Richard Doyle, Data Scientist and AI Space Science Researcher
For the Jet Propulsion Laboratory, operating at the literal and metaphorical frontier of space science and engineering, leadership in the fields of machine learning and artificial intelligence has been a priority since the 1980s. From navigation systems to rover decision making, JPL has demonstrated that leading-edge research in artificial intelligence can dramatically increase efficiencies and capabilities across a wide range of planetary and space missions - and in certain cases, can even make them possible.
Richard Doyle has spent his career at JPL at the center of these developments. After completing his doctorate at MIT, where he focused on artificial intelligence and simple machine capabilities, Doyle joined JPL's Navigation Systems Section. His other key roles included managing the Information and Computing Technologies Research Section, the Artificial Intelligence Group, and the Information Technologies and Software Systems Division. With his most recent responsibilities as program manager for Information and Data Science, Doyle has a wide-angle view not only of how machine learning and AI has revolutionized JPL's capacities over the years, but how these technologies serve as a point of connection across the spectrum of federal science agencies.
In the discussions below, Doyle expresses particular pleasure with the opportunities he has had to collaborate with Caltech professors. At the dawn of Astroinformatics - coming at a time in astronomy when digital detectors were collecting more data than humans could reasonable process - Doyle's leadership in harnessing JPL capabilities with Caltech science objectives to find "signals amid the noise" demonstrates the power of the JPL-campus partnership, it heralded a new era in astronomy, and it was a foundation point for researchers in other scientific fields to grasp the utility of artificial intelligence. In many ways, Doyle's career can be seen representing bookends of the early days of artificial intelligence research and the current framework of AI's widespread adoption in science. But as Doyle emphasizes, it is impossible to predict where the field goes from here. That lack of predictability is one of the most profound issues confronting humanity's future.
Interview Transcript
DAVID ZIERLER: This is David Zierler, Director of the Caltech Heritage Project. It is Thursday, February 23rd, 2023. It is my great pleasure to be with Dr. Richard J. Doyle. Richard, great to be with you. Thank you for joining me today.
RICHARD DOYLE: Thanks for including me in this project.
ZIERLER: Absolutely. To start, would you please tell me your current titles and affiliations?
DOYLE: I am recently retired from JPL, as of about a year and a half ago, but I still have a connection, and I'm called a Technical Consultant for Information and Data Science, which is pretty close to my last role at JPL, which was the Program Manager for Information and Data Science. That's the punctuation of a long career, and the theme of the whole career arc was computer science meets space exploration, which I always think is a pretty cool job description, and it was a privilege for those 40 years.
ZIERLER: Are you serving as a consultant externally for any organizations also?
DOYLE: Yes. In fact, recently I did a project with AIAA—American Institute for Aeronautics and Astronautics. This was to curate a one-day strategic planning meeting, a summit, on the future of space autonomy. I found it a rewarding piece of work because in many ways it allowed me to continue engaging with some of the strategic challenges which hadn't—it's in the nature of strategic challenges that they don't get crisply resolved; they kind of continue. This AIAA work allowed me to pick up on some of the same threads, but now at a more national level, as opposed to an institutional or agency level—Caltech, JPL, or NASA.
ZIERLER: Was the decision to retire a natural conclusion, or did COVID play a role in the timing of your decision-making?
DOYLE: That's an interesting and fair question. I can say that COVID did play a certain role, not a dramatic role. It wasn't myself becoming ill or family members becoming ill, knock on wood, but it had more to do with—a few months into the COVID disruption—retirement was on my mind. Because I am a strategic planner, I always had a plan that when certain milestones were reached I would consider retirement. What was unexpected is COVID created a psychological separation from the workplace, and I realized that that was one of the transitions involved in retirement. So in some weird way, COVID made it easier, more natural, because this place I think of as JPL, I hadn't been experiencing it for several months, so it just helped to catalyze my thinking.
ZIERLER: Even for a life in computers, where theoretically you can do these things remotely, for you being on site, having that interaction, that was important?
DOYLE: Oh, yes. I think that's always true, even for a committed computer geek like myself. Over the years I learned the value of face-to-face interactions and those dynamics that happen, which lead to creative activities, whether it's science, engineering, or other kinds of endeavors. Having that mix of human interaction with gestures and facial expressions and those nuances—
ZIERLER: They get lost in translation.
DOYLE: Zoom and similar, it's a great technology, but it doesn't go all the way.
ZIERLER: For your current consulting work, what are some of the things you're working on, and broadly, what's interesting to you in the field?
DOYLE: Some of the long-arc challenges—I'm jumping ahead a little bit, but we can come back to this later—one thing that's interesting about computer science and data science is that they're fundamentally cross-cutting in nature. What I mean by that is if you find a solution that applies to—I'm just going to use this for the sake of example—that applies to astronomy, it might apply with just a little bit of tweaking to, say, Earth science, and then again to something unexpected like how we formulate missions or even business practices. In that sense, solutions in computer science and data science can be very general. In fact, people who get their PhDs or whatever are trained to think in terms of general solutions.
If you contrast that with traditional engineering disciplines, which is in some ways more the core of JPL, people in those fields are trained to think in terms of optimization, solutions that are specific to a well-defined problem. That's great, especially for the first-of-a-kind missions that JPL does, but then carrying over solutions to the next mission can be more challenging, because you weren't necessarily designing it with that generality in mind, whereas computer scientists do that naturally. When we're coding, we're always thinking about, "Okay, it solves this problem, but what else? What larger scope can this code address?" That kind of thing. It's a different way of looking at things. I think both are important. Sometimes there's almost an interesting kind of tension. It's almost like two different world views—optimization and generalization. Back to your question, that's one of those itches that didn't get fully scratched, because—
ZIERLER: Probably never will.
DOYLE: —and maybe never will, because there's some fundamental opposition there, and a good tension, but it needs constant work. I have always felt that as a computer scientist, part of what I was trying to do was to find those not only effective solutions but ones that could be readily reused on the next mission. That didn't always work as smoothly as I might have wanted. So, that's one of those itches that still needs scratching. In doing the AIAA work, maybe a little unexpected but rewarding at this national level, indeed there were plenty of people that were thinking about those same challenges, in terms of perhaps influencing research programs at a national level, with large agencies—NASA, DARPA, et cetera. That appeals, and so was rewarding to get involved with that work.
Space Science Via Computer Science
ZIERLER: Of course by training your area of expertise is computer science, but in a career as long as yours at JPL, have you almost become an honorary space scientist? Has your focus and support of space science really broadened your academic area of expertise?
DOYLE: There's probably more than one way to answer that question. First of all, yes. I think everybody, especially if you spend decades at JPL, you learn a lot about space science. But I can actually go deeper than that. I would say that my interest in coming to a place like JPL in the first place had more to do with my interest in astronomy and planetary science than originally my interest in computer science.
ZIERLER: Oh, wow.
DOYLE: Computer science became the—I don't want to quite say a means to an end, but I found I was good at that kind of thing, and to this day I feel it is certainly relevant, maybe even central, to what JPL is doing and striving to do. But yes, the call was always about astronomy and space science in the more general sense. So, yes, I basically soaked it up for all those decades. I learned and experienced as much as I could, the thrill of discovery, and that continues. I don't think I'm ever going to lose that. Back to the computer science meets space exploration, as I became trained in computer science, and got more excited about that field on its own merits, particularly artificial intelligence which is where I concentrated, and then combining that with space science, it was kind of like the dream job.
