioned to a different kind of problem, because now you have an instrument. The instrument works. The instrument looks at ground shaking. And now it's the geologists and civil engineers using that instrument for their purposes, understanding the L.A. Basin, understanding buildings. At this point, the computer science part, which was more of a hand maiden in creating the instrument—plays less of a role.
This is a success story because it has been going on for more than ten years. Initially it was funded by the National Science Foundation because there was a research component, but now the tricky thing has been transitioning into administration of an ongoing instrument. It still requires funding, because if you have thousands of sensors in all these buildings, somebody has got to go look at these sensors when they fail, when batteries run out. It's a wonderful thing, at least so far, that the Community Seismic Network has continued to get just adequate funding to keep that instrument going. That's one aspect of the research. The other aspect more generally is coming up with mechanisms for understanding and reasoning [for] multi-agent systems. So you've got all these agents, you've got these sensors—sensors in cars, for example—different kinds of sensors in cryptocurrency, but they're all working—multiple of them are working together to somehow achieve a goal. Quite often these algorithms seem correct but are wrong. When they are wrong, it's very hard to catch, to detect, very hard to test, because there are so many agents you can't guarantee that they will behave exactly the same way each time. The other part of what I'm working on is what you might call correctness, proving that these multi-agent systems work as they are designed to work. That's where the research has been.
ZIERLER: Who are some of the new institutional supporters of this research, both in the government and in private industry?
CHANDY: There's a lot of support. Again, let me break this up into two parts. It's much easier to get support for research as opposed to getting support for maintaining a complex instrument such as the Community Seismic Network. For the research part, the support are all big companies, because whether it's social media or Google or Amazon or IBM, they're all working on fundamentally distributed systems, so-called concurrent systems, many things happening at the same time. So there is support from them. Sometimes the support is tied to their products, coming up with a research plan but with incentive to use their underlying infrastructure, which is not necessarily bad. I don't think of that as a bad thing, provided you can do the basic research. So I think there's support for the research part from NSF, ARPA, and most recently now ARPA-E. That's the energy part. I wouldn't say that's taken care of, but people can, I think, get funding for research.
There's a trickier question about funding for instruments which are producing research. Because you can't go to NSF and say, "Here, I need money not to do research but to maintain this instrument." That's where Caltech has been helpful, and donors to Caltech have been helpful. Me personally, I don't need research funding anymore, because I don't have students. The equipment that I need to keep going is very inexpensive, and I've got enough funding to buy the sensors that I need or the computers that I need.
ZIERLER: In both aspects of this research, who are the key collaborators you're working with, both within Caltech and beyond?
CHANDY: In basic research, most of my collaborators are outside. I have people from the University of Texas where I used to work, people at Microsoft. It's a fairly large collection of people that I've written papers with and continue to interact with. In Caltech—and again, this might be worth emphasizing—you don't see the sort of research happening that much at a lot of universities where computer scientists would work with a geologist and a civil engineer like that. There's Tom Heaton, who is in Civil Engineering, and Rob Clayton who is in Geology, and Monica Kohler, who is both a research professor of Geology and Civil Engineering, and a bunch of other people who I work with. Initially, we had the research phase of the three of us: computer scientist, civil engineer, and geologist. That's where we got NSF funding. Now that it has gone on to the implementation phase, I think it's run primarily—we're also getting older, so people are retiring. I think both Tom and Rob are now emeritus or part-time. But the instrument is still functioning and we still can do some interesting research.
ZIERLER: Mani, back to your title, I'm curious if your research has any particular connection to Simon Ramo's research or if you understand the circumstances of there being a chair named in Ramo's honor.
CHANDY: Ramo is a very famous person, as you know. I happened to have the fortune to meet him, I think for his 100th birthday. He was amazing! Of course he has an amazing history. He started Thompson Ramo Wooldridge. He played a very significant role in helping the U.S. with its military strategy. They branched out into commercial purposes. He was really a marvelous engineer, and it's great to have that name, the Simon Ramo Professor. I really think it's an honor. There are many eminent people at Caltech, but Ramo surely is one of the really outstanding ones. Back to his 100th birthday, I think he had a cane, but he walked to the microphone, and he gave a terrific speech. Gosh, I'm 77. If I could do something like that [at his age…]
ZIERLER: Mani, as is well known, I think by far the most popular major for undergraduates at Caltech now is computer science. If you compare that with 40 or 50 years ago, the most important or popular major was physics. What do you think at a broad level that tells us about Caltech, about the field of computer science, and about the motivations and interests of undergraduates over the course of this transformation?
The Popularity of CS at Caltech
CHANDY: That's an interesting question, and it has again many parts to it. One part is what does it tell us about students. The other part that is implied is what does it tell us about how Caltech responded or should respond. Students are voting with their feet, and why do they do it this way? It's a difficult question, partly because there's so much in the news about computing, artificial intelligence, cryptocurrencies. It's all over the place. I think there's very exciting research going on in physics, but it probably doesn't get quite the same airtime, at least in the social media that, say, a 15-year-old would look at, as computing does. When I walk around here, I meet parents of 12-year-olds and 13-year-olds, all of whom want to do programming right now, they want to learn how to do programming in Java, Python. They take the physics for granted. You've got to learn physics though. So I think a lot of it has to do with airtime, at a very young age.
Another aspect which I've been told—I've got to think about this carefully—is that the initial difference in population of males versus females, which has changed by the way, was due in part to gaming. Boys were more likely to play online games, and they always associated the game with computing. Of course there was an aspect of computer programming with a game, and that influenced them to go into some aspect of computer science. The other perhaps—again, very, very important part—is we've had some undergraduates from Caltech who started companies and have done very, very well. There have been others who have gone to startups, not necessarily to start them up on their own, who have also done very well. So there's an excitement, this whole entrepreneurial spirit, forging ahead, just as you would in research, but in building and identifying a need and fulfilling it. There's an excitement there as well, which is probably higher in computing than in physics. Not that physicists don't make startups, too. So these are a collection of factors, a constellation of factors which I think influence this.
Now the other part of the question, which is sort of implied, is how should or how did Caltech respond. Again, this is a tricky thing, because you don't want to respond too quickly to students voting with their feet, because they may vote with their feet one direction, and then vote with their feet the other direction after a few years. These institutions have a lot of momentum, or inertia. I don't mean inertia in a bad way, but you can't respond back and forth too quickly. On the other hand, if you don't move it at all, you will just go ahead and hit an iceberg. There's again this tension between doing things just right, not too quickly but not too slowly. Part of what Caltech has done right is to think now of computing not limited to a department, but that "Caltech computes." That's one of the taglines we have—that Caltech computes. All of Caltech computes. Computer science works with the rest of Caltech, with biology and physics. This I think is an opportunity to distinguish Caltech from many other institutions, in that we're not high behind these walls and say, "Here's the Computer Science Department, and that's something else." We encourage these interactions.
Having said that, there is an issue—that for teaching, we've got a challenge. Caltech prides itself on having very good faculty-student ratio. We have 1,000 undergraduates, about 600 postdocs, 300-something faculty. The PhD to undergraduate ratio is almost 1:1. I'm teaching a class now with I think about 70 students, somewhere between 65 and 70 students. This is a junior/senior class. Ideally, if we look at Caltech averages, I should be teaching a class of ten. Again, the challenge is students have voted with their feet. You've got to meet that need and do it in the best possible Caltech way, which requires paying individual attention to individual students. The tension now is how to do that when you've got such a large class. Part of the answer, which again I think Caltech has done reasonably well, is to have so-called—are you familiar with SURFs?
ZIERLER: Yeah.
CHANDY: So to have SURFs in computing, to deal with computing, that are not limited to faculty in computer science.
ZIERLER: These are SURFs, S-U-R-F-S, not serfs in the feudal sense, of course. [laughs]
CHANDY: [laughs] No, no, not at all. Which I think is a good thing. I think the key has to be more things like SERFs, encouraging undergraduates to work with individual faculty. To the extent that computer science is overloaded, to work on computer science problems with faculty in other departments.
ZIERLER: Given that computation is so fundamentally important across the board in science and engineering, is part of the solution then for computer science professors to expose or to gently push students into exploring, for example, biological engineering, or neuroscience, from a computational science perspective?
CHANDY: I wouldn't use the word "push" or even gently nudge. I would say that we want to emphasize the applications of computing as well as the fundamentals of computing. Again, this is anecdotal; I think there was a study at Harvey Mudd on again, population of women and men in computer science, because early on, the population was largely men, at Caltech, percentages. The Harvey Mudd study, if I recall it right, showed that if faculty in the environment emphasized the consequence of the research—so in computer science, to see how computer science helps with creating and understanding molecules and DNA and going into vaccines, that that motivated women more than just saying, "Here's a really cool algorithm." I've got to be very careful with what I say here, because it's not suggesting basic differences in gender.
Getting back to your question, there's two things that are exciting about computer science. One is the basic theory, the basic algorithms, are fundamentally exciting. They're just worth studying, merely on their own, even with no consequence, just because it's interesting. On the other hand, it's also worth studying because there's immense applications. Getting back to your question, I would rephrase it as describing the consequences of what we do, rather than saying, "You should go look at neuroscience." Because I think the students decide on their own what interests them.
ZIERLER: As you well know, we are experiencing something of a quantum revolution globally, and of course Caltech is a center for that. In your research and the things you're interested in, are you connected at all to the world of quantum science, quantum computing, quantum information, from an interdisciplinary perspective?
CHANDY: No, I'm not. What I'm doing is not connected to that. On the other hand, I think Caltech again did the right thing. There's tremendous opportunity because of the relationship between information theory, computer science, and physics. I think Caltech, the institution, did the right thing by emphasizing this very early, long before it became sort of the "in" thing.
To answer that question, no, I'm not, but to follow up on it in a different direction, this has been a challenge at Caltech for the Department of Computer Science, which now has officially—I don't know how many—12, 14 people—whereas Georgia Tech might have 114. MIT has god knows how many. The ratios are orders of magnitude. A consequence, when we do research, is that the group has to somehow make bets on what's going to be important ten years from now. Sometimes these bets pay, and sometimes they don't, but we have no option but to make these bets, because we're so small. If we were as big as MIT, then what we would do is just cover the waterfront. You'd keep hiring people as the waterfront got bigger. But because we're so small and we're going to remain small, we've got to play these bets. One of the bets that is right, that's paying off, is in quantum computing.
ZIERLER: Just so I understand the binary correctly, if you are not involved in quantum computing, is that to assume that your research world is strictly one of classical computing, or are there other titles in that decision branch to call this?
CHANDY: At this point, it's exclusively classical computing, because there are a whole raft of problems that we need to overcome there first, before we get into quantum computing. If you're driving a car and you're concerned about how all the different—a car has many, many sensors and computers, and they all interact. There's so many basic problems in classical computing before we think about how quantum computing could play a role in autonomous vehicles or cryptocurrency. There will come a time when we've got to get over these first challenges.
ZIERLER: I'm sure you've heard people in the field talk about—one of the challenges with quantum computing is not only what exactly is quantum computing, but what will it be used for. Is there a connection then between all of the fundamental work that needs to happen in classical computing before we can achieve finality in those questions in quantum computing? Or are in some ways these trains of thought or these knowledge bases separate or disconnected somehow?
CHANDY: Oh, no, there's two parts to that question. These two trains of thought that have to overcome their own series of challenges, they're going to meet, and they're going to meet in the not too distant future. You can see that happening now, because you have entrepreneurs financing quantum computing, the work that Google is doing. They wouldn't be doing a lot of that work unless they saw a confluence of these two trains. The question is, when will that happen? That's a tough question to answer, but it definitely will be. Certainly almost everything that's going on in cryptography will be influenced by what's happening in quantum computing.
The Origins of Computer Science
ZIERLER: Some broadly conceived questions about nomenclature and titles. Your education, your diplomas are all in electrical engineering, but your professional life as a professor has been in computer science. What are some of the big takeaways there, both in terms of the intellectual trajectory, and the development of both electrical engineering and computer science over the course of your career?
CHANDY: When I started in 1965, there was no computer science. There was no computer science; there was no computational science. Computers were hard to come by. I got my master's at what is now NYU in—graduated in 1965, got my master's in 1966. When I began at NYU, we didn't really have a computer science department, so a lot of the computer science was done in mathematics or in electrical engineering. Then I went to MIT, where I got my PhD in 1969, but I got it in what's called operations research. What MIT had at that time—still does, actually—is you join one department, but you got your degree in the Center of Operations Research. You could be in computer science or engineering or wherever, but you got your degree somewhere else. So even though my official degree was in EE at MIT, it's in what's called operations research. My professors were all in the business school. But by then, 1969, in computing, there was a computer science department, and a lot of excitement in computer science.
Getting back to question as to what does it all say, academically there's a question of when do you start a department. It's a very difficult question. Again, you don't want to do it too soon, because otherwise you're starting departments right and left. On the other hand, if you wait too long, you miss the boat. A partial solution is to start these so-called centers. When a new idea comes up, you start a center, and the center is really successful, and then it evolves into a department at some time. I think, again, Caltech is doing a good job with that. We start a lot of centers, but we don't start departments that often.
ZIERLER: Negatively defined of course, it would make sense why your diplomas are in electrical engineering and not computer science. There were no computer science departments. As a matter of identity or intellectual heritage, do you then consider yourself an engineer who went into computer science? Or did you use electrical engineering to pursue interests that reached a level of maturity so that you could live in an academic computer science world once that became available?
CHANDY: Let me push back a bit. To the extent that research is research, the quality of the research [is not rooted in the] background. You can be a poet and do fundamental work in computer science. It's an interesting question. It's a larger philosophical question, so I'm philosophizing here. I think in some institutions, there's perhaps more of an emphasis on pedigree—the schools you went to, the professors you worked with, the departments you graduated from. I think—at least I'd like to think—that Caltech is different, in that all that matters is the research that you produce. Is it fundamental? Is it new? Does it have an impact? It doesn't really matter that much what your pedigree is.
Getting back to your specific question, I got my degree and my PhD in operations research, but I joined the Computer Science Department at the University of Texas because they were interested in using operations research for designing computers, specifically something called queuing theory, which deals with how queues are formed, and designing queues in computing systems. I joined the Computer Science Department at Texas because they were interested in operations research for computer science, and then it turned out to be a very fertile field, so I just sort of stayed on.
Getting back to the larger question, people really should be changing their fields of research quite frequently, definitely every ten years, maybe every five years, because the fields are changing so quickly. What really matters is not so much your educational pedigree as your ability to ask the right questions, to ask the bold questions, and luck, I guess. But I really would like to think that Caltech doesn't really care what your background is.
ZIERLER: I wonder if another distinction in the question of computer science and electrical engineering, is the extent to which the distinction between hardware and software is relevant as well? Of course I would overgeneralize here, but to the extent that electrical engineering is a world of hardware, of transistors and circuits and things like that, and computer science is more interested in programs and software, I wonder if those were part of the consideration for you in terms of the things that you were interested in?
CHANDY: Yes, certainly. I was interested, all along, in algorithms, which in that sense are software. But if you look at our EE Department, I don't know the fraction, but I think more than half deal with what you would call software. Less than half deal with transistors or antennae and so on. Again, I think it's really dangerous to draw a hard line between the two, dangerous in many ways. One practical way is that there's a whole area of what's called asynchronous computing, developed in large part at Caltech, not by me but by Alain Martin who has retired, where essentially you take a program and implement it with a computer, so there really is no hard line between hardware and software. Caltech has been good in emphasizing that there is no such hard line. In general [laughs]—again, waxing philosophical—hard lines between anything are dangerous. I think it's a problem for academia, in general, but I think much less of a problem for Caltech.
ZIERLER: You mentioned 1969 for the development of computer science at the departmental level. Just technologically and intellectually, what were some of the advances in the mid to late 1960s that made a department of computer science feasible where it wasn't when you were a graduate student earlier in the decade?
CHANDY: I'd say there were three things. First of all, computers became much more widely used—the Defense Department, banks. I'm talking generally, not about Caltech now. There was pressure on universities from the Defense Department and industry for people who specialized in using computers. The issue became not so much the hardware but how you actually get operating systems, how you get these computers to work to do useful things. There was external pressure from both government and industry, is one part. Then there was sort of internal, grounds-up pressure from students, because they had all these exciting problems, for example in operating systems, and you've got all these people going into all these jobs, which really are not hardware problems. So there's pressure from the top, and then sort of groundswell from students. Then I think faculty found really exciting problems, so there was this confluence of things. I think the research establishment, like ARPA and National Science Foundation, were also fairly early in identifying the importance of this class of problems and providing funds for it, which causes natural groups to form and departments to form.
ZIERLER: Very broadly conceived, over these roughly 50 years, from 1970 to now, what aspects of computer science remain the same? In other words, what are still some of the ongoing fundamental questions that are as relevant today as they were 50 years ago? And what questions are being pursued today that as a result of technological, computational advances and just different ways of approaching problems would have been inconceivable to a computer scientist in 1970?
CHANDY: That's a good question. Certainly one thing that stayed pretty much unchanged over the years is the development of algorithms. I'll give you a very specific example. There's a so-called shortest path problem. You want to find the shortest path from one point to another. One of my colleagues actually called Dijkstra came up with a solution—it's a very simple solution—I think in 1965 or 1964, but we still use it 60 years later. The question is, here's a problem, here's an algorithm that solves that problem, and here's how that the algorithm correctly solves that problem. It has remained unchanged all these years, and the fundamental theories that support this have been really more or less unchanged. New ideas have come up on top of this foundation, but the foundation has stayed unchanged. I don't know how much to get into detail.
Another fundamental problem that has been here all this time is—cut me off if I'm getting too much into the weeds—there's a class of problems called polynomial or P, and what might be another class called NP, non-polynomial. How shall I explain it? There's some problems that are fairly easy. We know they are easy, and we know how to solve them. These problems, the time to solve them goes up only polynomially with the size of the problem, the shortest path being one of them. So if you double the size of the problem, you're going to solve the problem in a polynomial of two.
But there's other problems that we don't know how to solve fast. A slight variant of the shortest path problem is the traveling salesman problem. The shortest path just asks for the shortest path between two points in a graph. The traveling salesman—I guess we should say traveling salesperson—the person has to go visit all these cities and come back to the origin in the shortest possible time, or the shortest possible distance. In one case, you're trying to find the shortest path between two points. In the other case, you're trying to find a route. The second problem is inordinately hard. We don't know how to solve it. Even though it's easy to explain, we don't know how to solve it fast. We don't know how to solve it in polynomial time. As the problems get larger and larger, the time taken to solve that problem goes up exponentially. So way back, the question is, are these two types of problems just fundamentally different, that we'll never be able to solve the shortest path problem and indeed the traveling salesman problem fast, never ever, because there's something fundamentally different about it? Or, is it just that we aren't smart enough? This is a very basic question, and it has been there forever. We still don't have the answer to it. So there have been these questions that have lasted for decades.
The other part of your question—what has changed—one thing that has changed is that computing has become so cheap that you couldn't imagine this in the 1960s. I'm thinking you couldn't even imagine in the 1960s having all the power that computers back then did. As a consequence, back then what you would consider a waste of computing power, today it's like water. It's just widely available. You can now do things in artificial intelligence just by looking at a lot of data with a lot of computing power, which would have been unimaginable in the 1960s and 1970s. So it changes the question. The question is no longer what can you do with limited computing power, but really, what can you do with almost infinite computing power? The scarce resource becomes data, not computing power. It has shifted what's scarce, what's important, and what's necessary.
You can see the importance of data, even in the discussions going on in Washington. Facebook and Google and many other companies have immense amount of data, and data is the gold these days, whereas computers are [laughs] the water that you use to produce gold. That has been a massive shift in thinking. Another example of how computers have become so cheap in its application is they talk about mining—mining for cryptocurrencies, mining for bitcoin. You have huge numbers of computers using huge amounts of electricity to mine the next bitcoin. Even if somebody had come up with a bitcoin algorithm in 1970, nobody would have thought of actually pursuing that idea, just because computers were the scarce resource. There has been a really, really massive shift from thinking about hardware as a scare resource to thinking hardware is free. That has created multi-agent systems because sensors are everywhere.
Now there are all these ethical issues—not that there weren't ethical issues, but now that it's pervading everything we do—computing is ubiquitous—there are fundamental ethical issues, privacy being one of them, and they're not always easy to answer. It really requires—and I think Caltech emphasizes—ethics. It emphasizes the honor code. They're tricky questions. "Well, I had a sensor out here, and it looked at all the cars going by my little cul-de-sac, and identifying license plates." We were looking at the people driving by, which is really not that hard to do now, for a Caltech undergraduate to do. I told my neighborhood here who was coming by—is that an invasion of privacy? Is that okay? I think legally, it's okay, but is it the right thing to do? All these questions come up, which are new, new meaning the last ten years, which just weren't there back then.
ZIERLER: Given your longstanding interests in algorithms, either by osmosis or your specific interest in the areas of inquiry, to what extent have you been involved in artificial intelligence or even deep learning research?
CHANDY: I haven't been involved in deep learning research at all, except to the extent that I'm interested in using large numbers of computers in clever ways. I haven't really worked on deep learning research or AI, but I follow that work because of the numbers of computers that they use to solve those problems. I'm interested in figuring out the best ways to use collections of computers. Now, a specific interest of mine, which is really not deep learning, is looking—to what extent can you as an individual, as opposed to you being part of Amazon, use your collections of sensors for your goals, without necessarily revealing your data to a company? Could you get a collection of computers, a collection of sensors in your house, in your neighborhood, where you did all the analysis yourself, without the standard approach now of sending all that to the cloud, to a company—Amazon or Google or somebody—and have them do it for you?
Again, this is a question—is it a good thing to do or not? The reason to try and do it is again for privacy. It's your data. We should be able to keep it. Is it important? And I'm not sure anymore, because it's not clear to me that most of us really care anymore about privacy, and we're quite happy to make our data available. To the extent that nothing terrible has happened—passwords get lost, but nothing terrible has happened. You might hear of some other countries where there's massive facial recognition or DNA recognition and so on. Maybe at least at this point in time, it's not an issue for Americans. That's a roundabout way of answering your question.
ZIERLER: You emphasized the ethical components of algorithms and AI. Given your interest in teaching, do you see it as your place as a professor, as a mentor, to students who are well on their way to being the next generation of leaders in the field in computer science and industry, do you see it as part of your duties to bring the ethical dimensions of this work to the fore, as a teacher? Or is that sort of beyond your area of expertise or beyond what's expected of you or what you expect of yourself?