ZIERLER: Some overall questions as they relate to the discipline. If we can imagine a Venn diagram—there's computer science, there's artificial intelligence, there's machine learning. Where are the shaded areas? Where are the unshaded areas?
DOYLE: That's a very difficult question to answer, and it's almost a squishy question. I'm going to give maybe an unsatisfactory answer to that question.
ZIERLER: Maybe it doesn't have a satisfactory answer!
DOYLE: I find that striving for whether it's a Venn diagram or some kind of definitional treatment of terms—in the long run, I don't think it's that critical. We do want to understand categories and boundaries and that kind of thing, but sometimes people just get into the deep dive about, like, automation versus autonomy, or AI versus machine learning, and it can actually have the effect of keeping people confused rather than really clarifying things. I'll admit I don't myself have a crisp answer or definition to those terms and where the boundaries are. They do tend to blend into each other. Somewhere along the way I just became okay if not completely comfortable with that and didn't find it terribly productive to keep striving to find that crisp definition that people could agree on.
ZIERLER: Have those definitions been even muddied over the years? Is that possible, also? Would that have been an easier question to answer?
DOYLE: I wouldn't say "muddied," but I think the way those terms are applied has evolved over the years. Artificial intelligence is really fundamentally a multidisciplinary type of endeavor. One reason why perhaps it's hard to land on one definition is you've got computer scientists, you've got psychologists, you've got mathematicians, you've got cognitive scientists, and they all come at it from a different angle. Neurologists. Those are all legitimate, but perhaps we shouldn't expect them to all come together on one definition.
ZIERLER: Has computer science at Caltech been an asset for you over the course of your career?
DOYLE: I would say yes, but not as much as I might have wanted. Part of it is my own career arc, where early on, being a more junior staff person at JPL, I wasn't always in the places where the interactions with campus were happening. Later on, I got more involved, for example with Professor George Djorgovski. I'm jumping around a little bit, but we first collaborated back in the early 1990s, and that was successful. I'm sure we'll come back to that. It was an early machine learning application to an astronomy data set. But once that happened, then I knew the possibilities for campus and JPL to work together and always looked to find other research collaborations like that. Those have continued, maybe in fits and starts, up to this day. I still know George well and we still interact and work together, so that has been very rewarding.
ZIERLER: If you have a handle at all on undergraduate students' interests today—as you may know, in the 1950s and 1960s at Caltech, everyone wanted to be the next Feynman. They wanted to do physics.
DOYLE: Yes, right.
ZIERLER: Today, of course, the overwhelming interest among undergraduates is computer science. Whether it's biology or physics or chemistry, they understand computation is so important. Or it's just computer science in and of itself that they're so interested in. From your perspective, what has accounted for this shift, the way students have voted with their feet?
DOYLE: There's probably a couple of reasons behind that trend. One is that we're all aware of a certain digitization trend of society in general, with the internet and all the rest. So, it's not surprising, and it's certainly natural that career interests and opportunities would track that. It seems like we're still just scratching at the possibilities. What does the digital future really mean? Computer science, as I said, I kind of started from astronomy and planetary science, but once I got engaged with computer science, it became fascinating on its own terms, particularly artificial intelligence.
Let me go back to my graduate student days, thinking about what I found so fascinating about AI, and then how it has changed, perhaps, in the meantime. One of the insights I had early on while I was a graduate student, during those many years I was at MIT, like six or seven years, I had the privilege of spending my summers at JPL, so it was a really nice mix of different kinds of activities. One little aha moment I had was, how do people approach AI? What does AI mean in terms of a technical endeavor? This is a little simplistic, but there were those—and JPL is an example—where AI can have use if it helps us with our exploration goals, endow our robotic machines with more capability than they might have otherwise. A great example of that, bringing it up to pretty much the current time, is what we do with the Mars rovers. The entry, descent, and landing sequence, with active computer vision, not only enabling a safe landing but a precise landing. Then once we're on the surface, the ability now—you literally just tell the rover where to go and it computes a terrain map with obstacles, and it is able to plan its own traverse path. These missions you could say have become to a certain extent enabled by the use of AI. So, that's AI as an engineering solution, to achieve some larger technical goal.
On the flip side, there's AI as a kind of laboratory for how minds work in the more general sense. Human minds, artificial minds, and those really tricky philosophical questions like, "What is consciousness?" The Turing test, sentience, all those things. Those can become and do become fascinating just on their own merits, even if they're not immediately attached to anything having to do with landing on another planet or what have you. So, there's AI as one category of engineering solution, and then there's AI as contributing to these more fundamental questions of what are minds, and how do they work? You can even string it to philosophy. Those are very different, but AI definitely touches both.
Now, if we ratchet it forward to the present day and think about the burgeoning interest with younger people choosing AI as a career, I think all that is still there, but there's more. There's driverless cars. There's ChatGPT, very recently in the news, which has created quite a bit of buzz. Maybe that's something else we can come back to, because that's both good and bad. It's good because it shows the potential for AI as a technology, but very quickly things can get a little bit overblown, perhaps, as to what it is doing, and what its limitations are, and what are its proper uses, and that kind of thing. As far as we can see, that's just going to keep happening. AI is going to be central. It can go to everything from careers, employment, technological advances, social aspects, care. It will have a role in all these things, and I'm sure many things that we don't see yet.
Of course, it can give some pause, too, to the extent that AI is a technology that's both hard to explain and—how do I put this?—maybe where I want to leave this is another one of those central and tough questions surrounding AI right now, which is the explainability question, which is, we observe an AI system doing something interesting, but we're looking at it in an input/output or black-box manner. Can the professionals really explain what's going on, and will it reach a point where that gets trickier? Even today, with some of the neural network technologies, it can be very difficult to understand exactly what's going on internally and computationally. We can see the results, we can try to interpret the results, but can a machine effectively explain itself, its decisions, and its choices, as part of interacting with people? It's a very nuanced kind of question and it comes with some pretty severe challenges.
JPL Leadership in Artificial Intelligence
ZIERLER: In reflecting on almost 40 years at JPL, all of the discovery, all of the engineering achievements that you've witnessed and you've been a part of, if you can just in very broad terms separate out, what has AI and machine learning made more efficient that would have happened otherwise, but it was just done more efficiently, and what has AI and machine learning simply made possible that it wouldn't exist without it?
DOYLE: I'm not sure there's a sharp distinction between those. There may be kind of a spectrum. Actually I think I can give a pretty good example or response to that question. Recently we used a particularly sophisticated computer vision approach to landing on Mars with the Perseverance rover. Without getting into the fine details, basically what was going on there was that there was a pre-computed map of the landing area in terms of go- and no-go zones, safe places to land and hazardous places to land. The job of the computer vision system was, during those hectic few minutes of descent, to basically be able to track its trajectory relative to this map and, as needed—you use control and thrusters to steer towards a safe landing zone. Now, what made that more interesting is that what was really pushing the need for that kind of a precise landing for the Perseverance rover, the Mars 2020 mission, was scientific interest in landing at certain places on Mars which basically had great scientific interest. In the case of Perseverance, this is Jezero Crater and being able to look at different geological eras because they were exposed in that terrain. Everybody knew that that part of Mars was going to be a trickier, maybe more hazardous place to land, but the science imperative had become strong enough that, "Well, do we have the technology that can allow us to do that safely?"
What was used on the Perseverance rover, you can see as having been in development for probably about 20 years, at that point, but until there was an imperative to say, "We actually need to be able to land with this level of precision because of the science goals"—for prior Mars missions, that technology would have been considered too risky without the strong imperative that it's actually needed to accomplish the mission objectives. Maybe it depends on your viewpoint but I would say that it is enabling in terms of accomplishing those particular scientific goals. Without those goals, it would be considered to be on the wrong side of the risk equation. Another way of looking at it is that it wasn't that the technology had been suddenly developed overnight. It had been in development for some time. But it was only until that context for it to be needed to go after a particular mission objective that the decision was made to utilize it. So, there's always that interesting combination between risk and capability. The way to summarize it—it's more of a continuum. It's not like, "Oh, suddenly we need to land with that precision on Mars. We never thought about that before." It wasn't like that at all. We had been thinking about it for a long time. But now the context was there because of the science imperative, that this is what we actually need for this mission. And it was ready to go, and that was the good news part of it. Andrew Johnson is the person who knows more. He was the lead technologist and developer for Terrain Relative Navigation, the key component of the Lander Vision System.
ZIERLER: Is there an element of your career that might be framed chronologically where there was a certain amount of evangelizing that needed to happen in terms of getting the word out to non-computer scientists about the capabilities that might make these missions more efficient?
DOYLE: That's a good question. I'm going to give another example for that one involving science on Mars again. Some scientists look at the potential for AI to be applied for Mars and other missions sometimes with a kind of skepticism. It's understandable and I think it's natural, and it's part of a good dynamic conversation. But it can be boiled down—and this is a little bit unfair—"It's fine to use AI to traverse the surface, but I really don't want the AI messing with the science data. The science data just needs to come back to the ground for us professionals, the scientists, to then work with and interpret and look for insights and perhaps—"
ZIERLER: What would that look like, for the AI to mess with the science data?
DOYLE: For example, for AI on the spacecraft to actually be part of making decisions about what data might actually come back. Because that's always a challenge. Mars and even further out in the solar system, you come up against bandwidth limitations for communications. The sensors and instruments are very capable, and it's almost always the case that more data can be collected than can be realistically sent back to the ground. This only gets worse as you go further out into the solar system. One way to deal with that is you just sort of take a deep breath and say, "Well, it may just take a long time for the data to come down, but that's how we're going to do it."
Another way of looking at that is, well, perhaps some kind of analytics on board, whether it's based on models that came out of machine learning or are otherwise constructed, which can help recognize data that is more scientifically interesting than other data. But I would say—and again, this is reasonable—the scientists were kind of skeptical of that whole scenario, early on, until we were able to show its value. Let me describe that now. Years ago, on the Spirit and Opportunity rover, we had a capability which we wanted to try out, and it was based on change detection. The bottom line is we were able, with that capability, to recognize and actually track dust devils on the surface of Mars. Now, up to that point, most scientists had assumed that because of light time delays, dynamic or transient phenomena like that were just not going to be able to be studied in that sort of real-time mode. In fact, it was frustrating for the scientists, because sometimes randomly the rovers might be able to image a dust devil happening off in the distance on the horizon, and it came down in a data set. By the time a scientist was able to look at it, this was something that might have been days or even weeks prior, and obviously the opportunity to study it in real time was completely lost, never really available.
When we put this dust devil detection software on the rovers, it was successful at being able to, first of all, detect a dust devil as it was happening, and actually track it, and create a whole data package which included video of the dust devil traversing and other ancillary data of interest to the scientists. Instead of the after-the-fact "Oh, there was a dust devil on Mars last week," instead a whole package came back, which had been designed with the scientists. "This dust devil occurred on Mars, and here's the whole data package that describes it." It kind of put the lie to the old reasonable assumption that, "We're just not going to be able to study in a real-time mode transient, or dynamic phenomena." This software was able to do it. With that as a point of success, then that started to open up the thinking about what is the potential for having these kinds of analytics whether it's rovers or other orbiting spacecraft which can look for such transient phenomena, study them, and then send summary data packages, fully designed by the scientists. "If you were there, what would you want to know about this event as it is happening? What data would you want to collect?" Now we do that, I wouldn't say routinely, but with less controversy; let me put it that way. And people see the value of it.
ZIERLER: I've come to appreciate that at the top, personalities really do matter, and who is the director of JPL really is important in setting the tone for what happens. Have there been directors or have there been periods during your career where you felt like computer science was more or less out in front in terms of its overall centrality to JPL's mission? Or is it more of a sort of standard growing trajectory?
DOYLE: That's an interesting question. I don't know if I've ever quite asked myself that question in those terms.
ZIERLER: I'm thinking, for example, like Ed Stone and his deep interest in the Deep Space Network and how that might filter to what you're doing, or Charles Elachi's background in radar imaging. Those kinds of things where there's an area of expertise that a director brings from their own career, and how that might filter down to where they see the role of computer science at JPL.
DOYLE: I think it's a fair question, but no, I can't think of any particular really executive top down examples like that, whether it was a director actively saying something or just generally being known that the director is interested in such and such, and that influenced how computer science was taken up. I don't really recall that happening quite that way. It's more like the challenges had to do with the culture itself. Earlier, you asked me, even though I'm a professional computer scientist, did I become a kind of space scientist. The answer was yes, but another answer to such a question is, everybody who works at JPL for some period of time also becomes a systems engineer, and this is deep in our culture. Obviously that relates to what we do with space science, Earth science, astronomy, and all the rest, but systems engineering is way deep down in our self-image at JPL, and for good reasons, because fundamentally the challenge for each of our first-of-a-kind missions—well, first, it's first of a kind. We don't have a reference point to say, "Here's how you do this." And always, we're under these extreme constraints of designing the spacecraft and instruments with power limitations, communication limitations, tight volumes… Any resource that appears in an engineered system like a spacecraft, that resource is going to be in short supply, especially on deep space missions. So how do you get all the—moving parts—to work together, under such resource constraints, and accomplish these very aggressive scientific and engineering goals? Everybody comes to realize that systems engineering is key to what we do, and to our successes.
I'm going to return to an earlier comment. Part of, I would say, proper systems engineering thinking is going back to that term I used earlier, optimization. You're going to have limited resources, so how do you optimize the use of those resources to accomplish these often unprecedented science objectives? We're good at it. We've had successes again and again, finding these very intricate unprecedented solutions. Every time we land on Mars, we're biting the fingernails, because seven minutes, and we're behind the light time delay, and it's either going to work or it isn't. [laughs] We've had good success with that. Of course, it's an amazing feeling when you know it worked. But at its core, it's a systems engineering success when something like that works.
Data Science as Interagency Connecting Point
ZIERLER: Has your interface with Washington D.C. been exclusively with NASA? Or given, for example, NSF and DOE, both very interested in computation, have you had collaborations or interfaces beyond the NASA-JPL relationship?
DOYLE: The answer to that is yes, and without getting into too many of the fine details, JPL is what's called a Federally Funded Research and Development Center, FFRDC. Under our contract with NASA or Caltech's contract with NASA, operating JPL, JPL is free to pursue work for other government agencies. Often it makes perfect sense to do that. Sometimes JPL itself as an institution is contributing to national imperatives, and it's just a great thing to be able to participate in such things. In other circumstances, and this is certainly true of computer science but not just computer science, if there's, let's say, an emerging capability or an emerging technology and it may not quite fit into the way NASA does its programmatics at the moment, where they're ready to invest in that area, it could be that DARPA, say, is ready to invest in that area, and so there can be kind of a win-win situation, where we're able to support an initiative like that for another government agency but also advance capability and our understanding of such capability in a way that can come back to a future JPL and NASA mission. Those are the kinds of win-win scenarios that we look for. So, yes, that's the context in which JPL or myself personally had gotten involved in doing work for other government agencies. I think it's an effective model.