CHANDY: It's certainly beyond my area of expertise, however let me give you a specific example. I teach the algorithms underlying cryptocurrency. Now, is cryptocurrency a good thing, or a bad thing? It's an ethical question.
[End of Recording]
ZIERLER: This is David Zierler, Director of the Caltech Heritage Project. It's Thursday, October 14th, 2021. Once again, I'm delighted to be with Professor Mani Chandy. Mani, great to see you again. Thank you for joining me.
CHANDY: My pleasure.
ZIERLER: Last time, we were interrupted by a power outage, and in the middle of that, we were engaged in a very interesting discussion about your considerations relating to the ethical or moral dimensions of artificial intelligence and computer science generally. I heard as much that you qualified saying you're not an expert in these matters, but it is important for you to consider them as a teacher and a mentor to students. The question there, which I didn't hear, is in what ways do you engage with students on questions of morality and ethics as it relates to artificial intelligence?
CHANDY: First of all, I'm most definitely not an expert in artificial intelligence. It's far from my area of expertise. The ethical questions that I was going to talk about were related more to what I do, which is distributed systems. I was going to give you an example of what I do in my class. One of the examples of distributed systems is cryptocurrency. A cryptocurrency like bitcoin works by having many distributed agents follow what's called a blockchain, but's it's essentially a distributed algorithm. It relies on having many, many, many agents participate in the algorithm. I teach it in my class, and one of the ways in which bitcoin works is that people can quote "mine" for bitcoins, and they mine by essentially solving a puzzle on a computer. The puzzle has no social value. It's merely a way to do something to get you an award, which is a small part of a bitcoin.
Now, you had an earlier question as to how things have changed over the 50 years, and 50 years ago, the key resource was a computer. Very few places had computers. Now, computers are essentially free. What bitcoin mining does it is allows you or anybody else to use a collection of computers to solve these puzzles. To the extent that computers are free, it costs nothing to solve a puzzle. However, what's not free is the energy required to run the computer, so there's a huge amount of energy, electricity, used to mine for bitcoins and to solve these puzzles which are essentially—so here comes the ethical question. Is this a waste of energy, when the planet is warming up and it's critical that we manage global warming? So on the one hand, it's a bad thing. On the other hand, the argument is that bitcoin allows transactions that are not under the eye of credit card companies, the federal government, or any other government. A libertarian would say that this is the sort of future, away from any sort of control or observation.
So it's a tradeoff, and it's a tradeoff that occurs in the area that I work in, which is distributed systems. It's not an algorithmic question; it's an ethical question. I teach algorithms, however I feel it's my responsibility to highlight the ethical issue for the student without giving my own viewpoint one way or the other, just so that they think about it. For example, we haven't gotten to bitcoin yet, but I put a question on the homework saying, "What are the tradeoffs?" The homework, they get full credit if they just think about it. This is an example of where we're just encouraging students to think about ethical consequences of what they do, without being high-handed about it.
There is a lot of work at Caltech—you should talk to the AI people, who really think about this. Most recently, there was a talk about it. It's a much bigger issue in AI. A different issue in distributing computing, which I do, is—the other thing that's very, very cheap now are sensors. A couple of dollars, you can get all kinds of sensors. That gets to the issue of privacy because it's so easy now to monitor and analyze all kinds of things. There's a legal issue as well, but it gets very, very tricky, as to what should one do, even if one could do it. What should one do, even if it's almost free? Yeah, these are questions that I bring up, and in some cases encourage students to talk among each other, but in no way provide quote "an answer."
The Social Utility of Distributed Systems
ZIERLER: We can zoom out, since we're at the portion of our discussion where we're describing these issues in very broad terms, and that is, what does distributed systems mean, and how far back does this area of computer science go?
CHANDY: Distributed systems are essentially multi-agent systems. There are multiple agents, sometimes working together, sometimes working against each other, to achieve their own goals. This is related to many aspects of science, and even economics. Going back to where it started, I would say it started in the late 1960s, when there were computers with multiple processors that had to interact. This was a relatively simple kind of distributed systems because they were multiple processes all within the same rubric, if you will, with the same machines. It was in the control of one person designing it. Then it got to be like the ARPANET, multiple computers talking to each other. Then more generally the internet, where you have all kinds of—and then the web—many, many agents interacting to do all kinds of things. Distributed really means having all these multiple agents.
In some ways, it's related to economics, because economics is all about multiple agents. The difference between distributed computing and economics is that we don't assume that each agent has its own goals and that it's necessarily rational in trying to achieve that goal. In some sense, we are designing the agents generally to collaborate rather than to compete. Now it's everywhere. Everything you see is distributed. In particular, when you come to so-called quote cyber-physical systems, sometimes called CPS, that are cyber systems, computing systems, that control physical systems all around us—elevators, cars, airplanes, air traffic control—they're all highly distributed. Sensors within a car are distributed. Sensors within an airplane. Then collections of airplanes. There's really almost nothing that's not distributed anymore.
ZIERLER: Because this technology is so relevant in industry, I'm curious if you could reflect broadly on the changing culture of both intellectual property at Caltech and also the culture of entrepreneurship at Caltech, professors being involved in business ventures and things like that, and how these may have changed or shifted over the years.
CHANDY: Professors and students—and especially students; I really want to talk about students more than professors—being part of entrepreneurial activity, either starting their own companies or going to startups, I think is a really good thing. There has been a change over the years. As I said earlier, our students would all go to grad school. There was almost no question. Everybody would think about which grad school to go to. But now it has changed, and I think it's a good thing because the students are now more actively engaged with what they do, how it impacts society, as opposed to just the mathematics of it. I think both are important, but I think this active engagement with society is very valuable. I think it's a good thing for students.
Likewise, I think it's a very good thing for faculty as well. There are some universities where I think this is not being handled well. I think Caltech faculty genuinely pay—first priority is their students, their research goals, the institution of Caltech, and do their consulting or entrepreneurship all within very strict guidelines. That's Caltech. I do think there are some institutions where that's not quite so carefully done. Again, I think it's a good thing, because computer science is in no sense any part of an ivory tower. The time from a research idea to dissemination and its impact is very short, which is the way it ought to be. So I'm generally in favor of it. Does that answer the question?
ZIERLER: Yeah. The question about intellectual property, the way that professors or students or whoever is doing research on campus that might have commercial value, the question is as much an administrative one as it is a scientific one, and that is the way that both the individual researchers and Caltech institutionally has responded to intellectual property, to going about granting patents and things like that. How have these things changed over the years?
CHANDY: My interaction with the Caltech intellectual property [office] has been amazingly good. I'm really, really impressed with Caltech. Again, I have friends in other universities where things are nowhere as good. Everything we do at Caltech belongs to Caltech. However, when an idea comes up, and it's possibly patentable, the Caltech office always asks you, "Do you want to patent it?" They could be heavy-handed and say, "Everything you do has to be shown to us, to see whether we can patent it or not." But Caltech isn't that way. They leave it to the individual faculty to decide whether to try to patent something or not. That's really nice. In my point of view, I think a lot of what we do should be made free, freely available to everybody, all over the world. There's a lot of things that I would prefer not to patent. Now, there have been some cases where I work with a colleague in industry, and we work together and come up with an idea, and before I know it, the company, jointly with Caltech, has applied for a patent. Of course that's okay, too. I think the IP situation at Caltech is again very, very good. I don't see any problems with that. I think it's the right thing to leave it to the individual faculty member to decide what to try and patent and what not.
ZIERLER: Where I'm coming from with this interest is, Stephen Wolfram shared with me his experiences, when he was at Caltech, with intellectual property, which were rather negative. I'm so glad to hear yours are the opposite. It's one of those things where I'm trying to determine—obviously given what Stephen Wolfram went on to accomplish—if the patent office at Caltech responded in a way that that kind of a situation was designed not to happen again.
CHANDY: Yes, so Wolfram left Caltech—I'm trying to remember the date—but before I came in. There were some changes in the patent office. It could be that we just experienced different people and totally different experiences. Of course what Wolfram did is great. Looking back at Caltech's history, especially computer science history, there's some places where you say, "Oh my gosh, I wish we had done something differently." Wolfram was one of them.
ZIERLER: For you, when you go to your faculty page and then you click on "Research Group Website," it brings you to assemblesoftware.com, and I have no more context as to what assemblesoftware.com is, its origins, who some of your key partners are there. Let's first of all start with the name, Assemble Software. What is meant to be conveyed with that term, Assemble Software?
CHANDY: Before I get there, the dot com is unfortunate, because the idea again with that website is to make everything free, so anybody can take it, modify it, patent the modifications. So the dot com suggests an entrepreneurial activity, which is not the case at all.
ZIERLER: What would be better? .io? .org?
CHANDY: .org would be the right thing, I think. That's a mistake, .com.
ZIERLER: Just the name, then. Assemble Software; let's start with that.
CHANDY: The idea of Assemble Software came especially with working with all these sensors, is that the way hardware has progressed so rapidly is that we're used to thinking about components, and each component is tested. There's a library of these components. You pull these components together and then you plug them together to get larger components. The focus and design is not so much designing a component, but figuring out which components from the library are useful for a given problem and how you plug them together. The name Assemble Software came from this emphasis on thinking about software modules that should be plugged together, and the focus of designing a module and how to put together, as opposed to just writing software as such. The particular application domain that I was investigating initially were collections of sensors, which again are very, very pluggable. Sensor for acoustics, sensor for vision, and you often want to plug them together to achieve some goal.
ZIERLER: When did the website launch?
CHANDY: Five years ago, [perhaps]?
ZIERLER: This was a post-Emeritus initiative?
CHANDY: Yes.
ZIERLER: Was it something that you could have done earlier but you didn't have the bandwidth as a full-time professor?
CHANDY: Yes. Because there's a lot of coding.
ZIERLER: You mentioned that. You wanted to get back to coding in retirement.
CHANDY: Yeah. Professors really shouldn't spend a lot of time coding, because coding does take a lot of time. It's more like a mechanical engineer retiring and working on a lathe. It's just a fun thing to do, but it's not necessarily the right thing to do while you're a professor.
ZIERLER: Who have been some of your key partners in this initiative?
CHANDY: Mostly [laughs] some of my students, and some of my ex students who have gone on to various companies. This was motivated by the work at Caltech on the Community Seismic Network, the work I told you about with geologists and civil engineers. Because that's very much an Assemble Software application. There's thousands of these sensors, and they're all assembled together to understand what's going on underneath the Earth. That's what motivated it. What we could do with sensors for earthquakes, we could do for all kinds of activities.
ZIERLER: And properly named, it would be a .org; this is for anybody to use freely. Who are some of the—customers and clients would not be the right word, because that would suggest a commercial aspect, but some of the end-point users?
CHANDY: The end-point users for that are the same as the end-point users that I'm working with for my other website, which I use for the course. They are really students who want to explore ideas. It's very much grassroots. Again, my vision is somebody in Africa or India or something gets sensors for two or three dollars, computers are cheap—"I want to try something out. Is there someplace I could go to get some ideas for what to try?" Some of the applications deal with looking at streams of data from anywhere. The data could be from sensors, but the data could also be from text. It could be texts produced by Caltech. It could be text from The New York Times. One of the students had the idea of looking at text on Google or elsewhere, to identify whether COVID was more likely to occur in a given community based on the frequency of searches or the frequency of texts dealing with that.
ZIERLER: I remember reading about this.
CHANDY: Here you've got a stream of data, and a student wants to ask this question. Then they can ask that question by writing a lot of code, or by going to a library and seeing what's available and plugging it together to help them answer their question. This saves them time. The abstraction is that there are streams of data being generated freely now—all kinds of data, webcams, and all that—and how can you use them, not to monetize them, but how to use them for whatever purpose. This was something that a student did. This would be my target audience, a student somewhere in the world saying, "I'd like to try this. What's available?" I hope that one of the things they'd find available was this website. The same thing with the class I'm teaching; again, the goal is to make it—it's kmchandy.github.io—let's make it freely available to anybody. The target audience is typically a curious undergraduate.
ZIERLER: I'm particularly intrigued about how the sense and respond applications you're developing—as you say, they run forever. Can you explain first from a technical perspective how that's possible, and then what is the value of running forever with some of the ways that these sensors are applied?
CHANDY: The work on computer science in the 1950s, 1960s, 1970s or even later, was mostly about a program which you submitted, it ran, and it finished. You had data as input, ran it, and you got an answer. It was a very limited view. There was a time span within which a problem was solved. There's a certain kind of analysis, a certain kind of mathematics, to show that these programs work correctly. In particular you want to show that when they stop, what they produce matches what they are expected to produce for that given input.
What happens with many, many applications today is that there is no end point. You think about Amazon; think about just about anything. Certainly way back in the 1950s, the Defense Department, when they started looking at command and control, were looking at systems that operated "forever," forever in quotes. Because there was no point at which you could stop and say the problem was over. What happens when the problems go on forever is you think about these problems in a different way. Especially when you're trying to reason about them, you're not talking about, "What do I get at the end point?" but "What do I get at every point?" There's this whole notion of—there's a scientific term called progress in the computation, where the progression now is over this infinite time horizon. Things are getting better over this time horizon, as opposed to the earlier work, where it stops and you just see what happens when it stops. It's a different mindset when you think about the endpoint versus thinking about all the time.
ZIERLER: These oral history discussions are such a wonderful opportunity for researchers and professors to convey their day-to-day craft to a broader audience who might only have a vague notion of what you do on a daily basis. I'm intrigued by this idea, as you emphasized, that coding takes a long time. For those of us who only have a vague notion of what exactly it means to code, take us there in the moment. You've had your coffee. You're sitting at your desk. You're ready to code. What does that look like, and why does it take so long?
CHANDY: Coding doesn't necessarily have to take a long time. I talk about two kinds of coding. One is where you've got an immediate problem to solve. You just want to see what the answer is, you code, get the answer, you get done. The other is when you're building an artifact, an infrastructure, for others to use. For that infrastructure to be usable, it has got to be crafted really carefully, so you've got to build—it's like architecture. You've got to build the components so that they can put one on top of the other, and where the interfaces are very clear, so that they can be coupled. And you often make mistakes. You start off with one architecture and say, "Oh my gosh, it doesn't work." Back up, start again.
The word "coding" is sort of again unfortunate because it suggests the actual writing of the code, whereas I'd say 95% is more thinking about how to organize the pieces, what the pieces are, how they are organized, what the interfaces are, also what the documentation is. This is I think important, because as a lot of younger and younger people start coding—there are all these classes, companies that teach very young people to code in, say, Python—I think there was a story where Bertrand Russell was sitting next to somebody, and she asked Russell, "Well, what do you do?" He said, "I'm a philosopher." And she said, "Oh, we finished it last term." They might think, "Well, I'm finished with all this coding." Which misses the point. That it's not the actual writing of the statement; it's thinking through the architecture.
ZIERLER: As you well know, it's something of a parlor game to think about in the future which career paths will become overtaken by AI, things that will no longer need to be, or be best performed by humans. Where do you see coding in that?
CHANDY: A lot of coding will be overtaken by AI, so that if you know exactly what a given module of code is supposed to do, so you know what its inputs are, and you know what its outputs are supposed to be, that I think will be overtaken by AI, especially if efficiency isn't a critical requirement. But I think what's not overtaken by AI is more the thinking through the architecture, because that's really an art. It's really trying to create something like a painting where the parts of the painting can be generated by AI, but thinking through what the painting ought to be is not an AI question. Maybe that's the way to distinguish it. The design question is, "What should be?" Once you know what should be, and especially if you break the "what should be" into small parts, then I think AI will take over. Elementary coding I think will be taken over, but the overall design will not be.
ZIERLER: Because the overall design will still require human imagination, decision-making about what should be worked on, that it will not be in the province of AI to make those decisions, you're saying?
CHANDY: Not for a long, long, long time. It's, as you say, imagination, creativity, even [having] good sense—[laughs] I'm thinking of sense almost in a cultural way. There's people of good taste, and there's a good taste in how you create these architectures. Someday AI will get there, but it will take some time.
ZIERLER: I'd like your take on how you feel about the word or the term "the cloud." It's sort of funny, because in reality, it's anything but. These huge buildings that use enormous amounts of energy, that have all these enormous computers in them, that are vulnerable to all kinds of infrastructure threats. In what ways is the term "the cloud" aspirational, because at some point in the future, what we mean by "the cloud" today will be much less bound physically than the current way we have to store all of this data?
CHANDY: There are many, many aspects to that question. The initial impetus for the cloud is looking at what are the scarce resources. The scarce resource used to be the computer, but no longer is. Computing is free. The scarce resources are people and energy, people in particular. It takes an IT person to manage a computing system. Even in a home, you have some kid who's the IT person for the home. The impetus, the economic impetus of going to the cloud is that a large number of computers is managed by a relatively small number of superb IT people, and they take care of it for you, so you don't need your own IT desk. That's a very powerful argument. That's part one. Part two is that because the quote "cloud" can afford to have huge—I mean, amazing numbers of computers—they can manage fault tolerance within the cloud better than you could with your own connection at home, or in an institution, because if something fails, it can flip over to somewhere else. The different question that you brought up is points of attack. When there are a million people, each with their own little IT systems at home, the points of attack are more diverse, they're more distributed.
ZIERLER: More localized, even.
CHANDY: More localized. You can try and attack the network, but you could attack either Caltech or Stanford or somebody else; you wouldn't attack the entire infrastructure. Now, there are these large points of failure, so Facebook goes down for six hours, and the whole world—Africa, India, Indonesia—suffer. To use a phrase, we're putting all our eggs in a few baskets, and we are watching over these baskets very, very carefully. Which may be the right thing to do, but you've got to watch them so carefully that they never spill over. They do provide direction for hostile agents, hostile countries, to attack, because you become so vulnerable because of these relatively few spots where the attack can reduce a country to suffer very badly.
The cloud question goes on and on, this question of data. For example, we store our data from the Community Seismic Network first on the cloud, but we also keep a copy locally, partly because data is so cheap. But as the centers are generating data, it's easier to send them off onto the cloud, do some analysis there, and then keep our own archival copy for ourselves for the next 100 years. It's a long discussion. I can keep going on.
ZIERLER: Looking to the next 100 years, and of course your answer by definition will be provisional because I'll ask you to imagine where things are going, but to the extent that we can appreciate that our current way of doing things with the cloud—like I said, these enormous energy-intensive buildings—in 50, 75, 100 years, in the way that we might look at the steam engine today or some antiquated technology, what might the cloud look like, or the way we store our data? What might it look like where we can get beyond the obvious shortcomings of the cloud now, with regard to energy use, with regard to vulnerability to attack?
CHANDY: Interesting question, but you have to be careful. I'm going to push back a bit and say that for both the questions that you asked, that the cloud solution might be the better one. Again, there's a tension. Let me look at it question by question. Energy—a cloud computer can set up energy in Alaska, or somewhere where there's a lot of hydroelectric power, whereas if the same amount of money was distributed all over the country, the demand for energy could be less efficient than having it in areas where it's easily or cheaply available. So it's not clear that the cloud necessarily wastes energy. It does consume a lot of energy, and it's centralized at that cloud point, whereas the users, if they were to do all that computing locally, would have it all distributed. Having it in a cloud location is not necessarily a bad thing. It could be, but it's not necessarily a bad thing.
ZIERLER: Cheap energy could mean coal-fired energy, though. There is that consideration as well.
CHANDY: It could be coal-fired energy, however it could be coal-fired energy if you're doing the same computing in your home. That's part one, then. It's not clear that you're using energy less efficiently by having it in these cloud warehouses. Now part two [laughs], which is a social question, which is sort of beyond computer science, is that because it's so easy and it appears to be totally cheap—you do a Google search; from your point of view, it is completely free. When a resource becomes free, it tends to be overused. I believe that we are overusing these cloud services because they are free for us. But they're not free to the commons, the commons in this case being the world. The commons is using up energy. This is the tragedy of the commons in this sort of situation, right? Because there's so many things that appear to be free to us which are really taking resources from the commons.
ZIERLER: To use that metaphor, the shepherds who are using their sheep in the commons to graze, who's losing out in these commons, in these digital commons, with the overuse of these seemingly free technologies?
CHANDY: Well, the world is, because at least today, we're using energy that isn't all green. At some point in the future, it will become all green. The good thing about these cloud locations is that one could imagine that they're located where green power is available.
ZIERLER: Would you include in that this amazing research that the Brens are supporting at Caltech to capture solar energy in space and to beam it back to Earth? In the way that you talk about Alaska, some faraway place that we could draw energy on for these clouds, would you extrapolate that and say that this is a possible technology that would be relevant for resolving or making this problem better or more sustainable?
CHANDY: Definitely, definitely. Again, the key changes are what's the scarce resource. Computing is now free, and the scarce resources are energy and people. For both reasons, the Bren Center makes perfect sense.
ZIERLER: Thinking about space, I can't help but wonder if JPL has been an asset to your research at all, or conversely, if you've done things that have been of value to JPL?
CHANDY: We worked with JPL in two ways. One is that our Community Seismic Network, we have sensors all over JPL. That's important at JPL, because it's such a valuable institution for the country, and it's critical that after an earthquake, that JPL knows which buildings are damaged, where they're damaged, and how quickly they can come back. That's sort of a side issue. It's a practical value that we had some research done, we built an instrument, and the instrument is being used at JPL, to help protect JPL.
More broadly, I've worked with JPL primarily on questions of helping ensure that their programs are correct, because the consequence of a failure for a space flight, the cost is so enormous. We're mostly collaborating with JPL scientists on working on helping to ensure that the code is correct before the space flight goes up. The connection to JPL has been very valuable. It's nice to do research and then come up with something that's immediately available to a sister institution like JPL.
ZIERLER: An administrative question—you're in the Department of Computing and Mathematical Sciences, but the main schema at Caltech is at the division level. Where does the Department fit in within Caltech's divisions?
CHANDY: It fits in within E&AS, Engineering and Applied Science. The secret sauce of Caltech is that the divisional walls aren't that rigid. It's really Caltech's secret sauce. Without it, an institution this small couldn't survive in the competition. There's a lot of interaction now between Computer Science and Economics. It's sort of a natural interaction. Something new, for example, is if you look at Google Ads, the way ads are served, that's an economic problem, but it's very much a computer science algorithmic question as well, so there are people at Caltech working on that. There are ties to biology, and the key questions of protein structure are really computational questions.