ZIERLER: In the all-important area of JPL's contributions to climate science with radar missions and satellites and things like that, what has been the role of computation and machine learning?
DOYLE: I'm going to back up from that, and I'll come back to climate science and Earth science, but maybe the best way to approach that question is to note that when it comes to AI and machine learning, the needs and the opportunities for AI and machine learning are different in planetary, versus astrophysics, versus Earth science. Let me try to unpack that. Planetary science is still—planetary science is still in its early phase of fundamental discovery. Every time we go to another body in the solar system, we're just blown away by something we didn't anticipate.
ZIERLER: The plumes of Io, for example.
DOYLE: Yes, and there's just on and on like that. Titan is such a fascinating place. Pluto just blew our minds when we got a good look at it. Planetary science is still in that sort of fundamental discovery mode. I mentioned change detection before. Change detection, basic pattern recognition or object recognition, looking for anomalies—these are the kinds of examples where AI and machine learning can really directly contribute to planetary science goals right now. Now, let's shift to astrophysics. A lot of what is going on, all the way to JWST, is we're doing these fundamental and deeper and deeper sky surveys, of really just finding out what's out there, and particularly categorizing galaxies, stars—all kinds of interesting phenomena—neutron stars, star formation, on and on. What comes to the forefront for astronomy with AI and machine learning are classification techniques. What are the different entities out there, and how do we recognize them? Now if we look at Earth science, in some ways Earth science is further along, only because we've had the opportunity to do observations for longer. We have these sophisticated climate models, where we don't necessarily have the direct analogs of such deep physical models for planetary science. Some are available in astrophysics. The challenges in Earth science have more to do with modeling, physics-based modeling, and also with uncertainty quantification. Those are also areas that computer science can contribute to, but they're different. So, it's not a one size fits all. Astrophysics has certain forefront needs. Planetary science, they're a little different. Earth science, they're different yet. But the good news is that AI and computer science, et cetera, can contribute to all of those. Not always in the same way, but we have something to say or something to contribute to all of those challenges of scientific understanding, even though those fields are at different places in their own life cycle phases.
ZIERLER: Have you been engaged in some real far-out questions about the future of space exploration? For example, if we ever get to Alpha Centauri, it's going to take us 70,000 years.
DOYLE: That's a great one.
ZIERLER: What does that even look like? Obviously machine learning and computation need to be a part of the equation. Just on a general level, have you been engaged in those sort of far-out, long-range, almost sci-fi level discussions that take place at JPL?
DOYLE: The answer to that is yes, and it's a lot of fun. I'm not the only one, but every few years, a study is done of the—usually it's called the Interstellar Mission. Alpha Centauri, maybe Proxima Centauri, is a natural target to think about. I was involved with one of those, back in the day, probably somewhere between 10 or 20 years ago. JPL with NASA's interest put together a team of different subject matter experts. I still have fond memories of being on that particular study team. I was the AI person on the team, but there were other experts for, not surprisingly, propulsion, communications, power, structures, on and on. It was truly a fascinating thing to work on. I think everybody who worked on that came away with the idea that, "We actually can do this." Of course it's a matter of cost and will, as it always is, and it might push the envelope much more than some other things that NASA and JPL are ready to do, but in terms of a technical approach, we had something that had a good feel to it.
Now, let me come back to the AI contribution or my contribution to that particular study. What it had to do with is, going to—let's just say Proxima Centauri—it really pushes the envelope of, how do you even know what you're looking for by the time you arrive there? Assuming the propulsion and the power folks did their job well, and we can actually imagine our space platform arriving at Alpha or Proxima Centauri, but at that point, you're behind a four-year light delay, so you're not going to have anything approaching useful communications. Basically the mission has to unfold on its own, so how do you know what to look for? It came down to fundamental questions of trying to characterize what the signature of life might be. Obviously this gets into biology as well. But taking some of those scenarios I painted before, if you can recognize the occurrence of a dust devil on Mars, that's actually not a life form, so that's interesting both in terms of not getting confused, but also it seems like motion of some sort can be one of those signatures of life forms, as well as other things. How do you endow the very remote spacecraft at that point with enough of the right kinds of knowledge so that, having arrived there, it can pursue these fundamental questions? Very tricky business.
To this day, I think right up to the moment, there's work that has been looking at motion and motion detection. It turns out there is some understanding of the nuances between motion that is biologically powered versus motion that is powered by whatever other natural phenomena, and there may be ways of making those distinctions. If that's so, then that's something that we should be able to capture in a machine learning approach, in a classification approach. So, it's those kinds of questions. That was probably 20 years ago or maybe more. It was a real privilege to work on that. I hope that the Interstellar mission will stay within people's interests, whether it ends up happening as part of a government initiative or maybe even a private endeavor. Things are changing now, so who knows what the answer will be, but I hope that the interest in such fundamental questions will continue.
ZIERLER: You alluded to it, and it's worth going into a little more detail—astrobiology and finding exoplanets and maybe even detecting biosignatures or technosigatures. What will be machine learning and AI's role in that world-changing discovery if it ever happens? What would that look like?
DOYLE: We're probably approaching the threshold of where we can start to image exoplanets and look, for example, for seasonal variations as they traverse their orbits. There is interest in perhaps some of those seasonal variations having to do with shifts in the composition of the atmosphere. The question everybody is interested in is, could some of those shifts, which maybe are reflected in chemistry and spectroscopy, be driven by a biosphere of that planet? That's not my area, but I think that's getting into one of those fundamental questions. Given the success with JWST and other telescopes that are still planned, it seems plausible to me that within my lifetime, your lifetime, we may get real answers to such questions. If we have good models of what would be the biosphere-originated signatures that could be detected in a remote planetary atmosphere, yeah, then as far as machine learning as a technique, it would be up to being able to model that, and then being able to look for those signatures, and dealing with the data and all the noise in the data, extracting those signals. That seems quite plausible that that could happen in, say, the next few decades. Very exciting. That's as fundamental as it gets: can there be life out there? Can we find it? Maybe we'll get an answer soon. Very exciting.
Machine Learning and the Deep Roots of AI
ZIERLER: Let's go back and establish some historical context here. As a graduate student at MIT, the discipline is computer science, but even then, in the early 1980s, you were thinking about artificial intelligence.
DOYLE: I was. Yes.
ZIERLER: What was your point of interest? How did you get involved in that endeavor?
DOYLE: That's a good question. In some sense, it's almost a little bit circular. Let me go back to the very beginning of my JPL career and how it unfolded. I first came to JPL before MIT. I had a degree in math and astronomy from Boston University. That, as it turns out, made me well qualified to work on what JPL folks call ephemerides or ephemeris work or trajectories, fine mathematical models of the orbits of planets and satellites. My first work at JPL was not AI at all. It was this pretty intense mathematical modeling. I had a great time doing that, but finding myself at JPL, I made it my business to poke around and see what else was going on. There was some early work on Mars rovers, way back. This was the early 1980s. I just became enthralled with it, fascinated. I had a notion that coming to JPL with a bachelor's degree, eventually going back to get a PhD, but that's when it started to all come together. I said, "AI is what I want to do my graduate work in." But it was inspired by basically a JPL or a space science theme, if you will, or an application. I didn't start from AI just as a discipline, but AI and its potential to contribute to what JPL was doing.