Getting back to your point, having it within E&AS is a helpful administrative structure, because you need some sort of administrative structure. You can't be completely flat. There's a structural issue for administration, and the different question is what's the structural issue for the dissemination or diffusion of knowledge. The power of Caltech is that regardless of what the administrative structure is, the diffusion of knowledge, in my view, happens much more broadly in Caltech than at other institutions. Somehow we've got to keep encouraging that because that's really our secret sauce. I got my degree at MIT. Well, I don't want to appear to be dissing MIT, but it's so big that people in different floors of Tech Square don't get to talk that much. I'm trying to think of benefits of having a smaller size, and perhaps the key benefit of small size is that it's easier to talk across divisions or departments.
ZIERLER: On the topic of talking across divisions or departments, you mentioned some opportunities of synergy between computing and economics, for example. What about between computing and mathematics? There the question about the distinction in divisions and sciences are, there's Mathematical Sciences, of course within the Department of Computing and Mathematical Sciences, but there's also a Mathematical Department in PMA. So for your work, are there obvious routes that you would take in terms of interacting with mathematicians in PMA versus within your own department?
CHANDY: I'd really like to have that question asked for the theorists in our department. There are many commonalities. One common area is combinatorics. Combinatorics is a branch of pure mathematics that has been that way forever, but it's absolutely critical to everything computer science does. Perhaps the way of phrasing the question is, the combinatorics that computer scientists do is often with a practical end goal in mind, trying to solve a specific kind of problem. Even the Google AdWords problem is a combinatorics problem. Whereas I think a pure mathematician would have the luxury of just asking the question for itself. Another common area is probability theory, and a lot of what we do is based on probability. All the queues that go into a computing system, it's probabilistic. Many of the algorithms are probabilistic. But the difference in emphasis is more phrasing the question—using probability for some fairly well-defined end goal that the computer scientist has in mind, whereas if you're asking the question primarily from a curiosity point of view.
ZIERLER: For you, to the extent that there is this distinction between pure mathematics and applied mathematics, the kinds of questions you're asking or you're after, you would be just as likely to go to a pure mathematician as you would somebody that does more applied mathematics?
CHANDY: No, actually. I'm more likely to go to somebody doing more applied mathematics, because they would be motivated by the same sort of end questions that I would be. Conversation is a lot easier within computing and mathematical sciences. I should also say something about Caltech, which is that Computing and Mathematical Sciences as a department, is an anomaly if you go across the country. There are computer science departments. There are computational science departments. There's sometimes applied mathematics departments. But to put them all in the same rubric of computational and mathematical sciences, it's an anomaly. It's different. I think it again shows the power—I don't know if power is the right word—the difference in emphasis at Caltech. The presumption is that somebody doing mathematics, differential equations, whatever it is, and somebody building a system, writing code, that there's no intellectual barrier between the two. Somebody building a very practical system is expected to understand whatever mathematics is produced in the department. It's not like there are people who build things and people who do math; it's all one thing. That's unusual, and it's not the case in most institutions.
ZIERLER: Relatedly, but perhaps even a more fundamental question, as a computer scientist, as opposed to a computer engineer, if you were to ask, for example, a chemist, about the distinction between their approach in applied science versus basic science, there would be a basic understanding that the fundamental research is just understanding DNA, for example, and the applications of that understanding might be for translational research, for human health or whatever. For you in your fields, what is the fundamental aspect of your scientific research? What are the things that you're trying to understand about computers, about people, about how things work at their most elemental level before you even begin to think about ways to apply that fundamental knowledge?
CHANDY: Again for me, for distributed systems, it's a fascinating question of how you orchestrate a collection of agents—that's the word we use—each carrying out its own activity, but that the orchestration achieves a common end. You could be anthropomorphic about that, and we often are anthropomorphic about that, because in computer science, we talk about, "What does an agent know?" Of course "know" is a human characteristic. Software doesn't "know" anything. But it's helpful to think about what an agent does know, what does an agent say, as these collections of agents end up doing something.
The fundamental question is, there's this notion of orchestrating large numbers, collections of agents to achieve a common end, and how does that orchestration take place? Because the orchestration, if it's centralized, if it's some central commander telling everybody what to do, then that's no longer purely distributed. Because again you have one locus of control, which if attacked could cause the whole system to fail. So to what extent is the orchestration truly distributed? Getting back to the earlier point about bitcoin, one of the ideas of bitcoin is to have things so distributed that you don't need a bank. You don't need Federal Reserve. Again, getting away from the ethical question, the fundamental question again is, how do you do this orchestration to achieve a common end?
ZIERLER: Another key binary—we talked about fundamental versus applied research—is in science, of course, there's experimentation and there's theory. In your world of distributed systems, talking about orchestration, where are you experimenting, in a laboratory, even if only that laboratory is metaphorical? And where are you developing or relying on theories that both guide the experimental questions and serve as a framework to know if the experiments or the propositions are on the right path or not?
CHANDY: Actually, because the big quote-unquote "experiments" in this instance are people building systems, usually companies building systems, or startups for big companies, the progression of theory and experiment happens very close together. You come up with an idea, and if it's practical, the computer would use it very quickly, within hours. There's so many of our students and so much interaction with companies that questions in industry, when framed properly, become theory questions. The issue is really framing, because an industry question might be, "How do I solve this particular problem on this particular system?" What an academic could do, or should do, is to frame that as an extraction not just for that particular problem but for a class of problems. In that sense, that framing takes the problem from an industry-specific "What do I do today?" problem to a theory problem which would be valuable, hopefully, for decades.
Even just today I got a message from one of my students working now for a very big company, who has a very practical problem and was interested in again this question of framing. How do you think about this in a more general way? If one were to think of a characteristic of some of the greatest computer scientists in this area, it's this ability to identify a very practical problem, frame it as an abstraction, and then have it apply more generally. Some people have a knack for doing that, but it does require a considerable amount of practice because that's where the problems come from. There's very little point in framing questions that don't arise in practice. But then how do you think about this as abstraction, and what problems do you extract?
Computers and a Theory of Everything
ZIERLER: Is there an effort in theory and computation that things are moving toward a convergence? In other words, in the world of physics, the emphasis on achieving a so-called theory of everything, to bring it all together. From the earliest days in computer science, in the late 1960s and early 1970s, is there a longstanding effort to bring theory to some measure of convergence or singularity? Or by definition is distribution, the goals of distributed systems, does that make it allergic or inimical to those kinds of ideas?
CHANDY: It's an interesting question which I hadn't thought about. A theory of everything, going back—Einstein, Bohr—you could phrase that question, "What is the theory of everything?" Now, I can't think of any prevalent framing of a question that would capture all or most or even part of computer science.
ZIERLER: Like, physicists know that at some level we need to have a theory in which quantum mechanics and relativity work together. There's that understanding that until we get there, something fundamental is missing. Is there something similar in the world of theoretical computer science, where there are these massive schools of thought, and we know that there's something missing because things need to work better together?
CHANDY: I'll give you an example. It's not an exact analogy to the theory of everything. I think I raised it briefly last time. It's the question, "Is P equal to NP?" Are there classes of problems that are just fundamentally difficult and cannot be solved efficiently no matter how bright you are, how smart you are, or not? There's something called the Millennial Prize. Are you familiar with that?
ZIERLER: Yes.
CHANDY: One of the questions on the Millennial Prize is this one—"Is P equal to NP?" That has been there since the early 1970s, so there are these fundamental theory problems that are yet to be solved.
ZIERLER: But the assumption is that they are solvable, at some point?
CHANDY: Yes, yes, yes.
ZIERLER: As a pie chart, in terms of what it will take to get there, how much is ingenuity? How much of it is the next genius coming along? How much of it is we need more advances in technology and in computation?
CHANDY: I think it's ingenuity. It's really some collection of very bright people working together. The presumption is that the two classes are different, that there are really fundamentally hard problems, and to somehow separate the easier problems from the hard ones by finding intermediate steps showing that there are barriers between them. This is not a question of computational technology; it's more a question of brains. Brains this the wrong term again; it's small steps in getting there. It'll get there. But it's really ingenuity; it's not technology.
ZIERLER: Ingenuity and hard work, grinding it out, day after day.
CHANDY: Yes. I think it's going to be grinding unless somebody comes up with this brilliant view and all of a sudden solves the problem.
ZIERLER: On the question of theory and experiment and application, you have quite helpfully summarized your research career around this framework in seven different areas. I'd love to engage you briefly on each of them. First, number one, performance modeling and evaluation—what are you modeling? What is the performance that you're modeling and how do you evaluate it?
CHANDY: Within a computing system, within a distributed system, there's pieces of information traveling, all through the system. Within a computer, for example, you submit your job, your task, and it goes into a computer, it goes into the cloud, and so do many other people. The times at which you submit these jobs are probabilistic. They're random. We don't know for sure that David is going to send a problem in at ten of five. So the underlying workload is fundamentally random. And these workloads form queues because they need access to scarce resources. The performance is how these queues are handled, what's the best way to handle them, so that these jobs come out.
With the internet, you get the same sort of thing. There's traffic along the internet. You get congestion points with the routers. The performance question is, how do you analyze the overall system, which is fundamentally a probabilistic system? That's where the performance modeling comes in. This relates to an area called queueing theory. You have queueing theory problems in airports. If you go to LAX and you stand in line for TSA, and then you stand in line to get your ticket, and you stand in line for something else, this is a network of queues. You see this problem in computers and outside of computers. This is case where mathematics is applicable, is applied to computers, but the mathematics is very general because the things that are forming queues could be people or computing or whatever.
Again, I think that gets back to an earlier point, that it's really important not to think about the problem in a limited domain as, "This is something for computing." Here's a problem that's an abstraction that's very widely applicable, and then you think of one application, which is computing. In that sense, you're going from experimentation to systems to theory. The key issue is the abstraction. It must be the same in physics when you're going from making a mathematical abstraction of some observation.
ZIERLER: That's exactly right. We haven't yet talked about simulation. Next, your work on parallel and distributed simulation. What's the difference between parallel and distributed in the context of simulation?
CHANDY: It's really the same thing. Some people call it parallel; some people call it distributed. It's now sometimes called PADS, Parallel and Distributed Simulation. It's an interesting problem. I'm going to try and explain it. The way a simulation of a physical system works, with a computing system, or an economic system, is that there is a central controller that tells—so you're simulating a system with many, many agents. The agent could be in physics. It could be some part of a cell in a climate model. In chemistry, it could be a molecule, or in a larger system. The way the simulation works, we want to see how two molecules interact at a given point in time. Typically there's a controller that says, "Now, you two molecules tell me how you interact at this point in time." So there's something stepping forward in time with a single controller. That's really crucial for simulation, having a time-stepping manager that steps through physical Newtonian time as these agents interact. But of course what's happening is simulated time. There's a relationship between physical time and simulated time.
The question that came up for some very large distributed systems, and even non-distributed systems, is can you get rid of the central controller. The philosophical question is, can you sort of get rid of Newtonian time by having each agent have its own view of time, so there are in some sense multiple clocks, but somehow even with multiple clocks—these different agents are using their own clocks—the resulting simulation as to what agents do matches what would happen in the physical domain. To me, it was a really fascinating theory question. Can you get rid of this clock? It happened to have some very practical consequences because it made some simulations much faster, especially simulations of circuits, of circuits and chips. It's a difficult question to—but I'm trying to give you some of the excitement about framing a question—"Can you get rid of the clock?" That was an example where the question was asked first, just because it was fun.
Then talking about grants, we went to the Air Force Office of Scientific Research with this question. "Here's a fun question." They funded us, not a large amount of money but enough funding to have students think about this. Then it so happened to have some practical value. So that was a case where, getting back to your earlier questions, it went from just theory, curiosity, to something practical. It may not have succeeded. The theory might be interesting but not be used, but in this case it happened to be.
ZIERLER: We talked about simulation, and of course you operate in a classical computing framework. So much of the excitement in quantum computing is the power of simulation that quantum computers will be able to do. On that point, in your work on distributed simulation, parallel simulation, are there areas of obvious shortcomings that classical computers simply can't do, for which quantum computing might be particularly exciting or helpful?
CHANDY: Oh, yes. I think classical simulation, there's a fundamental problem, which is that you try to get one piece of computing hardware to model essentially a collection of agents, where an agent could be a cell in a climate model, and then modeling it by modeling each part, each agent for a given instance in time. So do this agent at time t, then the other agent at time t, and so on. If you're trying to simulate something really big with a smaller collection of computational power, moving through time one step at a time—quantum computing allows us to break through that particular paradigm. Again, it's not so much for modeling computers or the internet or distributed systems so much as modeling physical systems. It's a different paradigm shift. I think it's going to make a breakthrough. We aren't there yet, but I think it's going to make a huge difference to science, and people who do simulation have got to think about that very carefully now.
ZIERLER: Next is distributed algorithms. What about algorithms needs to be distributed? What does that mean?
CHANDY: This is again having multiple agents collaborate to achieve an end. Each agent is pursuing its own algorithm, but somehow the orchestration achieves a common goal. That's what the distributed algorithm means.
ZIERLER: Would this be one aspect of your research for which theory is especially useful?
CHANDY: Oh, yes, yes, yes. Again, what I'd like to say about theory is the emphasis is on abstraction. There's a collection of practical problems, and you abstract it into a more mathematical question. It's not theory divorced from practice; it's theory obtained by abstracting practice. Sometimes the theory question comes first because somebody has an abstraction in mind. Sometimes it's by observing practice. I'm getting on my soap box here, but it's important not to divorce theory and practice. It sounds like there are a bunch of theoreticians and a bunch of engineers. They've got to be tied together very carefully, very tightly.
ZIERLER: You pose such interesting questions on this topic. Can a process learn the global state of a distributed system? What is a global state?
CHANDY: How shall I phrase it? Looking back at the cloud, there are multiple locations where cloud services are performed, all over the world actually. What you'd like to do is to, for example, have traffic load, when you put in a question on Google, travel to the location where it's best handled. But making that statement—"where it's best handled"—suggests that there's a view of the entire globe at that instant to decide where the best place for this is. This is a global state, it's a global view, at that instant in time, as to what's going on. But this gets back to a little about physics. The problem is that if you don't have precise clocks, if the clocks aren't synchronized to the nanosecond, you don't really have a global view, because each location, each agent, has its own view, because it has its own clock. So when one agent says it's 10:00, and another agent thinks it's 10:01 or 10:02—so what you really have if you have everybody record their states at some time, when they think it's 10:00—so you've got a jigsaw puzzle where each element of the puzzle is what each agent records its state, and it says, "This is what I think my state is at ten."
You get all these pieces together, the jigsaw puzzle, and you put together the puzzle, what you get may not be what actually happened at 10:00, because of clock drift. Now, in the future, clock drift will go away because we all have access to some sort of physical mechanism that guarantees precise timing. But today, there is clock drift, and there's clock drift regardless of how good computers are. So the jigsaw puzzle we put together might give you a wrong picture of what's going on. The abstract question is, how can you get the pieces of the jigsaw puzzle that guarantee that when you put them together, you get a right view of what's going on? That's an interesting tricky question. It's not a hard question to solve, but that's a framing of the question.
ZIERLER: When you ask what does it mean for a process to learn something and to forget its basis of learning, to what extent is that as much a philosophical question as it is a computer science question?
CHANDY: It's a philosophical question, and the philosophers have studied it. Philosophers have got axioms of knowledge. But in computer science, here's what it means. Right now, when we are conversing, we have what's called common knowledge. That is, I know that you know that you're there, and you know that I know that you're there, and the depth of knowledge goes infinitely, so I know that you know that I know that you know something is going on, for example that I've got a picture back here.
Now, suppose we didn't have this instant interaction and we were communicating only through messages, and these messages were in the mail, so they took arbitrary time to get there. And suppose I know that you're wearing a blue sweater. I can't see you now; I only got that knowledge by means of messages. What would that mean? That would mean that you couldn't take your sweater off until I gave you permission to do so. Why? Think about it now. If I've got this knowledge only by means of messages from you, and I know this as a fact, now suppose you took your sweater off without telling me. Then I would know a falsehood, because my knowledge hasn't changed, my state hasn't changed, but your state has changed. To have me really know what's going on, it implies an issue of control, and I can control whether or not you have a sweater on. This is because we are exchanging information by messages. If however we have common knowledge, which we have now because we can see each other, I know at this instant that you have a sweater on. If you take your sweater off, I no longer know that.
So there's a very fundamental theoretical issue between what's called common knowledge—and common knowledge is very much based on this notion of time and that we have this particular instant in time where we share the knowledge—versus knowledge in distributed systems which is not common knowledge, where the knowledge is limited based on messages. It's really a fascinating topic, and I could again go on for a long time. Computer scientists sometimes do go on writing about this. It also has a practical benefit, in that when you design an algorithm, each agent is making a decision on what it knows, so you've got to define what knowledge means in that context.
ZIERLER: When you ask about network of processes determining whether it is deadlocked, first of all, what is "it," and what does deadlocked mean in this context?
CHANDY: If I am waiting for you to do something, I've asked you to do something and I'm going to keep waiting until you do it and give me your response, and to get that task done, you need to wait for somebody else to return something to you, and that person is going to wait for me, then we get deadlocked, because I keep waiting for you forever, you keep waiting for the other person forever, and he keeps waiting for me forever. The deadlock could arise in computing systems; it could arise in human systems. In human systems, we presumably wait long enough and say, "Something's going wrong," and we detect the deadlock. But deadlocks arise in all kinds of systems. Detecting a deadlock in a computer system is coming up with an algorithm by which these agents can detect when any collection of them are deadlocked. It's a practical problem. It's also an interesting theoretical one. What's nice about many of these problems is that they really are anthropomorphic. You can think of an agent as a computer agent or a human being.
ZIERLER: It really doesn't matter.
CHANDY: It really doesn't matter. It's the same theory, same everything. One just assumes that human beings will be creative enough that if they waited for a couple of days and nothing happened, that they would try and find out what's going on.
ZIERLER: [laughs] Ideally, at least. In your work "determining formal methods for proving program correctness," the first question there is, correctness implies a certain value judgment. So who gets to determine what program correctness means? What's correct and what's not correct?
CHANDY: Correctness does not imply value judgment. A program is specified—so think about space. Think about the kind of work that JPL does. The program has a very specific goal, and that goal is specified somewhere, so the person in JPL making the specification—"This is what I want this program to do"—is not necessarily the same person who actually implements that program. There's a contract between the person making the specification—"I want you to build this, to do this"—and the person actually doing the building, just like there's a contract between somebody building a home and the constructor building it for you. Now, the question of correctness is—is the person who built the software correctly satisfying the contract? Or could there be some weird place where the contract is not satisfied? The problem is that for these fairly complex systems, it's very difficult to test, because there are so many things to test. There are so many things that could go wrong. In theory, you do a simulation, and the simulation would be so extensive that every possible situation would be handled by the test, and the test would show up something going wrong. You see this with autonomous vehicles, for example. You can do a lot of testing, yet things still go wrong.
The question for correctness is not a value judgment at all. The artifact that has been built by the builder, does it satisfy the conditions imposed on the builder by the specifier? So really that's very important. It's really not a value judgment. It's all about contracts. Because the person who created the contract had a certain expectation in mind as to how this would work. And if it doesn't work that way, the whole systems collapses.
ZIERLER: Your work on parallel processing mentions C, C++, and Fortran. Is Fortran still relevant?
CHANDY: [laughs] This must have been some time ago. This was done some time ago.
ZIERLER: But not 50 years ago?
CHANDY: No, no. This was done when Caltech had an NSF center with Rice University. The focus of the center was using computer science to help scientists, physicists, chemists, and so on. We had a lot of physicists and chemists participating in this. At that time, they were using a lot of Fortran. Why were they using Fortran? Partly because the coding had developed over decades, and many of them had then switched to C++. The computer science question was, how do we help these physicists and chemists and so on, who developed their code for the computers of the 1960s, to use that code for the computers of the 1990s? When the physicists had developed the code in the 1960s, they had one processor. In the 1990s, you had not thousands but 50, 60, sometimes 100, so now you've got a mismatch between what the expected hardware was that physicists wrote for, and what's available today.
There's two views as to how to handle this. One view is you start from scratch, discard everything, think anew about what's available. The other is more incremental. You spent decades developing Fortran and C++; let's figure out how to tune it, make incremental modifications, to fit what's available. In this particular center, we were exploring the incremental ways of doing it. Fortran was very much in use then, and the question was, can we tinker with it, use all that code but make small modifications to help the physicists continue with thinking about their code in terms of what they'd initially done in the 1960s but having it run faster on a different kind of machine. In some sense, the underlying foundation changed, but the abstraction remained the same. It's again a fascinating problem, and surely somebody working in 1960 or 1970 on a physics model didn't and shouldn't have had to think about what a computer might look like 20 years from now, partly because most of us don't think of our software living that long. Most of us think of software lasting months or years, but not decades.
ZIERLER: In developing program frameworks for IoT and social media, first, what is IoT?
CHANDY: IoT is the internet of everything, the internet of things. This gets back to this notion of sensors, that there are sensors everywhere, literally everywhere. Sensors get free, computing gets free—again, back to Assemble Software, how do you help the ordinary person leverage all this free stuff to do things that are useful to them? Clearly the big companies are going to be using this in a massive way. I can already see it happening. Every big computer is using sensors. Sensors in the home. The idea for this IoT was thinking that's true but also thinking about how an undergraduate could use some of the same technologies for their own applications.
ZIERLER: In the way you construct the sentence—"frameworks for IoT and social media"—is that to suggest that on some level, social media is outside the bounds of the internet of everything?
CHANDY: No, no, because social media also generates streams of data. Facebook generates streams. Google—there's streams of typically textual data but also videos and images. The world is just generating huge and continuous streams of data in all kinds of forms. The computer science question is how can you help people exploit these streams of data, for their purposes? The example that we had earlier was this student looking into COVID. There are many others. I think this is going to be a fascinating question for a long time, because the amount of data is just unbelievable now, and it is being created second by second.
ZIERLER: In your emphasis on developing these frameworks as they are applied to crisis management, what are some of the applications that are currently in use or in train, and what might be additional ways that sensors can be used for effective crisis management in the future?