ZIERLER: Did AI feel like really the dawn of a new field? Was it mature as a discipline at that point?
DOYLE: Certainly in retrospect I would say no, but it was moving. It continues to move. The origins of AI go back farther than most people realize, certainly into the 1960s and maybe even a bit earlier. AI in the 1980s was not exactly new, but it certainly was not in the general awareness the way it is today. It has been fun watching that whole trajectory of the field. Computer science itself isn't that much older. You can go back to Turing, and you can push it back to Babbage, say, in the 19th century, but it's not that much older. Sometimes maybe that's why people don't quite put it in the same bin as, say—I was going to say engineering, but also physics—fields that have been around for a long time and have had a long time to mature. Part of becoming proficient in a field like that is, first you learn the fundamentals, the received wisdom, if you will, and that's appropriate. As an early graduate student in computer science, it wasn't even very clear what the received wisdom was yet. Although I had the privilege of rubbing elbows with people like Minsky.
ZIERLER: Wow. What did you focus on for your thesis?
DOYLE: My thesis was actually an interesting mix of physical modeling and machine learning. I wrote a program which was given somewhat stylized data in the form of observations of the externally visible behavior of simple machines, in terms of inputs and outputs—and it had a library of fundamental mechanisms. The task of this AI program was, given those observations, could it come up with a design for a device that could actually reproduce and thereby explain those behaviors? These are very simple devices like a refrigerator or a tire pressure gauge. That was the challenge statement of my thesis, and I explored how far you could go with the machine learning techniques to come up with models of basically composed mechanisms that could make slightly more complicated machines that then matched the observations of the external behavior of an actual machine, an actual simple machine. That was my thesis.
ZIERLER: Were you oriented toward JPL? Did you see your thesis work as the stepping stone to get to where you wanted to be at JPL?
DOYLE: I wouldn't say it was strongly referenced to what I could imagine the work at JPL might be. It wasn't irrelevant, because certainly we're talking about machine learning now, so it certainly wasn't irrelevant in that sense. But no, I didn't imagine it as something that, "In the next ten years I'm going to keep working on this and it's going to be something JPL will take up." No, I didn't quite think of it that way.
ZIERLER: What was your first job at JPL?
DOYLE: As I mentioned earlier, it was the work on modeling the orbits of planets and moons.
ZIERLER: This is post-graduate?
DOYLE: Oh, post-graduate.
ZIERLER: Right, after MIT.
DOYLE: After MIT, what was my first job? I landed pretty early into the first level of line supervision at JPL. I basically became what JPL calls the technical group supervisor, of the artificial intelligence group.
ZIERLER: This did precede you? There was a group there already?
DOYLE: There was a group there. That's the one I had learned about involved with doing some of the early Mars rover work.
ZIERLER: Do you have a sense of the history, how far back that goes, or who had the vision to get it going?
DOYLE: That particular group went back, late 1970s, or early 1980s, I believe.
ZIERLER: Was there a mission that necessitated it, like Voyager, for example?
DOYLE: No, I couldn't put it that way. It was seen more as pretty much a research-oriented group, exploring solutions for the future.
ZIERLER: Did it recognize that data acquisition was becoming so large that there would need to be—?
DOYLE: No.
ZIERLER: That's too far afield?
DOYLE: Back then, that was not a burning issue at all.
ZIERLER: What was the cause, then? What triggered the need for AI?
DOYLE: It was exactly what I had picked up on. It was the potential for doing future missions with rovers, or mobile robots on Mars, and all that would entail.
ZIERLER: So that's how far ahead JPL's thinking is. It's the late 1970s and they're thinking about rovers 30, 40 years in the future?
DOYLE: Absolutely, yeah. You can definitely follow that thread. The artificial intelligence group had just been born at that time, and then I was privileged to become its next technical group supervisor.
ZIERLER: Was there an independent data science group, or was that all rolled into one?
DOYLE: No, "data science" wasn't even a term that was used back then.
ZIERLER: When does data science enter the mix?
DOYLE: I think it's surprisingly recently. In fact, "data science" was preceded by another term, closely related, in usage at least, if not in precise definition, and that was the term "big data." That started coming more into awareness I would say in the early 2000s. Then somewhere in there, it sort of morphed into data science. That made it sound more like a proper discipline, which is a fair thing.
ZIERLER: [laughs] The question that I applied to AI, it sounds like it's more related to data science, where there's this recognition that there's this huge amount of data that needs to be managed and maybe AI is a tool to help manage it? Is that a way of thinking about it?
DOYLE: Yeah, when people started worrying about big data, it was the recognition that our ability to collect data was beginning to badly outstrip our ability to usefully work with that data, analyze it, reduce it to understanding. That all started to come together, from my perspective, like in the early 2000s, and then up to 2010. Somewhere in there, the term "big data" which is just a statement of the challenge, started being replaced with the term "data science" which is more of an indication of a solution.
Computer Science and Mars Exploration
ZIERLER: To go back to your initial appointment in AI post graduate, thinking about rover missions on Mars, the manned missions on the Moon, did they provide a useful reference point at all? Or because by definition the Mars program was going to be unmanned, it necessitated a starring role for AI that simply wouldn't have been necessary even if it was contemplated for Apollo?
DOYLE: There are a few questions wrapped up in that. One is the dynamic between human space flight versus robotic spaceflight. The other is at what point did—or did AI become recognized as a more core possibility for finding solutions to the current set of challenges. Human spaceflight versus robotic spaceflight, that has been an interesting dynamic within the NASA family all along. Arguably JPL has been in many ways the leader for robotic spaceflight. Both paradigms are powerful, and ultimately it is about sending humans to these destinations, including someday Alpha Centauri, Proxima Centauri. That's the only angle that makes sense. But on the way there, much can be accomplished with sophisticated robotic spacecraft, and thinking about the role of AI and machine learning including all the way to the possibilities for these techniques to analyze science data that is being collected in a remote place. All of that is relevant. Often it gets framed in terms of either/or, or some kind of a tension, but I've never really thought of it that way. It's just different paradigms.
With human spaceflight, one of the challenges that I think is getting a lot of attention, particularly at Johnson Space Center and the other human spaceflight centers at NASA, is the whole human/machine interaction dynamic. There are some interesting and intricate questions there. Maybe this is a good time to come back to a term that I used before—"explainable AI." Fundamental to effective human-machine teaming is this notion of trust. Does the human have confidence in what the machine is doing, and the decisions it's able to make, the actions that it takes? It's a pretty fundamental question. The same question comes up in different forms, say like in Space Force or in DoD contexts. In fact, it might be even more central there in terms of the scenarios for human-machine interaction. But just staying in the NASA context for now, human life is involved. We're sending humans to dangerous places and having the machine there can extend what can be accomplished on the mission by the human and then just generally by the mission. But how does the human build confidence in the machine?