CHANDY: There are two examples of problems we have worked on. One is the earthquake. We talked about that. The crisis of course here is the Earth shaking and then buildings collapsing, and helping first responders go to the places where they are most in need. The L.A. Basin is a huge basin, and different parts of the basin operate very, very differently.
ZIERLER: Seismologically, you mean?
CHANDY: Seismologically. Very differently. Even things that are fairly physically close could behave quite differently. You want to know which buildings to go to, which floors of which buildings. There are all kinds of issues. This is a crisis management issue. It's both a crisis management issue—it also helps decide where to build once you understand the underlying geological structure of the Basin. Here's an example where you are using computer science sensors for a humanitarian purpose, which is dealing with earthquakes.
Another area that we worked on with some funding—it's an interesting problem—is detecting dangerous radioactivity. The motivating problem—this was some time ago—was a terrorist with a backpack having nuclear material, walking into the Rose Bowl, or leaving it in a garbage can somewhere. This is very close to physics, now, and actually a physicist is working with us a lot. You have sensors that physicists have developed to detect radioactivity, and the trick now is to use distributed agents to hone in on the location of the radioactivity, if there's one source for it. As a physicist, you can see where the mathematics comes in, because it's going out as a sphere, and the closer you are, the better your detection. How do you orchestrate a collection of multiple agents, for example police cars, to hone in on where this dangerous radioactivity is? Again, it's distributed computing, physics, and trying to help deal with a crisis. There are many, many such problems. The crisis management was more because it's simply something useful for mankind.
ZIERLER: To extrapolate into the future, what are some potential applications in crisis management for which this technology might be relevant?
CHANDY: The technology again is an abstraction. I think it's relevant to everything, from pandemics to tsunamis. A lot of situations happen very rapidly, and happen geographically distributed. You're trying to get a global view of a rapidly changing system where the data is available in very local pieces. You're getting info about COVID in a given hospital or a wave in one part of the Atlantic, and you've got to put all these pieces together. It's a jigsaw puzzle. And it's tricky, because when you put the puzzle together, you can't afford to have a false positive, too many of them. You can't afford to give alarms when alarms shouldn't be given. You can't of course have false negatives. How do you put these puzzle pieces together to really help? Computer science is only a very small part of these problems. It's really working with geologists, civil engineers, oceanographers, and so on.
ZIERLER: It also raises the question, of course, of funding. It's an enormous amount of infrastructure to get these sensors in place so that they're relevant and useful when we need them.
CHANDY: The funding typically doesn't go through computer science. Computer science often gets funding for just a tiny bit of the basic computing behind it. Most of the funding goes through like civil engineers or seismologists or in this case people working on radiation. I think that's appropriate. That's appropriate to have a large amount of funds go to the actual infrastructure, and a small amount go to the basic algorithms that underlie it. To the extent that these problems become more visible to society—at some point, radiation was very much a concern—the funding follows.
ZIERLER: Finally, your work on electrical power systems, we already touched on this in our discussion about the cloud and renewable energy. My question there is, what aspects of this research are relevant for those endemic problems, ongoing issues about renewable energy and energy efficiency and things like that, and what aspects of this research are more on the crisis management things, about the threats of solar flares or terrorist attacks to the power grid and things like that? How is this technology relevant in both realms?
CHANDY: This work was done with Professor Stephen Low. You should probably talk to Stephen because he's a fascinating person. This wasn't work that I initiated; it's work that I joined, and I joined because again, some of the mathematics was really fascinating. The work started off with understanding so-called OPF, Optimum Path Flow. So you have a network, the grid, there are places where energy is generated—the wind farms, coal, nuclear—and there are places where energy is demanded—homes, factories. The path flow problem is what's the best way to get power generated and supplied to where it's demanded so that the supply has to match demand second by second. It's not a case where you have enough batteries where I can supply a lot of energy for you, and you can store it and use it whenever. Supply and demand match instant by instant throughout the day. So what's the optimum structure for part of—what economically and in terms of green energy to generate—where should power be generated and how should it flow to get to its destination? Stephen and the group were working on the mathematics of that, and so I helped on that. That has absolutely nothing to do with crisis management. It's just about this OPF problem. The OPF problem is related to green energy because optimally, you would try to use those sources of green energy that are available at that instant. Then we also explored the issue of storage, and what difference would energy storage make to the economics of energy production. How much of it would be produced. That was one class of work.
There was another paper, another project that we worked on with Southern California. Edison supported some of our work, and we had a strong relationship, Caltech did, with Southern California Edison. This is really more of an economics problem. If you look at your energy bill, the price you pay depends on how much you consume. It's like the tax system; the more you consume, the higher your incremental cost, for kilowatt hours. It's a convex function. If you have a large house, it's in your best interest to get rooftop solar to move your point of consumption from the place where the slope is high, down to where the slope is low. There are so called different tiers, so you come down to the low tier.
When you do that, the energy company loses a lot of money, because they make most of their money when the slope is high, when they get more dollars per incremental power supplied. So they're losing money, but they are required by law to provide energy to the whole community. When their income goes down, they're allowed to raise rates. But they're losing the most valuable customers, who are switching to solar. Not switching entirely to solar, but just enough to come down on the tier. So, there was this notion of so-called spiral of death, meaning that everything would have to collapse, because prices would keep increasing for the lowest tier, because people could afford to move to the lowest tier, and the goal of the lowest tier was to protect the poorest people.
We did some work on what actually happens inside California, which areas are developing solar, how much of it. Could there be a spiral of death? What could be done about it? And so on. That's not computer science, but one of my students was interested in solar, so we just explored that. Surprisingly, that paper has also received some attention, not in computer science but in policy.
ZIERLER: Last question for today. Of course, for all of this research, you have to write about it. You have to convey it. The question there is, when is it most efficacious to write an article, and when are you motivated to write a book?
CHANDY: The book is almost always for pedagogical purposes. Almost always. The first step is an article that gets peer reviewed. I'd say that's the way to do it. You first get peer reviewed, and then the book is a compendium of ideas. I think all through computer science, it's always the article first. Often it's a conference because things are happening so quickly it is often a conference first. That's even more important than the final paper.
ZIERLER: We'll pick up next time. We'll go all the way back to the beginning of your family background and your childhood.
[End of Recording]
ZIERLER: This is David Zierler, Director of the Caltech Heritage Project. It's Monday, October 18th, 2021. It is my great pleasure to back with Professor Mani Chandy. Mani, it's great to see you again. Thank you for joining me.
CHANDY: My pleasure.
ZIERLER: In our first two discussions, we took a wide-angle look at your approach to computer science and your interests in research and teaching. Today, what I'd like to do is go all the way back to the beginning for your personal history and family background. Let's start with your parents. Tell me about them and where they're from.
CHANDY: My parents, they were born in the state of Kerala, in India. The state is unusual in having women have much more authority than just about anywhere else in the rest of India. For various reasons women have always been treated as, perhaps not equal, but almost equal. It's also a state with the highest education levels. My father studied economics and law, went to England, and was called to the bar in England. Then he came back. He was very active in the Indian independence movement. At that time, India was a British colony. He was active in the so-called Quit India Movement, both while he was in England and after he returned. Then he joined a British firm, it's called—here it would be called Lever's. In India, it was called Hindustan Lever's. They make all kinds of things—oils, soaps, and so on. And in particular—the oil that most households use for cooking. He did that for a while. He was a director of the company. We could see poverty all around us, but we were really, really well-off, even by American standards. We were very lucky that way. Then he decided to serve the country again, so he started something called the Food Corporation of India. He started the first so-called IIM, Indian Institute of Management, which is now really famous, it's called the IIT. He was also the chairman of another Fortune 500—it's called the Hindustan Steel Corporation, one of the steel corporations in India. So that's what he was.
My mother got her degree in Sanskrit, raised five kids. It was sort of a British tradition to send your kids off to boarding school at the age of eight, so we were shipped off to what was at that time the remnants of a British boarding school, a so-called public school, what the British would call public school, which is really a private school. It was far away from home, and you got shipped off at eight. It was sort of a military school, a lot of very British traditions. We used to wake up to the sound of a bugle, go to sleep with the sound of a bugle, and we had military dress, that sort of thing. My brothers all went to the same school. My sisters didn't. After school, I went to the IIT, and from the IIT, I went to NYU. That's a brief history.
ZIERLER: We learned a little from your name that your family background has—would it be Christian roots?
CHANDY: Yes, yes. The story is that St. Thomas came to India, and apparently his body is buried in a place called St. Thomas Mount. He came and he converted people. Because he came from Palestine, that area, people in India who he converted are called Syrian Christians. Because way back, that's where they came from. We're not really Syrian at all. That's where the priests originally came from. Even today, there's a very thriving Orthodox Christian community, Orthodox like the Armenians or the Greeks or the Russians, so the same sort of heritage. It's a very small community, so it's a minority, in India.
ZIERLER: Is it a minority in Kerala, as well?
CHANDY: No, in Kerala, it's not a—it is a minority. I'd say it's like 25% of the state. The state is very multiethnic, multireligious. I think it's about 25% Christian, 25% Muslim, and maybe 50% Hindu. That's again one of these anomalies, in that they all got along well for centuries, probably because people came—they came there as part of trade, rather than warfare. We also had a large, at one time, significant Jewish community, some I think as way back as the first diaspora, but then later. It's partly because the trade winds blow back and forth very conveniently from the Middle East. It's a center for spice. A lot of the spice that the Europeans were looking for—Vasco de Gama and so on—they came to Kerala, because it's the spice center. So we had a lot of Middle Eastern interactions. We had Jewish, Muslim, Christian communities for many centuries. We have a Jewish synagogue. And there has never been, ever, any sort of animosity towards anybody.
ZIERLER: What kinds of religious traditions did you have in your household growing up?
CHANDY: The Orthodox church is very, very Orthodox. I don't know if you've been to or seen a Russian Orthodox sermon. They typically tend to be very, very long, for one. The rituals are very important, much more important—rituals—than I think even in the Catholic Church today. So there was that. When I went to the boarding school, the church we went to was at one point it had been called the Church of England, but by that time it was called the Church of South India. It was very sort of English. We had some English pastors. It was a protestant church; it wasn't the Orthodox Church, just because it was just more convenient. It was compulsory. You had no option. You had to go to church, and you had to be dressed up in your Sunday best. There was a Sunday uniform, very formal military uniform, serge blue with red stripes. You woke up, marched off to church, and marched back. [laughs] But it was nice. It was compulsory, but nice.
ZIERLER: What did Christmas look like in your house when you were a young boy?
CHANDY: Christmas was, for us, fairly Western, I'd say. The tradition in the Orthodox church is that Easter is the big day. Christmas isn't that big a day.
ZIERLER: By Western, did that mean that you had a tree and opened gifts?
CHANDY: Yes. [laughs] We had a tree. We had a Santa Claus. We'd open gifts. Yeah, all that. Sometimes there were other traditions. English traditions were to put a coin inside of a cake, one or two coins, and the lucky kid would get the coin. I think it has all changed. I think India has now become very, very Western. I think everybody celebrates Christmas. But it wasn't that way in the 1950s.
ZIERLER: You said everybody got along. Even if Hindus were dominant demographically, that did not mean that they were dominant necessarily socioeconomically or politically?
CHANDY: No, and there was—what's the word—religion was—there's a word for it, in the sense that it was integrated. A Hindu person might go to a Hindu temple as well as a Christian church. You wouldn't think that that was wrong. It was all part of—spiritual. And vice versa, Christians would go to a Hindu pilgrimage. It's only lately, lately meaning like—at some point, people decided that this was not pure. It wasn't pure. Then there was some reaction to trying to purify. It's perhaps purer than it used to be, but even now, people are very tolerant who are there. It's not in the rest of the country. As you know, there has been a lot of religious tension that has been stoked, even since 1970. Luckily, not so far in Kerala.
ZIERLER: What language or languages were spoken in your household?
CHANDY: The language of Kerala is called Malayalam. My parents spoke Malayalam. However, because they were both educated in English—the medium of instruction in their schools and colleges were in English—I would say that most of the time, my parents spoke to each other in English. Maybe 60% of the time in English, 40% of the time in Malayalam. I think the vocabulary was much better in English.
ZIERLER: For you, what would you say your mother tongue was?
CHANDY: I didn't know Malayalam. I had to learn it afterwards, so I went to boarding school. They sent me to a private tutor to learn my mother tongue, the language. I don't know if mother tongue is the right word, but the language that we spoke from the age of one was English. We learned English nursery rhymes, English hymns, and so forth.
ZIERLER: You would have been too young to remember, but what was your family's experience during World War II?
CHANDY: Ah! So my father was—there is a story of him returning from England. He was called to the bar, returning from England, and he was coming through—just past the Suez Canal, I think. I've forgotten exactly where in the ocean—on a ship, of course. And there were German bombers strafing the ship. And if I remember right Lady Mountbatten, who was the wife of Lord Mountbatten who later become viceroy—there were a bunch of English kids being sent off to India and places that were safer, and he says he remembers—my father says he remembers very clearly that she got all the kids to come together and hold hands and sing a hymn, while the bombers were coming, strafing the plane. So he got to India by the time the war started. In India, he himself didn't have any direct role in the War. My mother's brother was a lieutenant in the—I guess they were called the British-Indian Army, fighting in Burma. But most people in India didn't really fight in the war itself. There were some people who fought, mostly in the Burmese theater.
ZIERLER: You would have been very young, but do you have any memories of Partition and the dislocations that it caused?
CHANDY: No, I don't have any memories of Partition. At that time, we were living in Bombay, which did have problems. I don't know if there were massacres, but there clearly were Muslim-Hindu problems in Bombay, and much less so in Kerala. In Bombay, there were. But I myself, I don't remember any of that.
ZIERLER: What were your family's politics, in all of the debates that were happening in India at this time?
CHANDY: They really, really believed in Indian democracy. My father was—and again in England, there were some of the leaders of the Quit India Movement—Krishna Menon was the defense minister at one point. who was chief secretary—lots of people who were really big shots in India later on. They truly believed in an Indian democracy and Indian people. For example, he was quite shocked and upset when for a brief period, [called a National Emergency], democracy was put on hold. So he was really believed, I mean, truly, truly believed in democracy, that somehow democracy would make things work.
Generally speaking, they were what you would call—here you'd call it socialists, who believed—then, and even now, there's a big distinction between the well-off and the many that are not. As you know, there was a caste system—there is a caste system—and they believed it was appropriate for the government to deal with that by going out of its way to help people of lower castes. That's what I'd call their politics.
My father, after he left private enterprise, he started the Food Corporation of India, which at one point was very critical in keeping starvation away from many people. And the Hindustan Steel, which is the largest steel corporation. It's owned by the government. So he clearly believed that the government had a role to play. When he started the IIM, the Institute of Management, that was done with MIT. It was jointly MIT and IIT. So he had a lot of friends from MIT, professors from MIT. So he had a lot of American friends as well. But I think overall, politics would be first emphasizing democracy, and secondly emphasizing the need for the state to uplift the downtrodden.
ZIERLER: Did you stay at boarding school for the rest of your primary education, all the way until college?
CHANDY: Yes. That was the norm, for a certain class of people, I guess.
ZIERLER: Where was the boarding school? How far away was it from your home?
CHANDY: [laughs] It was far away. These were boarding schools built by the British, for the British, and it was very, very British. It was up in the hills, so it was cool, about 7,500 feet high. The weather was very nice. There was something called the downs, just like an English downs—very pretty, very green. It was far away, though. It was in a place called Nilgiri, so the Blue Mountains. At that time, we didn't really have the ability to call home. Phone calls were expensive. No cell phones, so communication was once a week we sent a letter home and got a letter from them. So it really was being sent away. It's not a good thing to do. I wouldn't do it again, to my kids.
ZIERLER: What about for the summers? Were you home in the summers?
CHANDY: Yes, yes, we'd go home in the summers, and sometimes we went to my grandparents.
ZIERLER: What were your interests, your hobbies, your academic inclinations when you were growing up?
CHANDY: Well, in a military boarding school, it's pretty regimented. You don't really have very much in the way of options. You all had to play sports. Sports were very, very important. There were different seasons. There was a soccer season, a hockey season, cricket season. You played whatever was in that season. I liked math, but the school didn't really emphasize academics particularly. I think things have changed for the better. I think the remnants of unfortunate British traditions had carried over. But I liked math, and school didn't particularly encourage people to go off and do their own interests.
ZIERLER: Do you think that regimentation served you well?
CHANDY: [laughs] It made me hate regimentation.
ZIERLER: [laughs]
CHANDY: Many of my classmates did go into the military—Navy, Air Force, Army—and did very well. Because it really was a military school. You'd walk around with rifles. It turned me against it.
ZIERLER: What were your favorite academic subjects in middle school and in high school?
CHANDY: I liked everything actually. There was almost nothing I didn't like. I liked science. I had really good teachers. Good physics teachers. I liked academics. I liked English. We had very good English teachers, too. We read a lot of Shakespeare. We read a lot of poetry. I could have gone off in any direction because I liked everything. But I particularly liked math. That's what got me into the IIT.
ZIERLER: Tell me about some of the options that you had when you were considering colleges. First of all, was leaving India an option? Did you think about going to the United States or England for college?
CHANDY: Not at that point. I finished high school when I just turned 15, so really quite young.
ZIERLER: Had you skipped grades?
CHANDY: I had skipped one grade, but I started early. At 15, to be going abroad would have been too much, so I didn't consider going abroad at all. The options were to do essentially engineering or law or medicine. Those were the typical options.
ZIERLER: This is modeled after the British system where you have to declare a specialty or a focus early on?
CHANDY: In the sense that you pick specific subjects that you want to focus on, for high school, for example. Then of the options of medicine, law, or engineering—pure science wasn't much of an option, because there weren't any jobs in pure science. Engineering was the nearest thing to pure science and mathematics, so I took engineering. I preferred that to law or medicine.
From Kerala to IIT
ZIERLER: Tell me about the exams to get in to IIT.
CHANDY: The exams at that time, the IITs were just beginning. When I went to Madras, I had an oral exam. We had to travel to Madras. At that time, it was started by a collaboration with West Germany. Germany was separated at that point. At least one half of the interviewers were German, and they would ask questions in math, and questions in engineering, and luckily I got in. It's much harder now, much more demanding.
ZIERLER: Is the IIT a place that would only accept people that were exceptional in high school?
CHANDY: Yes. You had to be exceptional in high school. Of course now there's this horrendous exam, so there's so much weeding out already that—to some extent, it doesn't really matter how well you do in the school, because there's so much selection already. You're pretty much safe getting anybody from an IIT, regardless of how well they've done in the IIT. You would know whether somebody got burnt out because the pressures are quite high.
ZIERLER: Tell me about when you first arrived at the IIT. What were your early impressions?
CHANDY: The IIT at that time was being built, so it was partly under construction. I guess we had a lot of German professors, because they were starting to build the university up. So many of our classes in physics, math, workshop, building stuff, was done by German professors. I think they came all the way to India because they wanted to make a difference, so they really worked at helping us. This is 1965, so this would be about 20 years after the war, and these professors would be in their 50s, so they must have been in the war. It's not the prototypical sort of "I'm superior to everybody else." The professors were very, very good. I really enjoyed it. We also had very good Indian faculty, because at that point, we didn't have a graduate school, so the really senior professors were teaching all of us, which was wonderful. It's like if Caltech didn't have a grad school. Senior professors do teach undergraduates at Caltech, but it's not covering the entire faculty just for the first few years of undergraduate. That was nice. The IIT was maybe the first time where I had students from a broader collection of the Indian population, than in school. School was filled with people who were fairly wealthy. It was sort of very homogenous, versus IIT was much more part of the general Indian culture. That was interesting, too. The medium of instruction all along was English.
ZIERLER: What was your curriculum like? What were some of the core classes that you had to take, and if you had opportunity for electives, what did you choose to take?
CHANDY: The core classes were not that different from Caltech. You had to do a lot of physics, chemistry. Not much biology, by the way. Physics, chemistry, and math. A lot of math and physics. And then unlike Caltech, there was two years of what's called workshop. You had to use a lathe and all that sort of thing. Then the third year, you could start selecting options. I chose something that the Germans called light current. There was heavy current and light current. Light current was electronics, transistors, making use of computers, so that was my choice. Again, because I liked math, and I thought that would get me closer to that. Again, some very good German professors in so called "light current" engineering. Again because I think the Institute was starting up, we had quote "research opportunities" which might be much harder to get now, because the senior faculty were very happy to have 19-year-olds working with them.
ZIERLER: Did the IIT have a computer on campus at that point?
CHANDY: The IIT did finally, at the end, have a computer. They had initially analog computers. There was one digital computer somewhere in Madras.
ZIERLER: What did it look like?
CHANDY: [laughs] It looked like a bunch of cathode ray tubes all collected together. It was quite big. The computing aspect was not that available to us and exciting so much as sort of the math behind it. So you could understand the math without doing a whole lot of programming. Everything changed, right?
ZIERLER: Did you have access to Fortran, or did you know what Fortran was?
CHANDY: Yes, I did know what Fortran was, but at that point, we didn't have much in the way of programming. I couldn't program much at that point. It was only after I got to the States that I got to program. But it was all Fortran, yes.
ZIERLER: Were you on a trajectory where you could have easily, with the engineering degree, entered into industry and stayed in India? Or were you always on a more academic path and you recognized that by necessity you would need to leave India to continue your studies?
CHANDY: I could have gone to industry, but I just liked academics, I guess. It was something I wanted to do. Not necessarily be a professor, but to keep exploring the math and science. I wanted to do research.
ZIERLER: Did your parents support this? Did your father ever hope that you would join him in his enterprises?
CHANDY: He hoped that I would come back to India and start an Indian research organization, but he certainly supported me as far as wanting to do research.
ZIERLER: What were you prospects when you graduated from the IIT? Where could you go? Where did you want to go?
CHANDY: Well, I got a scholarship at NYU. At that point, it was called Brooklyn Polytechnic. Again, I think we had some faculty from there in India, who knew about the IIT, who knew my professors and could see my courses. I got a scholarship there. I got admission to other places. I got admission to Stanford. But the scholarship made all the difference, and that's where I went.
ZIERLER: Did you have any family in New York or anywhere else in the States?
CHANDY: Yes, yes. Actually [laughs] it so happened that my other brother was at the same university. He hadn't gotten a scholarship, but he was there. So it was very, very convenient. I just moved into his apartment.
ZIERLER: What did you think of New York when you arrived?
CHANDY: This wasn't just New York; this was Bedford-Stuyvesant.
ZIERLER: [laughs]
CHANDY: Bedford-Stuyvesant in 1965. Which was two very different things. [laughs] But you're so excited about the university, and studying, and the students that you're with, that we didn't pay that much—I mean, we were aware of the milieu. Again, 1965 was a lot of student activity. There was clearly an issue with civil rights in Bedford-Stuyvesant. Our building had lots of African Americans. You could see the Archie Bunker mentality around. But I personally don't remember being discriminated against, either by Blacks or whites or anything else. It could be that we were just too innocent to know. But we got along, and I had some very close African American friends, and some very close older Jewish friends. In some sense, [laughs] that's the problem, if you will, with America, is that especially now, people assimilate so quickly. I don't know if assimilation is the right word, but you feel so much at home right away. I think if you didn't, you're more likely to go back. But if you feel at home and you start working, and work is exciting—so at least my experience has been very welcoming.
ZIERLER: Was this a terminal master's degree, or was it a PhD program and you left after the master's?
CHANDY: It was a PhD program. I left because I wasn't sure what I wanted to do next. I thought I might want to do biomedical—I was into trying to quote "help" make a difference for mankind, as opposed to doing science for science.
ZIERLER: What was the master's thesis on?
CHANDY: The master's thesis was on a certain kind of what's called automata. Trying to prove limits of what certain kinds of automata could do. It was esoteric, so I thought I'd try to do something closer to helping people directly, and that's where biomedicine came in.
ZIERLER: Even at that early point, you were thinking about the relation between automata and biology?
CHANDY: Not so much the biology. I wanted to do something where I could say I helped somebody. Sort of tangible, and disease of course was one slice. I went to Johns Hopkins biomedical program, PhD program, and there, they sent you to medical school for a year. I was at medical school with the other Johns Hopkins students, and I was a terrible medical student. First term was anatomy, and one of the things, we had to cut up a cadaver. It was too big a jump to go from thinking about math based on a small foundation, you're deriving everything from that foundation, to understanding how every different vein and muscle in the human body—I passed anatomy, I passed biochemistry, I passed physiology, but then I decided this was just not for me.
So then I went to work. I went to work at a place called Honeywell EDP, which was a computer place. For some reason, I got into a research program there. I only had a master's, but it was a research group. That got really exciting. For the first time, I worked with somebody on a paper, a research paper, that actually got published. This was Honeywell in Waltham, which is not far from MIT. Then I started going to MIT, to take a course or two. This was in operations research. I really liked that, so I then switched from Honeywell to MIT full time. I got my PhD in OR.
Early Computation at Honeywell and MIT
ZIERLER: Do you have a specific memory, either when you graduated from the master's or when you were working at Honeywell or when you decided to go to MIT, that you would be staying in the United States, you'd make a life and a career for yourself here, and not go back to India?
CHANDY: No, no. My parents were very, very patriotic, and I really thought I would go back. You'd feel terribly guilty if you didn't go back because that was the expectation. So for me and for many of my colleagues, it's more like you sort of fell into it, rather than made a specific decision to not go back. I got married, and my wife is Indian. We met in Mount Holyoke. Then you go to graduate school, and get your friends. I don't know if the word "accultured" is the right word to use—but perhaps multiculture; you get used to this environment. We were both avid hikers, so we were hiking all over New Hampshire. Before you know it, you get used to this kind of life. Hiking, running, being outdoors, sailing—these are different activities than I would have had in India. That's not the reason why I—you sort of fall into it. It's only after many, many, many years have gone that this comes about. I suspect that's the case with many people. Again, I don't know what it's like in other countries. I'm guessing—I have no idea—but if you go to Germany, it feels strange enough, or you're made to feel strange enough, that you would leave and want to go back. But maybe not in Canada or the U.S.
ZIERLER: Did you have access to cutting-edge technology and computation at Honeywell?
CHANDY: Yes. At Honeywell, and of course at MIT.
ZIERLER: What did the computers look like at Honeywell? What were you working on there?
CHANDY: Honeywell EDP was mostly building—they had gone from tabulation cards, tapes, to computers that processed them. It was primarily—EDP stands for electronic data processing, so supporting banks and things like that. It's a natural progression, as you know, from punch cards to computing. Those computers were physically big. We were just moving to the first tiny, tiny chips. People were talking about what would happen. This was before Moore's Law could project what would happen in the future, and that everything would shrink. But again, I think what I was telling you earlier was what's interesting is the basic ideas of programming from back then are still with us today.
ZIERLER: What was the interface between MIT and Honeywell? Were there professors who were doing consulting at Honeywell?
CHANDY: No, no. MIT allowed you to take courses, get credit for courses, in the graduate program, and Honeywell paid their employees to take courses anywhere, at least paid partially. I got part of my tuition paid when I went to MIT. I hadn't really thought of going to MIT full time; I just wanted to take some courses. But again, the courses were so much fun. They really were very well taught. I must say they were really well taught. It got very exciting, so I started to do that full-time.
ZIERLER: Was there a particular professor at MIT that you connected with, that made you jump into the program full time?
CHANDY: There was, Professor Drake. Alvin Drake. He taught probability theory. Of course now we would consider it fairly basic probability theory, but he taught it in such an exciting way, with exciting homework sets. Once you started with something, you wanted to learn more and more about it.
ZIERLER: What was the program? What department were you in? Was it electrical engineering with a focus on operations research?
CHANDY: No, MIT had these centers, so you joined a particular degree program but then you did all your work in the so-called center. There was a qualifying exam—two sets of exams, both written. There was one set of exams in EE, and a different set in operations research. So when I went to the OR program, I didn't do any of the EE exams. Their requirements were completely different. My degree is really in OR. EE was just a home to start from.
ZIERLER: How far back does the field of operations research go, from that point?
CHANDY: It really goes to World War II. Probability theory goes back to Pascal and beyond, of course. But as a science, as a separate field, I'd say it goes to World War II, and in particular to linear programming. Dantzig was one of the fathers of linear programming. They were used in World War II primarily I think for logistics. It made a huge difference for managing logistics. Then of course from linear programming it became mathematical programming, and optimization more generally. Again, that was very, very exciting.
ZIERLER: Did OR have connections with the defense establishment?
CHANDY: As MIT, you mean?
ZIERLER: At MIT.
CHANDY: MIT, I don't really know. MIT had its own lab but I didn't personally have any work with the Defense Department. My student colleagues did have work with civil organizations, looking at traffic, I think even crime reporting statistics, things like that. I can't think of any of my colleagues in class doing defense work at that time. It was also, remember, the height of the Vietnam War.
ZIERLER: This was certainly an interesting time to be in Cambridge, Boston. Were you politically involved at all?
CHANDY: Yes and no. Yes in the sense that I had strong opinions. These opinions were not different from I guess either most Indians, most students all around. It was a bad time for the U.S. So if you went to a northeastern college, you could see cops beating students and students harassing cops and things like that.
ZIERLER: You mentioned an Indian perspective. Was there a unique colonial lens that you understood events through?
CHANDY: I wouldn't say unique. There was a colonial lens, that the issue at heart was a colonial issue. It isn't that it was worldwide communism versus worldwide capitalism. It was more the remnants of antagonism towards French colonialism in Vietnam or British hegemony in India—there was a natural way of viewing things as a reaction to centuries of colonial rule. In that framework, we thought of it more in terms of many centuries of history of country or ethnicity, as opposed to the more recent global philosophical issues of capitalism versus communism.
ZIERLER: Who ended up being your graduate advisor at MIT?
CHANDY: Jeremy Shapiro, who was interested in optimization.
ZIERLER: How did you connect with him?
CHANDY: When I went to MIT after passing my exams, I took all his courses. I helped him teach a summer course. I could have worked with anybody, but I ended up working with him.
ZIERLER: What was your thesis on? How did you develop it?
CHANDY: The thesis was in dealing with very large optimization programs. This is partly computing, partly mathematics, because you had to break up a large problem into parts, where each of the parts was doing sort of local optimization, but then you ended up with a globally optimal problem. It was really hard to break up this big problem into parts and seeing what the interactions between the parts would be. There was a lot of interest in that sort of breaking up and putting back together, for large problems, at that time. Because computing wasn't that cheap, and so you had to do things efficiently.
ZIERLER: Did you see this research as responsive to your early interests in making something that would help people?
CHANDY: Not really. [laughs] It was just fun. I really liked it. It was fun. I had at least two goals, which are orthogonal as a way. There's a tension between the two goals, and I go back and forth between them. One goal is just to do science for the sake of science, just because it's fun. You also get recognitions in the science. The other goal, because of the way I was brought up, is you've got to make a difference to humanity, because humanity is suffering. You don't see that suffering in the U.S. right now, but 1940s, 1950s, India, there was a lot of suffering, and you felt guilty not trying to do anything about it. So there's this tension between doing science for the fun of it and trying to make a difference.
ZIERLER: What were some of the big questions in operations research at this time, and how did you see your dissertation as attempting to be responsive to those questions?
CHANDY: What was happening was, even back then—so this is 1969—problems are getting bigger and bigger and bigger, and problems are getting bigger faster than computers were growing, and computers are getting bigger, too.
ZIERLER: By problems, what do you mean? What does a problem look like?
CHANDY: The size of the problem. So doing an optimization problem. Before, you were looking at a small problem; now you're looking, for example, at American Airlines scheduling flights, and by the 1970s, there are huge numbers of flights. The problem size just got to be horrendously big. Even though the underlying mathematics would still apply, we didn't have the computing power to solve these big problems. Back then, one of the questions was how do you do it in a more intelligent way? That was one series of questions. A related question was, how can we use operations research to improve computing itself? The computer was a scarce resource. It no longer is. But back then, it was very much a scarce resource. It became a scarcer resource because the problems had become bigger. More people wanted their problems solved. The question was looking inwards, use OR for the computer itself. So we can use, for example, mathematical optimization techniques to optimize the computer itself. You can use queuing theory to improve queueing in the computer itself.
ZIERLER: When you mean improving the computer itself, do you mean at the hardware level or at the programming level?
CHANDY: At the operating systems level. To give you an example, there are many, many jobs that are submitted to a computer, many, many jobs going through. There's a scheduler that decides which one to do next, because there's only a few jobs that can be done at any given instant. You've got to choose among this large collection of jobs as to which ones to do next. That scheduler makes a big difference as to the overall speed, overall throughput, of the computer. This is all part of what's called the operating system. The hardware is fixed; the software is something else. But this is a system that operates the whole computer. You can make that more efficient, and using the same hardware, the same programs, just get better throughput.
ZIERLER: Was your appointment at the IBM Cambridge Scientific Center part of the PhD program, or that was separate?
CHANDY: It was separate. The Cambridge Scientific Center was inside the MIT building, so you just had to take an elevator down and get off on the other floor, and you were at IBM. I hadn't really thought things through. When I finished, we got married, and I had to get a job. My wife was going to school. In some ways, it was the easy thing to do—take an elevator down and go to IBM. They did have exciting work in this area, specifically looking at using operations research to improve computing and telephony.
ZIERLER: Who would have been some of the commercial clients at this early stage that would have valued these developments?
CHANDY: It was very, very widely useful. Everybody. Banks, insurance companies, IBM itself. Bell Labs. The telephone companies. It was a good time to be in this area. You could go anywhere you wanted. This was probably the best time to be in this area. You're in high demand. Things were early enough that you could come up with ideas that in retrospect seem trivial, but back then made a difference. Papers that I wrote in papers 1969 and 1970 that are still cited quite widely now, 60 years later. I don't think of them as breakthroughs in the sense of theory of everything. They were just simple things that happened to be early enough in the wave of progression that it happens to be useful for the entire wave. There are times in science where I think it's just luck, and there's a long wave, and you just happen to be at the start of it, and you do something trivial, and then it keeps getting referenced, because it was early. I think it was much easier to be quote "pioneering" than to be really smart. Because pioneering, you have to ask the right question, and the answer is often quite trivial. Just by having the right question, the question is repeated for decades, and they keep pointing back to you as the person who asked the question first, and got this trivial answer.
ZIERLER: And who was there at a formative time.
CHANDY: Yeah, yeah. But it's partly luck, I think, because the solutions get harder and harder and harder as you keep improving things. You improve it, and then improve it, and then the marginal improvement gets smaller and more difficult. I think it is much harder later in the life cycle of an idea than earlier. I think I was stupid but early enough that I was lucky.
ZIERLER: And well placed, of course. [laughs] By luck.
CHANDY: Well placed, very much so, yeah.
ZIERLER: Besides Shapiro, who else was on your thesis committee?
CHANDY: Shapiro, Kaufman, Drake are the people I remember. They were all in operations research, and they were actually in the Sloan School of Management. Drake was also in electrical engineering. That's, again, a nice thing about MIT, was they didn't—I got a degree in electrical engineering, but you worked with people in the Sloan School. I think that's important.
ZIERLER: The same question as when you were at IIT. Especially because OR was in such demand at this point, did you think about going into industry, even finance perhaps?
CHANDY: I did think about going into industry, again, going back to the tension between doing something fun and making a difference. I thought of going into consulting, not so much finance. It was sort of business consulting because my advisors were in the Sloan School. I would also perhaps have made more money. But there's again this tension between doing something useful in that sense, versus doing something fun. This was so much fun, and as I said, I was lucky to be in the beginning of this wave, that I decided to stay on.
ZIERLER: Tell me about the opportunities you had looking for academic positions.
CHANDY: Again, because of that period in time, I think we were just lucky. It's much harder to get academic positions now than it was back then, certainly in computer science. It was much, much easier then. One of my bosses, one of the people I worked with at Honeywell, had gone to the University of Texas as the chairman of the department in Austin. He invited me to come down to Austin to stay with him and do some work with him. I remember my wife and I leaving Logan—it was March, in the sleeting rain in Logan when we got onto the plane. We got to Austin, and stayed with him, and it was sunny and lovely. Springtime in Austin is lovely. When we got back to MIT, it was sleeting rain at Logan again.
ZIERLER: [laughs]
CHANDY: I had known him really well. He was a good friend of mine. Much more senior than I was. So it was sort of natural to go to UT.
ZIERLER: Tell me about the Department at that time.
CHANDY: The Department at that time was very, very small. It just started. The Computer Science Department had just started and it had grown from mathematics and electrical engineering. Again, it's impressive that UT started it fairly early, that a state school decided to do something new, before everybody else, before most other people had. That was a good thing. It was a very small department. It was a very exciting department. It was again a really good—you had lots of time to do research. We had no administrative responsibilities at all. I enjoyed teaching. There was also a lot of consulting and ability to work with industry in Austin. So we did quite a bit of work with some of the oil companies in operations research. Austin was a wonderful, wonderful place. Of course in 1970, the town was maybe 250,000, a quarter million, and now it must three or four million? It has really changed. It was a good time to be there. It was very much a university town, university culture. Now it has become very much a tech town.
ZIERLER: How old was the Computer Science Department at that point when you joined?
CHANDY: I think three years old.
ZIERLER: Oh, wow.
CHANDY: Three or two.
ZIERLER: So it was in a state of growth at that point, hiring new faculty.
CHANDY: Yes, yes.
ZIERLER: Were there other OR programs that you could have applied to? Did you specifically want to be in a computer science program?
CHANDY: Yes, I did. I did want to be in a computer science program because I had used OR for computer science, and that turned out to be really exciting. So I did want to be in a computer science program. There was the possibility of going to UCLA, but that was in OR, and I wanted to be in CS.
ZIERLER: Why was CS more palatable for you?
CHANDY: Because I could see the application. There's again part of the tension between theory and practice, is there's a theory, like queueing theory, but you can see it in operation in the computer. You can see it making a difference in the computer. You can see how it makes a difference in how Mobil Oil—some of its activities. It's a marriage of both theory and tangible, visual impact. That's part of one of the reasons. I had done some work in CS already, so it was natural to stay on there. It's not like I gave up operations research. I just started using it more for this purpose of designing computers better.
ZIERLER: What were the big questions that you were interested in at this point? What did you want to do to answer those questions?
CHANDY: I'm not being unduly modest. At that time, working on a problem, there was something exciting about it. It's almost like I stumbled on things, as opposed to asking the big question. Sometimes I asked a question; sometimes I stumbled on things. A lot of the early work that's cited today, that got me some recognition, was things I stumbled on. I was working on teaching a class on probability and queuing, and there were these networks of queues, very large networks, and people couldn't analyze them easily. I stumbled on a way of thinking about it which made it easier to analyze. Then it became useful in larger computer systems. I'd like to say that I sat down and thought about the issues at hand and said "This is a problem to solve" and then solved it but it really worked the other way around. I was futzing around with some interesting mathematics in teaching, and it sort of fell into place and I saw a pattern that eventually led to something useful. It was almost backwards. Yeah, it was backwards.
I got tenure very early. After three years, I became associate professor. At that point, I started thinking about what are important philosophical questions, what are important questions to solve, and what can we do about it. I don't know whether most assistant professors do work on their thesis and keep going and happen to do something that turns out to be useful. When they get a little older, they step back and sort of come up with a strategic plan. Maybe even when you're young, you're just going from continuing your thesis to strategic planning.
The Value of Philosophy in Computer Science
ZIERLER: At this point in your career, 1972, 1973, you're writing about trees, minimum spanning tree, teleprocessing tree network. I wonder if you can explain what tree means in this context, and if you would put this research on the earlier side of your transformation or on the later side, when you start to think about more philosophical concepts.
CHANDY: It was earlier. When I worked at IBM, we had a really fascinating problem. IBM had a huge telephone network, connecting all the IBM sites. It was huge, and they paid a bill to at that time Bell-AT&T. There was a mathematical problem as to how do you connect things, so as to reduce the bill and get the same efficiency. A lot of their problem was if you had a big center, then you had multiple locations connecting to that center, your connection is what's called a tree. You can think of the center as being the central, the root if you will, and then branches going all from the root. This structure is called a tree. At the leaves of the tree, you have computers trying to connect, communicate with the center. There's communication going from the leaves to the root and back to the leaves. The problems turned out to be very, very difficult to solve exactly. You could solve it approximately. That's where this problem—it came up from IBM. I worked at IBM. The abstract question was, why was that struggle so hard? That's when we started looking at so-called capacitated spanning tree. It turns out to be one of these problems that nobody knows how to solve, fast. You can solve it, but you can't solve it fast.
ZIERLER: What does solving slow versus solving fast mean? What does that look like?
CHANDY: Solving fast is what's called solving polynomially, meaning that if the x axis is the size of the problem, and the y axis is the time it takes to solve it, then solving fast would be where you could solve larger problems in polynomial time. If you double the size of the problem, then the time taken to solve it might go four times as much or eight times as much, in a polynomial way. You triple it—so you can essentially draw a polynomial like x2 or x3 or something. Solving slow is when it goes up exponentially, so instead of x2, it would be 2x. So if the problem becomes ten times as large, 210, which is 1,024, much larger. There's this fundamental question of, as a problem gets larger, does the time taken to solve it increase only polynomially, or does it increase exponentially? This problem is one of those that seems to be increasing exponentially. That's what solving slow and solving fast means. I really need to get a little board and draw to explain it, but you get the idea.
ZIERLER: Yeah. Does this lead to those deeper philosophical questions, though, in terms of historicizing this transformation of the things that were interesting to you?
CHANDY: This is a question I couldn't solve, but this then—this was an example of applying operations research to a computing and a communications problem. Because in the abstract, when you abstract this problem, it's a mathematical optimization problem, but the problem comes from very practical billing questions. The philosophical question, if you want to frame it that way, is coming up with abstractions. From very practical problems, coming up with abstractions. There's no mechanism for creating abstractions, but is there a mindset, a way of teaching, a way of thinking, that we can help people create the right abstractions from what appear to be a plethora of different problems? I don't think there is a way to teach—well, maybe there is. Maybe the way you teach it is more by example. There's no root algorithm to do that.
ZIERLER: Did you take on graduate students right away when you got to Austin?
CHANDY: Oh, yes. They were often older than I was, because I got to Austin when I was 25, and my first graduate students I think were in their thirties, even. It was again very nice, working with the graduate students and advising them. Many of them have done very, very well. Because they're often my age, many of the other ones have retired.
ZIERLER: In terms of the kinds of graduate students that were attracted to you, did they mostly want to pursue academic positions, or even at this early stage, a PhD in computer science was a great entre into the tech industry?
CHANDY: Oh, definitely. The tech industry, especially with a mathematical basis but which was very practical. They could see the tangible benefits very quickly. There was lots of demand for consulting. I did quite a bit of consulting myself. So students could go into industry or academia, even back then. The job market was terrific.
ZIERLER: Mani, I'm trying to divine from your publications where we might see this philosophical transition taking place. Perhaps it's over queuing, queueing networks, queueing problems?
CHANDY: I thought that certainly by the early 1970s that the queueing issues had been solved.
ZIERLER: What were the queueing issues?
CHANDY: In the sense of applying queueing theory to understanding a network of queues and how they applied to computing systems and communications systems, those problems had been solved. It was time to think about something else. That's when I thought about things like distributed systems and started asking fundamental questions. I wasn't very old back then. I'd say late 1970s, when I was late thirties. Things were secure. I think by then I was a full professor. I could do whatever I wanted to. It was fun to ask basic questions at that time.
ZIERLER: What is the intellectual bridge from queueing networking models to distributed systems?
CHANDY: There really isn't a natural bridge. It was almost a break. It's tough in the sense that you have a reputation in one area, and you know the mathematics in that area. You have a reputation. People know you, so you get invited to give talks, to start conferences and so on. It's easy to keep continuing in that. But then I decided to do something completely different where I had no reputation at all, so you really start very much at the bottom. "Who is this person and why is he doing this?"
ZIERLER: Is that to suggest that at this stage, the late 1970s, early 1980s, distributed systems was mature enough that it had a bottom to start at? Or was everyone at the bottom?