Some of the interesting research that's going on in that area has to do with, can the human and the machine have a kind of shared mindset about what the goals are, so that whenever the machine makes a decision or takes action, if the machine can reference those to how that will contribute to accomplishing one of the given goals or mission objectives, that's one way to build confidence that the machine is doing something reasonable. Another is just flat-out experience. The more experience you have with observing what the machine does, even if it surprises you sometimes, if it's able to explain why it did that, you say, "Well, I might not have done that, but that's an actual rational choice given the circumstances, and in the context of what the mission is trying to accomplish." Those are high-level ways of how to build trust between human and machine. But that whole area is still—it's kind of early to say that we've been able to build a machine that can explain itself. To the extent that AI has been coming on, awareness and interest stronger and stronger, I think that's going to be pretty critical to future success. People will not be comfortable tolerating machines that don't explain themselves, or if there's some reason why they're not going to be able to explain themselves. I think that's one of the big, looming research issues facing AI, and that's not unique to NASA; that's pretty fundamental.
ZIERLER: That's society-wide. To go back to the initial appointment, when you started to think about applying AI to the challenge of getting a rover on Mars, what was the game plan? What could you offer? How did AI make the Mars rover program feasible in a way that simply might not have been?
DOYLE: Mars rover missions have lots of interesting challenges, but the one that grabbed me early was the whole business of traverse planning, where the rover on its own can go from point A to point B. The way those algorithms work now is that with stereo vision capability onboard the rover, it's able to build up a map of the terrain, locate obstacles, and compute onboard possible paths to get from A to B—and execute them. That actually works pretty well now. For a long time, people weren't ready to trust that. But what changed the dynamic is that it just became too tedious to continue to try to operate the rovers in sort of a joystick mode. Each day you'd go a little bit and see where you ended up, and then the next day you plan a little more—it just became too tedious, particularly for the scientists. Again, there were people that were working on the algorithms, on how to do the autonomous traverse, and by the time the scientists and other mission personnel said, "Okay, I think we're ready, we really want to try this now, it has just become too cumbersome to do everything by joystick," the solution was pretty much ready to go. Now, it works routinely, and all moves forward. That mobility or traverse question was one of the first things that I was interested in, and saw where AI could contribute. It took a while, [laughs] but that is how things play out.
ZIERLER: Looking at the 30- to 40-year plan to get to Mars, so moving into the 1990s, what were some of the things that you were working on that might suggest even in today's future where JPL is headed next? For example, icy worlds and those kinds of things, did you get involved in planetary science beyond Mars?
DOYLE: Yes, I did. In the 1990s was when we were doing the early work on what we would now call onboard science analytics. That was, for example, the work that led to the dust devil detector on Spirit and Opportunity. We're still working on expanding the use of onboard analytics. You're asking me, what have we worked on recently that may pay off in the future?
ZIERLER: Yes.
DOYLE: Now I want to turn to something more recently that I was involved with, and it was right here on campus. This was one of the Keck Institute for Space Studies workshops. The question framed for that particular workshop—and I ended up being one of the leaders—the question was framed in the following way. Most people today are familiar with the term "the cloud" or "cloud services," and this question came up in the context of JPL and NASA missions. Cloud services, that's all well and good. You can imagine how Earth-observing missions might be able to access the cloud and laverage that kind of access to deep computation, but that's not really going to work in the outer solar system. This workshop was called "Nebulae"—the astronomical variation on clouds—that's how it got formulated. We asked the question, "Would it be possible to deploy some form of cloud services even deep in the solar system, and what would that look like?" Or is that just too much of a technological reach, and the most we can hope to do with cloud-type services would be Earth-orbiting, maybe cislunar, and some at Mars. Because even today, we see the use of relays at Mars, using multiple spacecraft. An orbiting spacecraft by definition is out of the direct line of sight with Earth up to half the time, but with relays, we can have pretty much on-demand communication with any spacecraft at Mars. So we've already started to put the lie to—there are at least some possibilities for deploying those kinds of services even deeper into the solar system.
Let me get back to your question now. I think one of the holy grails, if I can put it that way—if we could deploy such capability deep in the solar system, what would be the payoff in terms of missions and the ability to do interesting science? When you get out to Jupiter, Saturn, and farther, access becomes really difficult. The way that plays out is that you go to an interesting body or system—Jupiter, Saturn, you get your first look at Titan—you get excited, and you realize, "Now we know what questions we really want to ask." The problem is, the next mission which will have a different set of instruments designed to go after those more refined science questions is probably going to be 30 years in the future! That's the reality. Think of that from the viewpoint of the career of a young scientist.
ZIERLER: It's generational transmission of knowledge.
DOYLE: And gives a scientist pause. "Do I want to invest in that, 30 years before I know I've got something? That's basically my whole career." That's risky, just in terms of a career arc. Coming back to this Nebulae workshop—this wasn't how it was originally formulated, but one of the interesting scenarios that emerged is, if we can continue to deploy capability to remote parts of the solar system, maybe a smart way to use that possibility is to not even know what the future mission is going to be, but just keep putting more capability out there, in terms of raw computing, data storage, networking, ability to communicate.
ZIERLER: You're not constraining what the capabilities are.
DOYLE: Then you send your reconnaissance mission to, say—I'll just pick Neptune or Triton. That's of interest. It's just the nature of things that when you go there, you're going to make—back to planetary science still being in the fundamental mode of discovery, you're going to learn some things that are going to blow your mind, and that's going to motivate the next set of science questions. But instead of having to wait for 30 years for the next mission to now do the real Triton mission, we're incrementally deploying capability out there all the time, so all we need to do is refactor it, or reconfigure it, or reprogram it, for these new science questions that now we know are the right questions. Now, that's easier said than done, right? And that's kind of a vision statement, the way I'm describing it. But that ended up being some of the exciting output of that particular workshop. It boils down to, if you continuously deploy capability to remote places, then you can redefine the mission as you go, as you learn, as you gain insight, and just keep tweaking the mission that you want to accomplish there, given this sort of raw additional capacity that is being provided by continuing to deploy platforms out to this remote part of the solar system.
Again that goes back to that dynamic between optimized and generalized solutions. Under the optimized solution, eventually you get stuck. You can do the Triton mission and beautifully optimize a reconnaissance mission at Triton—optimize—but now you've get your new set of science questions, and you've got to wait 30 years for the new optimized next science mission at Triton. If instead you deploy generalized capability in terms of computing, data storage, and other resources too—obviously you have to address power, communications—then you're more ready to go for the next mission you want to accomplish there. If you've done it right and done it well, maybe it's just a matter of reprogramming and tweaking, which we can do remotely. You can't change the hardware remotely, but you can reconfigure the software. I find that to be an exciting vision, and that's probably the most intriguing output of that particular workshop. I would point to that as an example of something we've done just recently that could really pay off in the future.
ZIERLER: Has this borne out already? Can we point to examples where—?
DOYLE: No, it's too early. We're still in the mode of getting the word out, as a vision statement, and rallying excitement and support for it. I already tend to think of it as—it's a compelling vision, and I think it has enough merit that we will get there. It's not clear when exactly and how, but I think it's a pretty good take at what the next paradigm in exploring the solar system should be. Otherwise, we just get stuck. Eventually, 30 years, and then it's 50 years, and finally the will is not there to do it.
The Advent of Astroinformatics
ZIERLER: I want to move to, of course, the topic that brings us together today. Tell me about when you first met George Djorgovski. What was he after? What could you offer to help him achieve what he was looking to do?