CHANDY: It had quite a few people working in it already. I certainly wouldn't be the pioneer. Whereas I think for applying queueing theory to computing systems, I'd be one of the handful of pioneers, less than five. But now there were tens of people, not hundreds. Not very large, but there were enough people that I could start work. The way I did that transition was to look at distributed simulation. I asked the question, "Can you do a simulation with multiple agents as opposed to a single agent?" Multiple clocks. That got me thinking more generally about distributed systems, but it's an interesting larger question. So here's the question, and I don't know how we'd answer it today: assuming that you have an established reputation in one areas, and let's say you're mid-forties or something, sort of in the top of one area, should you attempt to do something totally different, starting at the bottom? It's not clear to me that today I would recommend that, because I think it's much harder, because the fields are more mature. But back then, first of all, you had the security to do that. That's one nice thing about tenure. You can do something that would be considered stupid, starting from scratch. Whereas supposing you didn't have tenure, you'd worry about that, maybe, if you don't have papers for a while but you're learning something new. This is sort of a much larger question for academia in general.
ZIERLER: But for you, you saw tenure as providing security, that you could be adventurous in your research?
CHANDY: Yeah. Tenure, reputation, self-confidence. [laughs] When you're that young, you have a lot of self-confidence. You think you can do anything. That essentially gives you the courage to say, "I'm going to burn my bridges and do something different."
ZIERLER: What did distributed systems need, and in your youthful confidence, what did you think you had to offer it?
CHANDY: I thought I could ask interesting questions that I didn't know the answers to. But I could ask interesting questions. I had a collaborator in Austin, Jay Misra, so I could ask him questions. He could ask me questions. We started answering questions. The questions themselves were worth exploring, and people started getting interested in the early questioning.
I became the chair at Austin, and we hired then this really famous computer scientist called Dijkstra. He was one of the very first Turing Award winners. He was very interested in distributed systems questions, so we would have lunch a couple times and just talk about questions. That was also very helpful. I was making a break, and there were people I could talk to, in this new area that I was trying to shove into. That, I think, might be difficult to transition to a new area, if there's nobody there to work with. Again, I happened to live in the good old days, good times. I think it's much, much harder now.
ZIERLER: How interdisciplinary was CS at the University of Texas? Would you have reason or opportunity to talk with people in the Math Department, in EE, in Physics? Or was that not really viable or even productive?
CHANDY: It wasn't really productive. CS at UT was somewhat—I don't want to say isolated, but it was a department—you worked in CS. There were some people who worked with physics in computational science, but it was mostly a CS-centric department. CS professors often thought they were the pinnacle of what was important, and everything else was much less so. Looking back on it, it was stupid, thinking that way, but I think a lot of us did. Maybe you could even make an argument that in a certain stage in life, that that's what you want to be. You want to think that what you're doing is more important than anybody else's. As you get older, of course you realize that isn't true. I think there are stages in scientific life, scientific careers, where you have different mindsets.
ZIERLER: You mentioned that you were interested in asking fundamental questions. What were they?
CHANDY: The questions for distributed systems that I kept coming back to was to get many, many concurrent agents, each with their own plan or goal to work collectively to advance a common goal. How do you think about this? How do you formalize it? How do you see it in different settings? And what happens when some agents are malicious? How many malicious agents can you handle and yet achieve a common goal? These are all questions that we asked in an anthropomorphic way, and can invest in these digital agents, where human capability, malice, knowledge, but then frame the problem so that's just an agent problem. You think about like malicious agents; you'd say it seems fun, but nothing to do with reality. Of course it does apply to reality because a malicious agent represents a system that just goes awry and might do anything. The abstract question is how do you get collections of agents to do interesting things. You can keep asking many such questions.
ZIERLER: For you at this point, the questions are more interesting than the answers?
CHANDY: [laughs] In some sense, yes. But the answers are fun, too. I keep working on the answers. When I teach, that's what I try to teach. It's not so much, "Here is an algorithm," but here is the question that motivated the algorithm. Here's a question about how do you get these agents to do something together; then the algorithm sort of comes through. I'm sure the students will forget the algorithm, however we do hope ten years from now, 20 years from now, they'll think about the questioning process.
ZIERLER: Was the personal computer revolution in the 1980s, did that make you rethink how distributed systems worked, or did it just make everything larger, more expansive?
CHANDY: It made the whole problem much more important. It made distributed systems become one of the really exciting areas to be in. Distributed systems, concurrent systems—essentially having lots of computers work together. It just wasn't an issue in the 1970s, but it became an issue in the 1980s.
ZIERLER: That's to say that even before the advent of home internet, that computers that were really not networked could still be thought of as part of the distributed system?
CHANDY: Yeah, because in the big organizations, they had lots of computers working together. They weren't distributed in the sense that they were often in the same building but they had massive operations. There really were multiple computer systems. So the problem of having many, many computers work together is a fairly old one. It didn't become ubiquitous until later. But some organizations were concerned about it way back.
ZIERLER: The titles of your publications, as to be expected, are highly technical and somewhat dry. Yet one jumps out at me: "The Drinking Philosophers Problem." That's one that I'd like to know more about.
CHANDY: [laughs] Computer scientists have a record of poor jokes, I guess. There's a collection of problems. There's the drinking philosophers problem, the dining philosophers problem, byzantine generals problem. There's something called cheating husbands problem [laughs] where we give sort of interesting anthropomorphic names to very dry subjects. I'll describe the problem and then tell you where the name comes from.
Before us, there was Dijkstra, who came up with the dining philosophers problem. He was trying to explain, in a simple way, the issue with agents collaborating, so you could explain this to anybody. He came up with the idea of having five philosophers sitting around a pentagonal table, and they each have a chopstick on each hand. There's a total of five chopsticks, because each chopstick is shared by the philosopher and his neighbor. I'm sharing the chopstick on my right with my philosopher. For him, this chopstick is a chopstick on his left. Now, to eat, I need two chopsticks, so while I'm eating, my neighbors can't eat, because I've got both chopsticks. The one on my left doesn't have the right one; the one on the right doesn't have the left one. The goal for collaboration is that every philosopher gets to eat, eventually. He came up with the idea of a life cycle of philosopher. A philosopher is either thinking—they think forever—or he gets hungry. If he gets hungry, he transitions to eating, only if he gets more forks. The problem is that if all the philosophers get hungry at the same time, and you can only grab one fork, then you'll never get the other one, and they'll starve. So it's to prevent starvation. This overall problem of collaboration got put in these anthropomorphic terms as the dining philosophers problem. It became very famous. It's a cute problem, but underlying it is a very important, very difficult practical problem. But it's something I can explain to a five-year-old. I could even pull out my forks and show him what happens.
The drinking philosophers problem is more practical. The idea was [laughs]—dining philosophers—that a philosopher, an academic for example, is either tranquil, and tranquil forever, or gets thirsty, and you get thirsty for a specific set of drinks, so you want a gin and tonic, or a brandy and cream, or something. Only one person can be drinking gin at a time, so the gin represents a file, a file to which you need exclusive access. So a program needs exclusive access to a file; it's like a philosopher wanting to drink gin, or some other specific drink.
The problem becomes, how can all the philosophers collaborate so they all get to drink what they want to drink, and get to be tranquil if they want to be tranquil, without anybody getting dehydrated, remaining thirsty forever. The underlying problem is very much a distributed operating systems file management problem. So it has nothing to do with philosophers, and really nothing to do with drinking. It's part of a long—I don't know if it's a good history, or a bad history—of computer scientists coming up with cute names, anthropomorphic names, for very dry problems. A problem that I didn't come up with is the byzantine generals, which was initially called the Albanian generals. It was changed for politically correct reasons. Generals are traitorous and all that. So a bunch of cute names.
ZIERLER: Your work on heuristic algorithms, just knowing what heuristic means, is this an early version of AI, where you're looking at algorithms and how they can teach themselves?
CHANDY: No, I wish it were an early version of AI. It's not. For many of these problems, like the capacitated spanning tree problem, that are very practical, nobody knows how to solve them fast. There do not exist fast solutions, but they're very practical, and they're important problems to be solved. Then the question is, if you can't prove that they're solved fast, can you get an approximation that is solved fast? That's where the heuristics come in. The heuristics were generated by me, by humans. What you're talking about is sort of the next step, where the algorithm learns its own heuristics. That's not something we did.
Again, I think partly it's because today, you can do a lot of AI just because computing is free. You can spend a lot of time learning, which we couldn't do before, just because computing was so expensive. You had to teach, as opposed to having it learn by itself. On a separate note, this is completely different story about my work, there's a semi-philosophical magazine called Noema—N-O-E-M-A—and the last issue raised this question, about is AI helping or hurting the planet. Its potential for helping the planet was to make everything sustainable with machines, everything can be sustainable. And how it's hurting the planet is because we all expect computing to be free, which it is, pretty much. But again, getting back to the commons, what's really happening is that what we think is free is really not, because of the energy consumption. Have a look at Noema; it's an interesting question. It's a larger question than computer science, whether the commons—the commons in this case being energy—is not being seen by all of those who use information technology.
ZIERLER: On the administrative side, did you enjoy your time as department chair at Austin?
CHANDY: No. No, I didn't. It was something we had to do, and I think I did a good job. It was an interesting time, because that was the time at which the government of the state of Texas, and some very rich industrialists, decided that the state was too dependent on oil—a lot of its income was oil-based—and it had to switch to technology. I got to know the governor, and he actually helped to get the department to grow. He helped to get technology to come to Austin. It's not that my role was different, but I happened to play a role in this transition, when Austin went from primarily government academia to a tech town, in the late 1970s. It was always industrial policy. It didn't just happen. The government helped make it happen.
ZIERLER: Was Michael Dell a player in this transition?
CHANDY: A little later, but he was a player in this transition. There was as big IBM operation. We started something called the Semiconductor Center. Admiral Bobby Inman was the director of that. You tended to look down on, quote, industrial policy, especially about something [laughs]—the right wing people looked down on industrial policy. But this was in some sense industrial policy which was very successful. I was happy to be part of that.
ZIERLER: Speaking of industry, did you serve in a consulting capacity at all during your time at Austin?
CHANDY: Quite often, yes. There was a company called Boole & Babbage in Silicon Valley, and I did a lot of work for them. In fact, they started a branch for me just off campus in Austin. So yeah, I did a lot of consulting.
ZIERLER: Last question for today, and it will serve as a segue for our next talk, and that is, by the late 1980s, were you looking for new opportunities? Were you happy to stay at Austin for the rest of the your career? What were the circumstances leading to you thinking about joining the faculty at Caltech?
CHANDY: I was very happy about Austin. I had no complaints at all. I liked the place. I had very good collaborators, superb collaborators including Dijkstra and Misra. What changed was I had two kids. In India, it's very much a part of the extended family. You live in a clan, with parents and children living together in the same vicinity. Then I had two brothers in Southern California, with their kids. We felt isolated in this sort of family structure. That was the primary motivation for moving to Southern California. There of course you had to choose between all the universities in Southern California, and I loved Caltech, because of its multidisciplinary aspects.
ZIERLER: That's a great place to pick up for next time, starting life in Pasadena.
[End Recording]
ZIERLER: This is David Zierler, Director of the Caltech Heritage Project. It is Thursday, November 11th, 2021. It is my great pleasure to be back with Professor Mani Chandy. Mani, wonderful to see you again.
CHANDY: Likewise. Pleasure.
ZIERLER: Today we're going to pick up when you leave UT Austin and you join the faculty at Caltech. Lots of questions about the transition. I'd like to start first with your impressions of the teaching environment at Caltech. I know that teaching methods is something very important to you, and simply coming from an enormous school like UT Austin to a much smaller institute like Caltech, what were some of the differences that you perceived in terms of teaching Caltech students, the expectations of professors at Caltech, with regard to teaching? What were some of your earliest impressions?
CHANDY: One very positive impression was the Honor Code. It was really wonderful to give finals to the students and have them work at home. Sometimes we had quizzes that were timed so our students wouldn't spend too much time on a quiz. We'd say, "No more than three hours." And yet our students turn in exams where they stopped after three hours, almost like mid-sentence. That was really wonderful. It's not that students at UT were dishonest but it's nice to have an Honor Code.
The other point was that the classes were smaller, and we had many more graduate students taking classes with undergraduates, whereas in UT, they were quite separate. The students in both places were very sharp. I must say UT Austin had very good students as well. However, the variance of students at Caltech was smaller. They're all very, very good, and it was a pleasure to teach. I really enjoyed teaching.
ZIERLER: What did the Honor Code tell you about Caltech, about the students, about the culture of education?
CHANDY: That students were—I didn't think of them as being taught so much as learning with the faculty. It's not co-equal, but we're all in it together to understand something, as opposed to a professor lecturing. Especially with graduate students at that time, because there were so few, they were like young faculty. They were treated like the stars that they were going to become, so there was never any condescension. And you could do that, because again there were so few of them. That's what it told me.
ZIERLER: Did you bring graduate students with you from Texas?
CHANDY: No. I had planned to come for some time, and I'd come first as a Fairchild scholar. I had two years as a Fairchild scholar while I was debating whether to go back and forth, back to Austin. During that time, I finished my graduate students there.
ZIERLER: Were there funding sources that transferred with you, or how did that work in terms of setting up your research agenda once you got to Pasadena?
CHANDY: There weren't funding sources that transferred, but there were funding agencies from which I had received funds at UT. I went back to those agencies when I came to Caltech and got funded here. But it wasn't a transfer of funds; it was going back to the agencies and reapplying.
ZIERLER: In talking to professors who of course were at Caltech earlier than the late 1980s, early 1990s, many have cited this time as a period of transformation at Caltech where an entrepreneurial culture, where more interest in startups, where professors felt freer to become involved in business ventures was all happening around this time, whereas earlier, Caltech very much saw itself in a purely education or a basic science approach. I wonder if you detected that this was a transformation happening at the time you joined.
CHANDY: No. I'd say the transformation was much clearer later. I hadn't been in Caltech earlier, so I hadn't seen the transformation happening. Certainly, ten years later, say 1997 or 2000, you could see that entrepreneurial spirit very strongly, especially with the undergraduates, which is I think a wonderful thing.
ZIERLER: Because you were consulting in Austin, you were involved in the world of business. We talked about Michael Dell earlier, for example. In what ways before that 1997-2000 period, earlier, did you try to foster those changes that you cited?
CHANDY: I wouldn't say I tried to foster the changes. I had too much faith in our students. I really valued their judgment as opposed to mine, so I wouldn't try to encourage them one way or the other, because I trust their innate intellect. If students came by wanting to do an internship in the summer, I would certainly help them. Something I did do with my PhD students or potential PhD students is I'd talk to them at length before they'd embark on the whole PhD, about various options—options going into startups, options going into industry, and options staying in academia. During these discussions, three or four of the students decided that they were better off not doing a PhD, stopping with a master's, and joining, for example, Google or Facebook. In this case, Google. I think it was the right decision for them. Especially now, you want to do a PhD only if you're absolutely focused on research. I think in terms of the lifestyle and balance of life and work and so on, companies like Google do an excellent job. So you talk to a student and find out what are their life goals. Not to sound too pontifical. Of course what happened now is these opportunities in industry and startups, it helped more students go into that earlier without doing a PhD.
ZIERLER: In the late 1980s when you first joined Caltech, this is before of course the tech revolution that would come ten years later starting with Google, Apple, companies like that. Another broad question—we've touched on this a little before—this transformation where physics used to be the dominant major among undergraduates, and then fast forward 50 years later to the present, where that now dominant major is computer science, where was that transformation by the time you joined Caltech?
CHANDY: Oh, it had already happened. A lot of students interested in computer science. I'm not sure what the enrollment was at that time, but that transformation had already happened. You can see it more generally in engineering. There was a transformation towards the Division of Engineering. I don't know all the reasons for that. I think it's a good thing that engineering in general, especially computer science, has got so much airtime if you will, that students are more aware of it. The National Academy of Engineering for a long time was trying very hard to make engineering an exciting discipline, and they've done various things to do that. But at Caltech, anyhow, fairly early on it was a discipline of choice I think for most students.
ZIERLER: What was the driver of that? Before, again, the late 1990s, the tech boom of the late 1990s and early 2000s where there are these stories of undergraduates with these eye-popping sign-on bonuses that they would get in Silicon Valley. To the extent that that was not so much the case when you joined Caltech ten years earlier, was it economic considerations, or was it academic interest? What was driving student interest to make computer science the dominant field already at that point?
CHANDY: In general for engineering there was both academic and financial interest. Things like aerospace, the whole space program, JPL. The supercomputing revolution, the ability to simulate these huge physical systems. Supercomputers at Caltech—our computing [ability] available here. There was a ferment, there was an excitement about the kinds of things you could do with technology, and the rate at which technology was changing. The VLSI revolution probably started thanks in large part to Carver Mead. That was another exciting driver. So I think it was both academic ferment as well as financial incentives.
ZIERLER: Did your research agenda change, the kinds of things you were working on, simply by switching institutions, by being at Caltech?
CHANDY: Yes. UT was a very large department and was focused very much on pure computer science, without too much concern about where it was applied. It was more basics, whereas here there was so much opportunity to work with people outside the discipline of computer science. One of the early institutions we built here was called CRPC, the Center for Research on Parallel Computing, with Rice. It was funded by NSF. It was a large operation. We worked with physicists, again on building these—with computer science basics, programming languages, how to do parallel computing, but with close ties to applied mathematics and physics. That could have happened at UT as well. It was sort of more natural here, just because the Department was so small. UT did catch up, in the sense that later they started their own large CRPC. But Caltech and Rice were much earlier and it was natural here to work across divisions, just because that was the culture.
Joining Caltech and Parallel Computing
ZIERLER: In what ways was that mutually beneficial? What divisions would there be and would you be able to collaborate with? Where was your expertise helpful to them, and where was their expertise helpful to you?
CHANDY: My expertise was helpful to them in that I was working on parallel computing, and simulations of physical and chemical systems were in large part on very large parallel machines. It was helpful to them in developing the software infrastructure that they could use, and they were helpful to me in providing me with the kinds of problems, the applications, that were meaningful to mankind, so that I could tell that what I was doing was actually making a difference somewhere, as opposed to being fundamental science. I could see the impact.
ZIERLER: You mentioned parallel computing. Of course at this time you are co-author of two books, Parallel Program Design in 1989, and in 1992 An Introduction to Parallel Programming. Just some nomenclature—you mentioned parallel computing, and these books deal with parallel programming. Is that semantics? Is there a distinction between parallel computing and parallel programming?
CHANDY: Not much. Parallel programming is developing the programs for parallel computers. Parallel computers often you think of the hardware as well, and programming focuses very much on how to exploit the hardware that's available.
ZIERLER: At a basic level, what is a parallel computer or parallel computing?
CHANDY: At the very basic level, it's a collection of large numbers of individual computers that are collectively solving a common problem. As opposed to having one computer doing a problem, you now have let's say 100, and now you've got to figure out how these 100 computers are going to work together to solve this joint problem. There are various difficulties with doing that.
ZIERLER: I can imagine the first difficulty is how do you ensure against redundancy? How can you ensure that 100 computers are not simply doing the same thing?
CHANDY: Yeah. [laughs] I can go into this for a long, long time. Each computer presumably what you want to do is have it work on a part of a problem. Then it needs to communicate with other computers working on other parts. How do they communicate? They're going to send messages back and forth. Then you have a problem, just as with people, having to wait to get information from the other one. If we're collaborating and I'm working with you, and I'm ahead of you, then you've got to wait, or I've got to wait for you to catch up with me. This problem of partitioning the work so that all the computers, all the agents as we call them, are more or less in sync, so that there's not too much waiting. And there's issues as to how much communication—if you're sending lots and lots of messages, then the computers are wasting their time, or spending most of their time just communicating as opposed to computing. This is a continuing problem. I forget which year I went to Microsoft, and Bill Gates I think said this was the most significant problem for Microsoft. Because each computer was getting so much smaller and so much cheaper, so it wasn't so much making computers cheaper so much as putting lots of them together to solve a problem.
ZIERLER: You mentioned ways in which there was interdisciplinary collaboration at Caltech. What would be an example that really exemplifies how parallel computing would be useful for chemists, biologists, engineers, physicists? Take your pick.
CHANDY: Going back to CRPC, there were many applications, but two of the big ones were physics simulation. There was a professor here called Geoffrey Fox, in physics. He was very interested in parallel computing. This was simulation of very large physical systems, including climate models. One of our collaborators was Argonne National Labs. Their big push was—this is again, way back—climate models were big, but the computers weren't big enough. They were simulating primarily climate but also weather. You can see intuitively how a climate model would map onto parallel computers. Because you think of the globe, and you break the globe up into parts, and each of the parts are talking to each other. There's wind that blows across. There's ocean currents. So it's sort of natural to take the globe and partition it into areas, each of which can be modeled by a different computer. But that's in some ways much easier said than done because the parts clearly interact. That was one example. There's also examples in chemistry and more recently in biology.
ZIERLER: I was going to ask—one of the major stories of Caltech now is the role of computers in biology. But as you say, that was a later development?
CHANDY: Parallel computing at this massive scale was a later development, in particular the three-dimensional morphology of proteins, for example, figuring that out, which is an optimization problem. Now a lot of parallel computing is used for that, but I think physics and chemistry were the earlier drivers.
ZIERLER: What about in HSS? I'm thinking for example of people like Charlie Plott, an economist, who really approaches economics from a scientific perspective and relies heavily on computer modeling for his study. I wonder if you had any collaborations in HSS.
CHANDY: Not on parallel computing. HSS and Computer Science started a center called SISL—Social and Information Sciences Lab. Charlie was active there. But the problems we looked at were more fundamental computer science slash economics, like markets for green energy, markets for power systems, solar mechanism design. But it really wasn't parallel computing oriented. It was mostly the fundamental algorithmic and economics.
ZIERLER: You mentioned climate modeling in places like Argonne National Laboratory. Of course this was a time when JPL was starting to seriously get involved in climate modeling with its satellites. Did you have any work at JPL?
CHANDY: Not again on climate modeling or on parallel computing. I did some collaboration with JPL more on the correctness of programs. JPL had put a lot of effort into ensuring that their software on their space programs, that the software was correct, because the consequence of failure was so extreme. You'd lose the probe. So there was a very important effort at JPL on ensuring that the software that ran the space program was correct. That's a very tricky problem because these are what are called sometimes cyber-physical systems. Cyber because of the software and physical because of course they're operating in a physical environment. So I did collaborate a bit with them. JPL staff taught in the Computer Science program at Caltech for many, many years, and I think they still do, so there was that collaboration. There was some work on parallel computing, with CRPC, but I'd say there's a lot more work that I was involved in on software correctness.
ZIERLER: When you use the term "correctness," what does that suggest? Is it simply the difference between right and wrong, or does that word have a particular meaning in this context?
CHANDY: It has I'd say two meanings. One is the meaning of right and wrong. Say this person doing a space program has a contract. They have a contract with the software developers saying, "Here's the specification for what I want the software to do." The specification is in some abstract language, possible English. Then the software is developed, and the right and wrong is whether the software matches the specification. It's right if it does, and it's wrong if it doesn't. Having said that, the problem is that the specification itself is very complex. You can imagine what the specification for a space probe is. That's where it gets to be more continuing, rather than a bifurcation between right and wrong. Because sometimes the specification isn't quite right, and then you get the software and you've got to modify the software as the specification changes. It's usually a collaboration between what's sometimes called quote "systems science" where the entire system has to work together, and you don't treat the software as an entirely separate bloc. There are two views of the software. One is the right and wrong view, and the other is part of being a component in a system.
ZIERLER: In the mid to late 1990s, the internet becomes more and more widely available. At what part of your career in research did you start thinking about computing and its role in the broader communications network?
CHANDY: It was more that the broader communications network allowed distributed computing more broadly. The network was available. The distributed computing hardware framework was available because of the internet, and that gave an incentive to continue work on the computing. I didn't work on the internet, but the internet gave me something that made my work more useful.
ZIERLER: In the late 1990s, you served as executive officer for Computer Science. What were some of your responsibilities in that role?
CHANDY: Executive officer has several roles. One is to ensure that the academic program is up to date and that it keeps going forward. The other one is to help recruit. Part of that is working with the Division to decide what the future directions of the Department ought to be. Then there's of course the mundane things of getting staff and faculty, and office space and things like that.
ZIERLER: In the idiosyncratic way that Caltech is set up, is this more like a department chair at other universities?
CHANDY: No. I was department chair at UT, and that was a big operation. We had maybe 40 or 50 faculty, whereas the entire Division of Engineering is like 75. So it's comparable to a division. Here it was very small and more or less collegial group, so I wouldn't say it was anything like a department head.
ZIERLER: When you say "more or less" collegial, what were some of the big debates among the professors in those conversations at the strategic level about the focus of the program, what it should look to in the future for?
CHANDY: The question in some sense is how far out of the box must you think about what computer science should be. From a teaching point of view, especially for undergraduates, they need a lot of what one would think of as well-known computer science. They need to know about operating systems, programming languages, databases, and so on. Then there's more completely innovative things like auto computing, neuromorphic computing, this biological computing. There's a tension between those sorts of directions and what students, undergraduates, need. I think we made the right decision, which was to actually encourage a lot of thinking outside the box. Early work on so-called neuromorphic computing, even analog computing, again thanks to Carver Mead, which I think paid off. The more general kinds of computing, you're competing with huge operations in Georgia Tech or University of Texas and so on. Caltech really had to be visionary, but visionary has its own tensions.
ZIERLER: As you say of course, to be competitive, Caltech needs to be visionary. But just in terms of the numbers, there's so many more people, there's so much more brain power as a collective at places like UT Austin, Georgia Tech, MIT. Beyond just being visionary, how does Caltech compete when there simply aren't enough people working on any given problem? How does that work in computer science?
CHANDY: The competition is hard. It really is very hard. Numbers do count. MIT is such a huge operation. They can do much, both visionary and basic. So it is difficult for Caltech. I think it's particularly difficult with computer science because I think the ratio of computer science faculty at Caltech versus MIT, compared with, say, geology Caltech versus MIT, the ratio is much, much, much worse in computer science.
ZIERLER: Oh, that's interesting. Why so?
CHANDY: I think it's more historical than anything else. I think MIT decided very early on to put a lot of faculty resources—they're continuing—they're still growing computer science at MIT. Even I think this year, there's a massive push. I think it's more historical. Getting back to the challenge, it is very, very difficult to be ranked high when you're so tiny. There are two things I think Caltech does and we should do more of. One is to encourage visionary thinking. The second is to step back a bit so that especially young professors, assistant professors, have the time to pursue their vision. If you ask every year, "What have you done in the last year? How many papers have you published? How much money have you gotten?" then you really can't be truly visionary because vision takes time. Those are the two things that we need to continue to do more of.
ZIERLER: How does that play out from a recruitment perspective, for the best young assistant professors out there, the best graduate students and postdocs? What is the case that Caltech can make to them? Given that they can't say that we're enormous and that we have all of these resources, how does Caltech remain competitive just in terms of recruiting talent for people who otherwise could go to these much larger programs?
CHANDY: Again, the competition is very, very tough, and it's very difficult. There are much larger programs, and certainly in terms of things like salaries that are competitive. One thing we do is to point out the interdisciplinary nature of Caltech, that we don't think of you as a computer scientist as a silo. We often expect a professor to be really bright but he chooses his or her own direction, which might include working in economics or in biology or somewhere else. I think one of the attractive parts of Caltech—and again, we have to compete with—this is like MIT, which has the same story—is that the disciplines aren't siloed. We really care about good research, but you might end up working across science and engineering, or humanities. So somebody who is interested in computer science and economics, you could say, "This is a really nice place to come to, because we'll help you. If you want to teach a course in economics, or take a year off to learn about it, we'll help you." That's one of the ways I think we compete. But the competition is hard. There are places competing for the very best students, very best graduates, and the competition is getting harder all the time.
ZIERLER: When you are executive officer in the late 1990s, you're engaged in these strategic discussions. Of course the broader social backdrop is this is the beginning of the tech revolution, of the rise of Facebook and Google and all of these kinds of companies. To what extent did that Silicon Valley culture inform or permeate the academic environment, the things that were being debated at Caltech at the time?
CHANDY: Somewhat, but I wouldn't say overly so. The culture has two parts to it. One is the underlying technology that enables things like social media, that enables Facebook and Twitter and everything else. That's the underlying computer science technology, software, hardware. Then there's the application space. What does Facebook do? It encourages people to interact and so forth. We at Caltech didn't have anything equivalent to the MIT Media Lab, which looked at both the technology and its influence on society, in particular how society was changing. We were very much focused on the technology. Strategically, again, pointed out the critical importance of very massive, huge distributed systems and its applications. We didn't and we continued not to really have much of a presence in the social consequences of that, whereas I think places like MIT, maybe Georgia Tech did.
Sensors and the Infosphere
ZIERLER: In 1996, you wrote an article for the IEEE which is intriguing, The Scientist's Infosphere. What does that mean, "infosphere"?
CHANDY: By then, sensors had become inexpensive. The instruments that scientists used had now become really pervasive. The instruments included satellites that had data about the Earth. Instruments collected data all over the Earth. So the whole notion of "what is an instrument" for science was changing. Thinking about the instrument wasn't so much in terms of hardware, like building a telescope, but now that there was so much data being generated, much of it was public, so you're working in a sphere with data of all kinds available. The challenge was not to build necessarily a new instrument in a traditional way, but also to exploit all the instruments that were coming up all the time. This was early thinking about the so-called internet of things, where you think what are the internet of things for the scientist.
ZIERLER: The phrase "internet of things"—it's so interesting. How do you understand that?
CHANDY: The internet started, of course, one of the big applications of the internet was messaging, sending messages, and then of course sending different kinds of documents. But again with sensors becoming ubiquitous, they're sending messages, and the messages they send are often on the internet. All of this technology has severe dangerous consequences, but they also have potential value. The early idea was you think of the internet with lots of sensors—sensors in your refrigerator, in your house, in your garden, in your car, all talking to each other. And what could you do with the consequence of that? You can imagine things like agriculture having massive changes, widespread sensors for forestation or deforestation. That's huge potential benefits.
There are many, many dangers. It's like Pandora's box. You always worry about whether technology [laughs] in the long horizon of many hundreds of years is good or bad for mankind. One danger, of course, is hacking. If you can hack into the internet of things, then you're hacking right into the entire environment in which humans live. There's no point in just trying to scare people, but anybody with any imagination can think of what can go wrong with that. The other is sort of more societal, in how people react to having all this information available. We've talked about being a couch potato, but you can imagine being a total potato by having everything done for you. You can just tell Alexa to close the doors and the blinds, to get the stove on. There are unintended consequences. The older I get, the more I worry about these unintended consequences.
ZIERLER: The increasing utility of sensors, of course, means that you can focus more and more on building systems that sense and respond to dangerous moments. Let's start first with seismic events. Was that really a Southern California story? Were you interested in tectonic shifts and earthquakes before you got to Caltech?
CHANDY: No. I got interested in it because the geologists and the civil engineers were. I helped provide some of the computer science thinking into the system that we collectively built. But I wasn't interested in seismology before I got to Caltech.
ZIERLER: Were there earthquake events that gave you particular satisfaction that you had sensors that were contributing to better safety, better precaution, even saving lives?
CHANDY: There weren't earthquake events here. All the sensors we currently have, almost all of them, are in the larger LA basin. Since we've had the sensors, like for the last 10, 12 years, we haven't had any cataclysmic event in which people lost lives. The value that I see is that we helped civil engineers and geologists in two ways. For civil engineers, we have sensors in the skyscrapers in downtown L.A. Some of this information as to which skyscraper and so on is confidential because it belongs to the building owners. But the sensors being inexpensive, we have multiple sensors on each floor, In particular, if you have sensors on two successive floors, then you can tell how the building is shaking, because you can see how two adjacent floors are moving and understand the stresses and strains between them. Civil engineers have gotten a lot of information from this instrument which they wouldn't have gotten any other way. For me personally, it's something very valuable that the computer science is enabling building safety and for the civil engineers. The computing aspect in some sense is being a hand maiden to civil engineering.
For the geologists, it's understanding what's going on in the LA basin, because the LA basin is a very complex basin. What goes on in one area could be very different from what goes on even a kilometer away. You can't understand this variation across the Basin without having lots of sensors across the basin. This is something again that we've helped the geologists understand. That's also, to me, a lot of personal satisfaction.
ZIERLER: In terms of understanding and even mitigating cataclysmic events, I'm thinking of a hurricane, for example, where the meteorology, the climate modeling, has gotten so good that we can see the threat developing in the ocean, in the Gulf of Mexico, on the East Coast, five days or a week before it actually makes landfall. What is the kind of advanced system warning that this sensor project can do in the L.A. Basin so that when, heaven forbid, that next massive earthquake comes, as many people are as prepared as possible? What would that look like?
CHANDY: It's very different from a hurricane. It's not predicting earthquakes at all, not at all. All it's doing is catching the ground shaking when the earthquake starts. It starts in one place. The ground starts shaking. There's information traveling from the sensors across, say, the internet, and it's traveling roughly at the speed of light. The shaking on the ground is traveling roughly at the speed of sound. The difference you get in terms of early warning is the difference between the speed of light and the speed of sound, from where the initial shaking happens to, say, downtown L.A. The extent of the warning depends on how far away the ground-shaking starts. This is of the order of seconds, 60 seconds to 120 seconds, of that order. It's not days. It's not even hours. It's a very short time.
ZIERLER: What does the communication system warning look like? Does everybody get a message on their cell phone that says, "Run outside" or "Get away from masonry buildings"? What does that look like?
CHANDY: You get a message on your cell phone. The way the messaging works is there's something done by the USGS, the U.S. Geological Service, and the inexpensive array that we are developing is something that's going to eventually feed into the USGS. For obvious reasons, the USGS, the government has to be responsible for alarms, for alerting people. The current system is through the cell phone. I'm glad that Caltech is collaborating with USGS, because the whole alerting mechanism is complex because of social aspects. You don't want to tell people to run out, necessarily, because that might make things worse. So there's a whole people aspect that is being handled by USGS with some Caltech people. All we're doing is again providing a different kind of instrument to feed into what the USGS has.
ZIERLER: Of course I'll have to ask the geologists this, but from your perspective, do you have any insight for why we don't have more advanced warning for a coming earthquake? Why do we not understand or can detect when tectonic plates shift and cause earthquakes?
CHANDY: I don't know. You'd have to talk to a geologist. I have talked to them, and they've explained things to me, but you'd be better off talking to a geologist.
ZIERLER: It is fascinating, though. The technology, our understanding, it's really quite primitive in that regard. We do not have any advanced system in the way that we do for hurricanes, for example.
CHANDY: That's true.
ZIERLER: What about your work on detecting nuclear radiation? First of all, would that sensor work include both civil energy disasters and nuclear war, or do you focus on one or the other?
CHANDY: Oh no, not nuclear war at all. We weren't doing anything at the nuclear war level. We worked with the Department of Homeland Security. The project was to detect, say, a dirty bomb, something with a small dirty bomb in a backpack or something else that had radiation from it, and to catch it as soon as possible. This was very, very different from anything like a nuclear bomb. You're trying to catch very small amounts, relatively small amounts of radiation. This was with Homeland Security and also work with Lawrence Berkeley Labs, who have been doing a lot of work in that area.
The difference between that and say the seismic work is that the sensors are moving. If you think there's radiation coming from someplace and you've got a police car, the police car can move towards that region. As it moves closer, it would get more signals. The signals would get stronger. Whereas in the seismic case, the sensors are stationary. From a computer science point of view, it's hard to control how these cars move, sensors move, and how quickly can you detect, with high confidence, that there is a problem in my area. We did some of the early work, and it is being continued by Lawrence Berkeley Labs and some of the government organizations.
ZIERLER: Is it conceivable that these sensors would be useful, in figuring out if a rogue state like North Korea, for example, detonated a nuclear weapon? Is that an entirely different world of sensors, or there is a feasible applicability here?
CHANDY: No, it's an entirely different world. It's an entirely different world of sensors. Actually the seismic sensors would be more valuable in that sort of situation because the seismic sensors would pick up a detonation, say, in North Korea.
ZIERLER: What about for tsunamis? Would it be relevant there as well, seismic sensors?
CHANDY: Oh, definitely. Very much so. The tsunami—again, you should talk to the civil engineers and the geologists, because they know a lot more about it. Of course, a tsunami, you can get an early warning, depending on where the underlying seismic event was. A seismic event in Alaska gives you a tsunami warning in Hawaii, hours ahead. A seismic event off the coast of Seattle gives you a very short warning for the beaches of Seattle. So for tsunamis, certainly. And there is work at Caltech—can tell you more about it—in geology, using sensors for tsunamis. That's a fascinating area of how sensors are used.
ZIERLER: When did you start looking at sensors and their value in medicine or medical events?
CHANDY: I can't say that the work I've done in medicine has been particularly valuable. It hasn't been groundbreaking. But getting back to engineering or just basic science, as opposed to just writing the next paper on some aspect of computing, I got more concerned about the social consequences, the human consequences, of the work I was doing. I thought it would be nice to try and do something that would help people in a more direct way. So the question of interest is, as these sensors become really, really cheap, two things can happen. One is that companies will sell the sensors, say, in watch bands and so on, and the data will go to the cloud, to them, where they will analyze it for you. The other is potentially like a home kit, where somebody builds a little system just for themselves to monitor their heart, to monitor their weight, and things like that. I was interested in seeing what would happen, whether we could do some of both. Whether you could build a home kit, if you will, from inexpensive sensors, and some Caltech student or somebody else who wanted to play around with it, what they could get out of it. I wish I had done more in medicine, but I didn't.
ZIERLER: I'm curious, in 2000, when John Preskill started the Institute for Quantum Information, if you were involved in that at all, or if you were following any of those developments at the time.
CHANDY: I wasn't involved in that at all. It was so far outside the kinds of things I did that I thought I should concentrate on what I was doing at that point. I think the learning curve at that point would have been so high. Given my age, I thought I should stick to my knitting.
ZIERLER: Is that simply a function of you operating in a world of classical computing, or is there some other intellectual divide there?
CHANDY: It's not so much an intellectual divide as—to do the next sort of things, it depends on how much time you need to invest to get anything in return where you can make a contribution. At that stage of my life, if I were to do anything substantial in quantum computing, I would have had to invest so much of my life that there wouldn't be much left.
ZIERLER: [laughs] Ten years later as the internet was maturing, you were focusing on internet predictions and the impact of sense and response. I wonder if you can explain that. What is the impact of sense and response systems as it relates to the internet?
CHANDY: The sensing part with the internet included a lot of data including textual data. There's so much data being produced from video, audio, text. The book that Roy Schulte and I wrote wasn't really a computer science book. It was a book for managers, if you will, for people in business, telling them very early on that there was this huge opportunity to harness this immense amount of data, which some of it was available to them for free. And to point out—sort of predict, way back then—what was going to happen in different areas, such as agriculture. So predicting back then that the drones and satellites and cameras, that agriculture would change substantially, and giving some ideas as to how that might happen. Similarly for medicine and everything else. It was sort of a vision statement. It wasn't a traditional academic book. It has had some impact, and people have read it, but it's not academic. Some of this really has come to fruition. Agriculture is changing—perhaps not as rapidly as we thought it would, but it is changing. Similarly, certainly medicine is changing.
ZIERLER: The book you're referring to, of course, is called Event Processing: Designing IT Systems for Agile Companies. I'm curious, there in the subtitle, was the connation that with these IT systems, that companies would become agile? Or are they already agile companies, and that's why they're responsive to the kinds of IT systems you're designing and proposing?
CHANDY: [laughs] Some of both. Agile in the sense that they now had to look at different sources of information than they were traditionally looking at, and agile in the sense of changing the whole company operation to focus on data sources. The whole emphasis is on data-driven engineering, data-driven management, and emphasizing the huge value of these new kinds of data. Just video, for example, all the stuff you could do with video in agriculture. Having a plane fly over an area, and using the video to understand how bad the area had been hit by a hailstorm, for example, as opposed to having farmers go out there. These are fairly simple ideas. One of the nice things is that what we predicted back then is actually happening.
ZIERLER: In 2012 to 2014, you were associate chair for Engineering and Applied Sciences. In what way was that different than your role as executive officer in the late 1990s?
CHANDY: There are two big differences. That was the time in which we were consolidating all the departments in Engineering and Applied Science. We had many more small departments, and we reorganized the Division. For example, Computer Science, Applied Mathematics, Control Systems, were all merged together into a department. Similarly, civil and mechanical engineering were merged together. This reorganization took a lot of time. Just the administrative reorganization, talking to faculty, explaining to them what was happening, why it was happening. Not everybody was equally happy with the reorganization, so there was a lot of discussion about this.
ZIERLER: At a broad level, what compelled the reorganization? What was happening that required this?
CHANDY: It was more administrative than scientific. It was just hard to manage so many small divisions. Strategically it's often helpful to have larger groups of people. Like civil and mechanical engineering, thinking strategically about space, for example, as opposed to having these smaller groups. I think this was the right thing to have done. I think in retrospect, we can look back and say this was the right thing to do. For example, in Computer Science, certainly having applied mathematics, computer science, and control all together—not that people weren't talking to each other before, but having them all together in the same department, I think it was the right thing to do.
ZIERLER: Is that related at all to your decision in 2014 to go emeritus?
CHANDY: No. I would make [laughs] a general recommendation that everybody go emeritus when they turn 70. Not necessarily that your mental faculties are weakening, but that it's a good thing to allow more younger people to enter the faculty. As emeritus, you're not divorced from Caltech. I still teach. I'm teaching right now. I have students, mostly undergraduate students. I work on PhD committees. I think Caltech benefits best by having people at some age—retirement of course isn't mandatory, but I think it would be a good thing for faculty to retire themselves. I had decided that 70 would be—because for me personally, if you don't make up your mind that that's a deadline, then when the time comes, it's easy to convince yourself to wait another month, another year, another year, another year. I think that hurts the institution.
ZIERLER: We talked a little bit about this way back in our first discussion. It bears a little more discussion now because we're in the historical chronology. Was there a particular area of research that you wanted to devote more time to that going emeritus would give you that extra bandwidth to do?
CHANDY: There were two things. One was—and continues to be—I'm really interested in teaching. This is a broader discussion. Caltech, among other institutions, is expensive. It's expensive for a reason. There's two parts to it. One is the actual teaching of a course, if you will. When you teach a course, there's material that's, say, ten or 15 years old that's more or less stable, and then there's new material. I think Caltech, for example, has a responsibility to make this material freely available to everybody around the world. The other part is where you work one on one with a student, working together on research. That experience cannot be scaled up because it's very much a one-on-one thing.
I was and continue to be interested in scaling up the teaching of courses, especially with the internet available, because you now can have videos and audio. You can have lots of diagrams, which you couldn't have in a textbook. The cost is per page and that limits how many diagrams one could have. Certainly couldn't have videos. I think MIT is doing this with OpenCourseWare. But I'd like to see what I could do in this area of distributed computing and later sometime in the future with looking at sensors. This course this term is the first iteration of this. It will take many iterations to make it really, really useful to everybody.
There are many challenges there, because to make it accessible to a wide audience, you've got to allow people who don't necessarily have the background to understand the material, so you've got to help them up without boring people that know all the material, so you need multiple ways of entering into the documents. I'm thinking through all of that. That's something that excites me and continues to do so. There is a question about the relative cost of education in the United States and what it's doing to the culture of the country.
ZIERLER: You mean in terms of income inequality? In terms of a lack of upward mobility?
CHANDY: All kinds of things. Because you've got to first of all know how to apply to Caltech or a similar institution. It's so very hard to get in. There's got to be a way of scaling the intellectual depth of Caltech to provide value to humanity as a whole, as opposed to the 2,000 people on campus. I don't quite know how to do that, but thinking that through in my course this term is the first step in that direction. That's one area. The other area is I'm still interested in thinking through how this revolution with distributed computing and sensors can help ordinary people in a meaningful way. Again, I haven't thought that through completely.
ZIERLER: Is this to suggest that, at this latter stage in your research career, you're more focused on applications, helping the day to day, than in earlier portions of your career when you might have been more quote unquote "academic" or theoretical in your pursuits?
CHANDY: Yes, yes. Again, there's so much of technology that has been maleficent. It has hurt people. It's worrying. It's worrying at many levels. As you get older, you sort of worry more about the planet you leave behind and what it's going to be like in 100 years. You don't worry about that so much when you're 25.
ZIERLER: I wonder if it's when you were 25, and the law of unintended consequences, if your outlook on humanity was different to some degree, where you might not have expressed concern about the ways these technologies might have been applied because you perhaps had a rosier outlook on human nature and what people would do with the technology, and not thinking about misinformation and threats to democracy and all of these things.