DOYLE: Meeting George was definitely one of those great pivot points when I look back over my career arc, because we had this wonderful alignment. That's always the basis of a productive research collaboration, where you get different perspectives but you get alignment in terms of what you want to do next. George was very much involved with the Palomar Observatory activity to generate the next sky survey. This was back in the early 1990s. We didn't use that term then, but it was an early example of a "big data" challenge.
ZIERLER: This is the advent of digital detectors.
DOYLE: Yes. It was the POSS-2 survey. They knew they were going to be collecting lots of these photographic plate images of the full sky with more resolution than had existed before. George posed what on the face of it sounds like a very simple question. "We would like to, as sort of a starting point for working with this new data set, just understand how many stars have been imaged, how many galaxies have been imaged, and not get them confused." [laughs] As a simple statement of purpose, it's easy to describe, but in terms of how do you accomplish it—because it turns out that when you get down to the level of the capability of Palomar and its ability to image faint objects, yes, there are things that are clearly stars, and there are things that are clearly galaxies, but there's a huge, gray, blobby area in the middle where a human, even an expert, can look at the images and it's not very clear. George—"Can machine learning help?" It was just a question thrown out there. I was still a fairly newly minted group supervisor and was still in the mode of hiring new PhDs. One of them turned out to be a really capable guy, Usama Fayyad, a machine learning expert, who came out of Michigan. It became, "Let's tackle this," and he became the point person. He approached it with what is now considered almost an antiquated technology in machine learning, decision tree analysis.
ZIERLER: What does that mean, decision tree analysis?
DOYLE: There's a set of features that you're going to use. You've got your stars, you've got your galaxies, and with expert astronomer input, you come up with a set of features to describe the objects, like extent, or whether there's anything that can be defined as an axis. Overall brightness. I don't remember what the exact features were. But we came up with a small set of features to describe these things, with the aim of, can we come up with a way of distinguishing between stars and galaxies. Decision tree analysis is very easy to describe. It follows a tree structure. At the top, it might be "less than this brightness, greater than this brightness," and then it creates a branch in your tree. Then you branch on a different feature like "overall extent, less than four pixels versus four or more pixels." I'm making this up but you get the idea. You capture all that in a tree structure. What you're analyzing is the ability to discriminate. You get experts together to create a so-called training set. "We're very sure these are stars, and we're very sure these are galaxies." Now, can you come up with one of those decision trees that given a different data set, not the one you trained on but different data, can it reproduce the human expert results, and if so, then you're ready to apply it to other data, and eventually the whole data set. That's basically the machine learning, supervised learning paradigm, as it is called. The bottom line is we had great success with that approach. Pretty soon we were able to just go ripping through the data set, that Palomar data set, and continuing with expert checks and balances, verifying the work, we were able to, in a very efficient computational manner, go through that whole data set and distinguish the stars and galaxies. Which would have taken astronomy graduate students much longer.
ZIERLER: It's tedious. It's not a great use of their time.
DOYLE: Because it's tedious, the possibility of making errors is always there, just because of human fallibility. But the machine, once it has got its decision tree algorithm, it's just going to crank on that. It's not going to make errors unless the decision tree itself is flawed. That ended up being a great success. It got written up in both the machine learning and the astronomy journals. We were off and running. That was the initial collaboration with George.
ZIERLER: In your recollection, was this the origin point of what would become the National Virtual Observatory, those kinds of institutional endeavors?
DOYLE: That's probably a better question for George, or Tom Prince. Certainly I know the NVO. As to how one led to the other and what that path looked like, I was just not as close to it. But our particular project—we called it SKICAT—was quite visible in the astronomy community, and of course George was there to champion it further. How directly or not that led to things like NVO, I'm not sure I can answer that. NVO represented an early example of an event detection and response architecture. The software detects an interesting event, and immediately attends to follow-up observations, which may utilize AI planning techniques. The canonical astronomy case is a supernova. NVO is ground-based, but the same architecture is at the heart of some of our onboard science analytics, such as the Mars dust devil detector, also natural catastrophe monitoring in Earth observing, volcanic eruptions, floods, sea-ice break-up, wildfires, and the like. All that followed, but I can say the astronomy community recognized the power of machine learning-based classification techniques and continues to use them to this day. In that sense—I'm not going to say they're "ahead of"—but that was a particular track of success that was available to astronomy which we now see in planetary science and in Earth science. To their credit, they really made the most of it, the astronomy community.
ZIERLER: More broadly, did JPL have institutional connections with Palomar that made this easy to wrap up in your portfolio? Did you do a detail or whatever you call it? How does that work just in terms of administrative responsibilities?
DOYLE: From the JPL perspective—
ZIERLER: Or is it just curiosity-driven research, and "go for it"?
DOYLE: At that time, because the AI and the machine learning efforts were still pretty nascent in the JPL context, we effectively sort of enjoyed a kind of—
ZIERLER: Free rein?
DOYLE: I wouldn't say it that way [laughs] but some independence, in terms of we had a budget, and we had some freedom to define the research problems we were going to tackle. That was one of them, and it paid off, so obviously it built on itself from that point. As far as the connections to Palomar, I think those basically came through Caltech. I did get to go to the Observatory myself.
ZIERLER: Oh, you did?
DOYLE: Which was a huge treat! It was great fun. I got to stay overnight, pretend I was an astronomer. I don't know if you've been there, but there's this interesting moment—I don't know if this is true anymore—but to actually step into the observing cage on the 200-inch, there's this moment when you're going up this sort of cherry-picker-like contraption and approaching the observing cage, and there's this moment where you're literally stepping out over into open space to get from one to the other. If heights are a problem for you, that's an interesting moment. I was okay with it, but I still took note of it. You're stepping out, and you're 50 feet off the ground or something like that.
ZIERLER: Because this collaboration did make quite a splash, that both the machine learning community and the astronomy community took note of just the potency of this combination of disciplines, was that useful for you bringing these capabilities back to JPL? "Hey, look what we did for astronomy; we can now do this for x, y, and z"?
DOYLE: I would say definitely yes. It wasn't, say, focused on astronomy as an application, but it was the power of machine learning and by extension AI. That got noticed, all the way up to Ed Stone, actually. So our group was in good stead at that point, and we were able to lean into the future. Those were probably the earliest days we started thinking in terms of what we now would call onboard science analytics. This was not actually an example of that because it was all done offline with a data set. It didn't involve the spacecraft at all. But that was some of the origins of that kind of thinking. Then eventually it led to, as I mentioned, the dust devil detector, and the work continues to this day. So, yes, that was a pretty important success.
ZIERLER: Now, did you stay involved with other digital sky surveys after Palomar?
DOYLE: Me personally, not so much. Our machine learning groups did continue to do work in astronomy, and in many other areas as well. I'm trying to think, did we ever work with a particular sky survey set again? They don't happen that often.
ZIERLER: Sloan came after. Zwicky came after.
DOYLE: Thank you, yes. So, yes. Now, roll it forward to say the 2000s, our machine learning—our effort grew over time. It had originally been just the AI group, and then there was a machine learning group that split off. Today, we have at least two machine learning groups. One of those did get involved with the Zwicky Transient Facility, for example, and I believe continues that work to this day. I'm not as close to it as I used to be, obviously. So, yes, there's a thread you can follow that stayed in the astronomy arena, starting from the original SKICAT work.
ZIERLER: I want to capture your perspective on this basic narrative of the value of machine learning for astronomy, as it exists from the interplay between sky surveys and much more focused, more powerful, high resolution instruments like Hubble or JWST, things like that. Basically, as I understand it, the basic setup is, the sky surveys operate at a lower resolution, they take a broader picture of the sky—
DOYLE: That's right.