CHANDY: This is a very deep [laughs] philosophical question. This is something that again goes back centuries. Who was this English physicist that said that the role of scientists is to do science and not worry about consequences? This was I think before the atom bomb was exploded. There's a reasonable argument that the role of scientists is to do science, and it's the role of politicians or society to decide how science is used. Maybe that's the argument I had in my mind when I was 25, but it's not an argument that I accept today. I can see the consequences of internet technology and computer science. I'd rather think about—again, there's unintended consequences. You think you're doing something helpful that can be used for all kinds of nefarious applications. I'm more interested in the applications now than when I started out.
ZIERLER: A year before you retired in 2013, you wrote a paper Distributed Snapshots: Determining Global States of a Distributed System, which won the Hall of Fame Award from the ACM. I wonder if you can explain why this paper resonated to the degree that it did.
CHANDY: It turned out to be one of the more—if you were to pick, say, four or five fundamental papers in distributed computing, it turned out to be one of those. In a nutshell, the question is what can one agent—an agent is a piece of software—what can an agent know about what another agent is doing? If you and I are agents in a distributed system, and we can exchange messages, what can I know about you are doing at this instant based on the messages that I've been getting? This concept of what can one thing know about another thing turns out to be very fundamental for distributed computing, as you can imagine. That's why it resonated.
ZIERLER: On this topic, you're a humble person, but if you'll indulge me if only to explain some of the history behind the recognition, I'd like to go over some of the ways that you have been honored over the course of your career. Let's go back to 1995, the Kobayashi Award from the IEEE. What is the Kobayashi Award, and what were they recognizing you for?
CHANDY: The Kobayashi Award is for contributions to communications and computing, but more emphasis on the communications network. I had done—again, luckily, there's a few fundamental papers on queuing networks, so networks of queues were jobs—people go from queue to queue. The internet is a network of queues. This was very theoretical work when we gave formulae and approximations for dealing with analyzing lots of networks of queues. This was why I got that award.
ZIERLER: That same year, you were elected to the National Academy of Engineering. I wonder in the chronology of the National Academy how far back computer scientists went in being elected to the Academy.
CHANDY: You know, I don't know. That's a really interesting question. When is the first so-called pure computer science scientist elected to the National Academy? I'm not sure. There were a lot of applied mathematicians way, way back. A lot of physicists who did computer science, Mead, way back. But I don't know when sort of the more traditional scientists got in. That's a good question. I've got to look it up.
ZIERLER: The other question there is given the voluminous growth of computer science, why was it glommed onto the National Academy of Engineering and why is there not a National Academy of Computer Science?
CHANDY: [laughs]
ZIERLER: I guess the deeper question there is, do you see computer science as a subset of engineering? Is that the way to look at it?
CHANDY: There's a lot of mathematics, but again you can think of applied math as a subset of engineering. There are some people who do more pure mathematics part of computer science who also get elected to the National Academy of Science. To some extent, these awards really aren't important. It's unfortunate that so much attention is paid to them. What really matters is whether the ideas you have last 50 years or 100 years.
ZIERLER: What I'm interested, particularly with the National Academy—it's a huge honor. Whether it's important to you personally is a separate story. But given that it's a national honor, does it open a world to you of connections and ideas, simply the membership? Does it have a utility as opposed to being something where you can feel really good about your accomplishments? That might be fleeting. Is there a material benefit in the membership, in the people that you meet and the opportunities that might be available to you that otherwise would not be?
CHANDY: One opportunity is I worked on some committees, in particular a committee on looking at sense and response systems for crises. This was a committee set up through the National Academies, in various committees, and I got to participate in that. In that sense, it's easier to participate in such policy committees being a member of the National Academy. But did being a member of the National Academy open doors for me? No, I wouldn't say so.
ZIERLER: Again, if it's not so important to you personally, perhaps as an opportunity to talk about the people that are associated with these awards, the ACM Dijkstra Award in 2016. I wonder if you can talk about Dijkstra.
CHANDY: [laughs] Dijkstra was a really fascinating man. He was one of the two or three earliest pure computer scientists, who thought of programming as a discipline in itself. This must have been late 1950s. It was Dijkstra and another person called Tony Hoare, who's now Sir Anthony Charles something Hoare. Dijkstra was also somebody that I recruited to UT Austin. He was Dutch. He did some of the really truly early ideas of what programming ought to be. He was also a very good friend of mine. So it was really nice to get the Dijkstra Award. It made me particularly happy.
ZIERLER: A similar question—the following year, from the IEEE, the Harry Goode Memorial Award. I wonder if you can talk a little bit about Harry Goode.
CHANDY: Harry Goode was an electrical engineer. I don't know all the details of all the work he did, but it was again, a long time ago. The work that I got the award was for the work with Jay Misra on distributed computing. Again, the Harry Goode Award, I'm really honored to have gotten that award. It is given to a very select few, and I am glad to have somehow gotten into that company.
ZIERLER: One distinction, and then I promise no more discussion on awards for you—must have been personally meaningful—in 2018, the IIT in India recognized you with the Madras Distinguished Alumnus Award. I wonder if that was a moment of reflection for you in terms of, first of all, how the IIT had changed over the decades, and perhaps even in a time machine, would you have come to the United States if you had graduated from the IIT in 2018, or were opportunities in computer science and technology in India so far developed that the need to do so would be less strong than it was for you decades earlier?
CHANDY: I'll answer the second question first. There's no place in the world that competes with MIT or Caltech as far as computer science research goes. The IIT is a terrific institution and there's lots of opportunity in industry and startups. Huge numbers of startups. If I had graduated now, I might have gone on to a startup in India, which I think is often the right thing to do. You can make a big difference. But again, if you really wanted to do research, there's no place like the U.S. If you look at the top institutions, even outside the U.S., there's a handful of institutions in Europe, but that's about it.
ZIERLER: Did you have a chance to go back to the IIT? Did you give a speech? Was there a dinner in your honor?
CHANDY: Yes, there was a speech [laughs] which is somewhere in their archives.
ZIERLER: What did you talk about?
CHANDY: I talked about getting older [laughs] and the value that the IIT gives in making you—the education was really superb, making you think about science, making you think about engineering, making you think about the world, if you will. It makes you interested in everything. It makes you interested in physics, chemistry, the Krebs cycle, what's going on in the garden outside. It makes you passionate about the planet, the cosmos, to use Carl Sagan's phrase. Caltech does that too. I had to give a talk in some Caltech venue to young scientists, and that's really what I emphasized, what comes across, is that there's—there have been some recent articles about science and religion. Yesterday in The New York Times, the question was, are they fundamentally opposed to each other? I think it was a debate between Dawkins and Sacks in Oxford. The article talks about the wonder of life, if you will, and the wonder of everything, of understanding. Understanding why and how a leaf does photosynthesis. I think science gives you that sort of wonder. Not that you wouldn't have it if you didn't know what the Krebs cycle was, but it gives you this additional wonder of everything around you.
ZIERLER: An overtly political question—given that computer science in the United States is so reliant on international students—as you say, places like Caltech and MIT for research remain the best in the world. When the Trump administration made it difficult for international students studying in the United States, did you feel that on campus? Was that an issue at Caltech that you had to contend with?
CHANDY: No, no, no. It wasn't an issue. By then, I had retired, for one, but I don't believe it was an issue on campus. I don't have the statistics in front of me, but again, it's something I read very recently—a very large fraction, a huge fraction of the successful startups have had at least one founder who was born outside the U.S. It's not like 5%; it's a huge fraction. A very large fraction of the Fortune 500 have senior people who were born abroad. So I think the U.S. benefits—not just I think; the statistics bear it out—it benefits hugely from being so attractive to people from around the world. Often people think of it purely in terms of financial gain, but that's not the point. It's attractive in ferment of ideas and being open. I think a lot of that would be horrible for the country if that was lost, if there was any sort of anti-immigrant feeling to arise in a major way.
ZIERLER: Are you concerned that that's a trend that the United States is going in right now?
CHANDY: If you look at the history of the U.S., it has gone up and down. There has been periods, the Know Nothing period. The history of the U.S. is fascinating. It has gone through these cycles. I don't want to go through electoral history now. I'm not too worried. I expect the cycles to come back, but I think eventually, good sense will prevail.
ZIERLER: Do you see the same patterns in India, where there has been a notable rise in Indian nationalism?
CHANDY: Yeah, there is. It's a global problem. There is massive flow of people for a variety of reasons. There are people flowing into India from Bangladesh, Burma, elsewhere. People flowing into Europe. As of today, there's Belarus, big problems, people coming in. People coming into the U.S. I think that these flows are going to become much worse, much larger, due to climate change. The consequence of these flows of immigrants is a nationalistic feeling to protect the nation against outsiders, if you will. This is just going to get worse unless we do something about the whole climate situation. If rice fields get saline, the salt gets into the rice fields and the productivity goes down, there's going to be huge problems. Those are really survival problems, and one can explain this whole nationalistic thing as a consequence of that.
Computation for Universal Benefit
ZIERLER: Mani, now that we've worked the narrative right up to the present, for the last part of this excellent series of discussions that we've had, I'd like to ask some broadly retrospective questions about your career. Then we'll end looking to the future. Just to stay on this topic of these massive problems that the globe is facing right now, in economics, in climate change, in population, in politics, and go back to an earlier conversation with the philosophical underpinnings of science and technology, for you, now that you are thinking more and more about applications that have a positive benefit, in all of the areas of research that you've been involved in, in computer science, where are you most optimistic that the research that you've done, the discoveries that you've made, can have a positive impact on the most distressing problems, wide problems facing the globe right now?
CHANDY: That's a difficult question. Surprisingly, I think looking back, in retrospect, that the theory that I developed has the potential for having a larger impact than all the practical work I've done. Which makes me think again about the relative importance of basic theory. Like a global snapshot algorithm, the queuing network stuff, it has been used by other people—not by me, but by other people—to build things which have had an impact. Now, has the impact always been positive? No. The distributed computing has been used for a lot of cloud applications, many of which are beneficial, but not all of them are. This gets back to the fundamental scientific question of how can one predict how one's research is going to be used. Who knows?
ZIERLER: You mentioned before that far more important than all of these awards is the question of longevity and durability of ideas. In 50 years, do your ideas stand the test of time? Well, Mani, you've been around for long enough where you can test that in your own research career. Going back to when you were a graduate student, when you were in your twenties, the ideas that you had that were a foundation, formative to all of the research that you've done since, what has stood the test of time and what hasn't?
CHANDY: Some of it has, to my surprise. The papers I wrote, for example, in 1969, 1972, they're still very widely cited. An interesting question is, in 1972, did I know that 50 years from now it would be widely cited? The answer is no. I thought it was a nice paper, but I had no idea what its longevity would be. Likewise, there are quite a few papers that have lived that long and that I expect to continue to live for another 50 years. But one of my students asked me this question—"When you write a paper, can you tell how long it's going to live?" Sometimes, you have a clue that this is going to be valuable, but valuable for the next ten years, 15 years. But valuable for 50 years? It's really hard to tell. I think it's partly luck.
ZIERLER: In all the research that you've been involved in, what stands out in your memory as really bringing to life the Socratic concept that when you learn a little bit about something, it opens up a whole world where you recognize all that you don't know, that you didn't even know you didn't know until you discovered the thing that opened up that portal?
CHANDY: I think all the important discoveries I've made have been through teaching. I've tried to teach something and make it clearer and clearer and clearer to my students. As I try to make it clearer, I understand the fundamentals that sometimes are missing. Then I build upon these fundamentals. So like the queuing network work, I was trying to teach these very complex formulae, teasing out what made these formulae work. Then it turns out there's something really simple underneath it. Exposition of that simplicity is what made those papers useful, and people built upon them. It's similar for distributed computing and this whole notion of unity. Just trying to teach, and I'm still trying to do that. Even today's class, after I finish our talk today, I'm going to go back and think about what I'm teaching and what I could do to make it simpler. Right now I'm teaching cryptocurrencies, and the cryptocurrency algorithms are tricky. I have only one week to teach it, so I'm going to keep working on making the really critical ideas available to students. Maybe something important will come out of it. Going back to the Socratic method, I think that's really the basis of many of the discoveries I've made.
ZIERLER: You've been thinking about computers and education for so long. I'm curious at what point in your teaching career you saw generationally the impact of students who grew up with computers, in a way that you certainly didn't, and even I didn't. When did that happen?
CHANDY: When did that happen? Certainly even the very young are now doing sort of programming. To think in terms of a program is sort of natural for a lot of people. How did that impact education? I'm less certain. I really don't know how that thinking for many years, as somebody starting programming at the age of 12, comes to Caltech at 18, and how that changes how they learn at Caltech. That I'm not sure about. It's again an interesting question.
ZIERLER: In the perennial debate about why Caltech always refuses to grow, why it's so insistent on staying small, specifically with your research career, computer science, what has been valuable to that, and what has been the challenge that has held back the discipline at Caltech?
CHANDY: I'll answer the question in a different way. If I were graduating from MIT, say, today, as opposed to 1969, and I had the opportunity to come to Caltech or go to Stanford or MIT, or even UT—huge institutions—I would really think twice about coming to Caltech, because the Computer Science Department is so small. I think it does help to have large groups of people, large numbers of students. I think Caltech has been hurt, really severely hurt, by being such a small presence in computer science. The good thing, of course, is that there aren't divisional walls. The campus is small. You can walk across from one place to the other. You've got The Red Door. You've got the Athenaeum. One little story—it's unimportant, really, but it's a story about Caltech—is that I was once sitting next to Ed Lewis at the Round Table at the Athenaeum. Do you know about Ed?
ZIERLER: I do.
CHANDY: Ed got the Nobel Prize. He was working on genetics. He had a little trivial computer science problem. We had lunch. "I've got this little thing I'm doing with genome sequencing." It was a problem that the programmer, any programmer, undergraduate, anybody, could do in a matter of hours. I said, "Hey, Ed, I'll send you a little program this afternoon." Which I did. From a computer science point of view, it's totally unimportant. But Ed got it, and he said, "Oh, thank you so much. It will help me to do something or other." And of course what I should have done was put him in touch with an undergraduate. He probably did get some undergraduates and built up on that. But I think it was so cool to happen—to sit next to a Nobel Prize winner who was so humble. Ed, he was a wonderful guy. Sitting around the Athenaeum Table, talking about something that he thought was difficult. I know somebody in high school could have helped him out. That's the sort of thing that you wouldn't get, and is less likely to happen, at MIT.
ZIERLER: Are you an advocate for growth? Are you in a position where you can say or where you're motivated to say that Computer Science at Caltech should get bigger?
CHANDY: Yes. I'm not in a position to say that, because I've retired, but I certainly feel that way.
ZIERLER: What are the prospects?
CHANDY: Unlikely. Because if Computer Science grew, something else has to shrink.
ZIERLER: Because the size of the pie stays the same is what you're saying?
CHANDY: Yes.
ZIERLER: The remarkable thing here, when you're talking about how small Computer Science is—well, as a slice of that small pie at Caltech, in relative terms of course, it's enormous. It's much bigger than other programs, given the fact that all of these undergraduates are interested in it. How do you square that circle?
CHANDY: Oh, it's a major, major problem. The circle is not being squared. It's because the faculty to student ratio is poorer in computer science. The faculty to student ratio is good for Caltech on an average, but not for students who vote with their feet and do computer science.
ZIERLER: Do you think that the next generation of students will be motivated to work as hard as they have in the past, where these people in tech jobs are working 70, 80, 90 hours a week? Do you see generational changes in the way people are thinking about work-life balances in the world of technology?
CHANDY: I don't know. I've had students who, for example, went to Google, after PhDs, who have had children, and young men get I think six months paid leave after a child is born, and who take that leave to bond with their children, even if perhaps—I don't know if there is a consequence as far as the career path goes—but who have chosen to take that time off. I think these tech companies, the big ones certainly, give you the opportunity to have a better work-life balance. But they do work very hard, too, especially in startups. But I think there's more opportunity now for better work-life balance.
ZIERLER: The promise of technology, going way back in history, has always been based on this false assumption that it's going to reduce the burden of labor in our lives. That we'll have more time for leisure and less time for work. Do you think that's universally true? Will technology actually at some point get us to a place where we're not working so hard?
CHANDY: That's again a difficult question, because work is valued. People have their self-value associated with work. A big problem with the opioid crisis, a big problem with longevity in the United States decreasing for three years in a row, I think, is a consequence of not having meaningful work. So the question you pose has a double edge to it. If you think of a society where there is enough technology that you get everything working say two days a week, would that be a happier one? It's not clear. It is an important fact that longevity in the United States, for the first time, has decreased the last three years. If you measure human happiness just in terms of longevity, something has gone wrong. Part of what has gone wrong, I think, is the absence of quote "meaningful" work, whatever meaningful is. So I wouldn't answer your question as to whether technology is going—I think people want to work, and they want to work where they get validated, and the validation comes from a group. If validation isn't activated through a group working together, then the validation is sought after through internet groups, and all kinds of weird things happen. I'm not that sanguine about not having a lot of work. I think you do need villages and families and factories and social cohesion that work in a stationary way in that area provides.
ZIERLER: Two final questions. Because you've approached computer science through a distinctly human prism, you've always been focused on the philosophical side of things. I'm curious, for you, as you assess the beliefs, the interests, the convictions that are most important to you—philosophically, politically, even spiritually—from all that you've learned and studied in the world of computer science, do you think those things have changed your core beliefs? Or do those core beliefs preexist all that you've studied?
CHANDY: The core beliefs preexisted, because growing up in India in the 1940s, there was so much evidence of suffering. You didn't have to look out far. You could look out the window to see suffering. To somehow alleviate suffering, in whatever way, was something meaningful. That's what engineering could help do. So this was, say, 1944. But now, I'm much more pessimistic than I was then. Because there are so many other kinds of suffering brought on by technology. Just look around. It's certainly not a panacea, because it depends on how society uses it. Some aspects of the technology—for example, the Green Revolution, there's a lot of opposition to GMOs, genetically modified organisms, but without GMOs, we wouldn't have had the Green Revolution and so many people not starving. GMOs have other potential impacts, long term. So it's a difficult question. I'm more pessimistic now than I was then.
ZIERLER: Last question, looking to the future. You mentioned that luck plays such an important role in assessing the durability of ideas. One of those lucks, of course, is simply a matter of timing. You came of age really at the dawn of computer science as a discipline, where there were computers that could be used towards scientific purposes. Today, if you're in a position to advise students—particularly because you are pessimistic, that there's a certain degree of despair in terms of looking around and seeing what technology has wrought—for students today who are in the same position you might have been in the 1960s looking at the dawn of a new discipline, what are the areas where you might encourage students to look at, both in terms of the quantum leaps in technology, the same kinds of leaps that you saw when you were a student, and your political and social concerns about what those technologies can do for good or bad? What might be some formative advice students today in computer science might look to, as they look to forge their future?
CHANDY: I'd say look at biology. If I were to get a PhD now, I'd look at computational biology, from two points of view, the two points being interesting academic directions and social impact. There's a huge amount of opportunity in understanding biology and biology engineering, bioengineering, with a computer science framework. As far as applications go, I think as far as dealing with agriculture, biodiversity, saving the planet through reforestation, using drones or whatever, understand medicine—it's this combination of computer science and deep understanding of biology. So if I were to pick one discipline, I would say biology, generally, more than anything else. Computational biology would be my recommendation.
ZIERLER: This has been a fantastically wide-ranging, deeply philosophical, and immersive conversation throughout all of your years in computer science and at Caltech. I'd like to thank you so much for spending this time with me. I deeply appreciate it.
CHANDY: Sure. My pleasure, David.
[END]
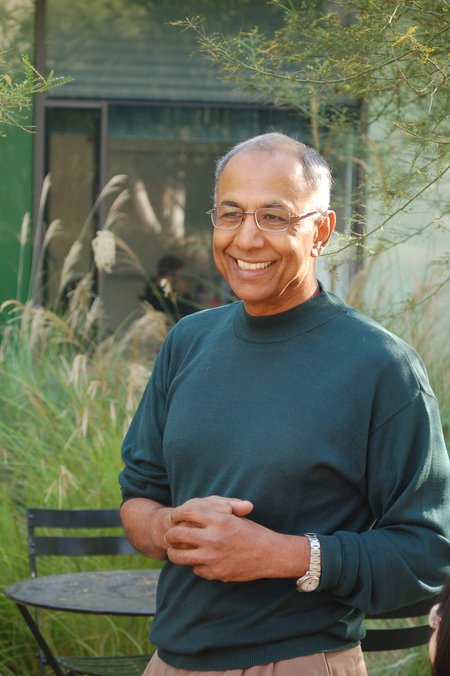
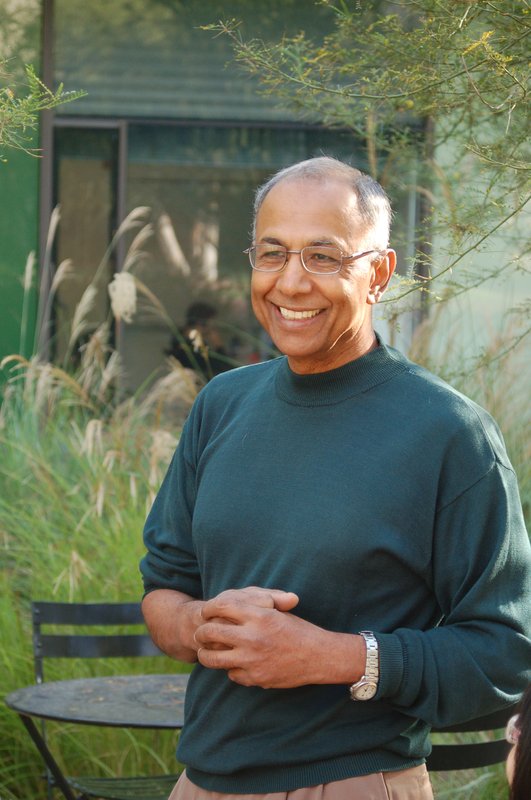
Interview highlights:
- The Popularity of CS at Caltech
- The Origins of Computer Science
- The Social Utility of Distributed Systems
- Computers and a Theory of Everything
- Early Computation at Honeywell and MIT
- The Value of Philosophy in Computer Science
- Joining Caltech and Parallel Computing
- Sensors and the Infosphere
- Computation for Universal Benefit