ZIERLER: —and that creates just an enormous amount of data. The challenge at that point is picking the signals out from the noise. I'll stop in the narrative there and just ask you to flesh that out. Give me your perspective on how all of that works, what it means for all of this data to be generated.
DOYLE: From that original Palomar survey, the resolution and the quality—well, it's quite different, in terms of wavelength and everything else than, say, what JWST accomplishes today. But that whole parade of more and more capable telescopes, it's just amazing what they can image. I mentioned the ZTF, the Zwicky Transient Facility. That has probably fairly direct heritage from the Palomar and the SKICAT work. Now, the objective there is a little bit different. They're looking for—the holy grail there is near-Earth objects, particularly ones that are more in the glare of the Sun and are harder to detect. Fundamentally, it's doing a kind of signal processing or image processing and detection that is similar to what was done in the original Palomar Survey. As we go forward to JWST, now the possibilities are broadening. There is still fundamental object detection like exoplanets. That continues. But as we were talking about earlier, we're going beyond that. For example, being able to detect spectroscopic signals, seasonal variations, at exoplanets. That is taking a step beyond anything that we ever tried to do with the Palomar Sky Survey. The sky is the limit, I guess is one way to put it.
ZIERLER: Because Palomar is literally looking at everything, what constraints do you put on the machine learning so that it identifies anything that might be of interest? Comets, asteroids, exoplanets, supernovae, transient events. What does that look like?
DOYLE: That gets into the spectrum of different machine learning techniques. The Palomar work was a very basic approach to what we would call classification now. Again, the problem posed was very simply stated: distinguish stars from galaxies. We were able to accomplish that. But it begs a different kind of question. That's called supervised classification, where there are objects that you already know you're interested in, and a human expert does the original work to say, "These are examples of object A, object B, object C," whatever it is, and then the machine learning algorithms do their statistical analysis and come up with a compact model for how to distinguish among them. That's the power. But it begs a different kind of question, which is, how do you find something interesting that you didn't already know about, that you didn't even know you were looking for?
ZIERLER: You don't want to constrain yourself away from that.
DOYLE: Yes. This gets into what is often called unsupervised classification, or sometimes it's called anomaly detection or outlier detection. These are different approaches, which aren't necessarily trying to recognize things that you already have a model of, but to look at the data, particularly a new data set, in a raw sense, and say, "What are the natural categories within this data set? How many different kinds of objects are there in this data set?" There are powerful, again, statistical techniques for doing that kind of analysis. Where it gets interesting is, when you find the natural divisions in the data, if one of those categorizations is something that you have no description of, something you haven't even seen before. That's not so much classification; that's starting to get into a different mode, a kind of discovery. Find the outliers, the things that we didn't know about before. This can apply to planetary science as well as astronomy. That's an exciting area. Since discovery is kind of the core objective of all the science that we're trying to do, that can emerge as a very powerful technique.
ZIERLER: All the data, all the noise, when the machine learning finds a signal, what does that look like? Does an alarm go off? How do humans get alerted that there's something that bears further investigation?
DOYLE: Usually that kind of a system wouldn't generate alerts in that sense. It would do its analysis of the natural divisions in the data, and then a human would come along and inspect. Now, to give an example of how tricky that work can be, those algorithms can work—for example, you can say, "Find me eight natural divisions in this raw data set." Then you come back and ask it, "Now find ten natural divisions in this data set." From a statistics viewpoint, those are well-formed questions, and the machine can do its operations and come up with those divisions. But the machine doesn't have any insight into which of those distinctions are interesting or fundamental or are exciting. It's going to take human inspection. So the scenario of a machine raising alarms—"I found something, I found something"—it probably doesn't work that way. It's more the machine doing this deep form of statistical analysis and then the results being presented to a human, and the human finding significance, or not, in what the machine came up with.
ZIERLER: What's the mode that gets from the machine to the human? Is it a computer screen? Is it a printout? What does that look like?
DOYLE: It's not one thing. Usually it would take the form of—we found let's say ten different classifications for the data, and in these ten categories, here's what a typical object in each category looks like. That's how it would get presented to a human. That could be a list of attributes, something very dry, tabular, or it could be an image, sort of a blended image description of that particular collection of features. Then it's up to the human to say, "That's interesting," or "That isn't interesting," or "We've never seen that before. I want to investigate further."
ZIERLER: When you cross that threshold, when the machine has found something that warrants human investigation, and the expert says exactly what you did —"Let's look at this further"—from there it would go to, as I mentioned, a higher resolution, more focused instrument like Hubble, Keck, what have you. Are you involved from that point forward? Is there a machine learning component to taking this very specific target and putting it into this telescope so that we can really zoom in to see what we're looking at here? Are you involved in that latter stage of the process?
DOYLE: Perhaps not immediately but let me unpack it a bit more. One legitimate response to having found a potentially new category would be, "We want to do higher resolution images on these objects." But before that, there's probably going to be some kind of peer review. It's great for one researcher to get excited about, "I think we may have found something interesting in this data set," but there are probably going to be some rounds of checks and balances that occur, with other experts looking at it and either agreeing or not agreeing that it's interesting or not. If it passes those kinds of tests or verification, then you'd get to, "Okay, now let's go image some more, and let's try to understand what this new kind of object is." With more resolution or different kinds of observations, whatever it might be. That's kind of how that process would play out. But that's how science gets done.
ZIERLER: That's right. Going back to your undergraduate interest in astronomy, what was most meaningful for you to be involved in this discovery? What did Palomar and its partnership with you and machine learning discover that launched astronomy forward, that you're proud of or excited that you had a role in?
DOYLE: That's a good question. I guess what I found truly exciting, perhaps a little unexpected, was the unabashed interest that I saw coming out of the astronomy community. I'm not saying across the whole community, but the people who picked up on it and started writing their own papers, as astronomers—
ZIERLER: Now that they see what the capabilities are.
DOYLE: —to build on what we were doing, that was very gratifying. Because it starts to shift that dynamic I mentioned earlier, where sometimes scientists are a little bit like, "Oh, don't touch my data." But when they could see the power of the techniques when used well and thoughtfully and then they start to run with it, that's a success.
ZIERLER: That's really being part of a movement.
DOYLE: Yeah, that's having impact. At that point, who even cares about the credit question—"Just run with it! It's working! Knock yourself out!"
Bringing AI Across the Fundamental Sciences
ZIERLER: The other thread that is interesting to think about in historical terms is that one of the big meta-narratives in science now is just how computational all scientific disciplines have become. Astronomy, as I've come to appreciate, is in many ways first in among the fundamental sciences. In the way that now, for example, biology is so computational—
DOYLE: Oh, right, yeah.
ZIERLER: —and thinking about CRISPR and the Human Genome Project, things that could never—
DOYLE: Protein modeling and all that.
ZIERLER: —exactly—never could have happened without this big data revolution. I understand with astronomy being first in, other scientists and other disciplines—first it was what you and George were doing, and other astronomers got excited as a result of that. But then beyond astronomy—chemistry, physics, biology—were you involved more generally in bringing these capabilities not just back to JPL and planetary exploration, but to where we see science in the last 25 years? What was your role in those developments?
DOYLE: That's a great question. In my case, I did stay fairly close to the JPL envelope, if you will, which is fundamentally space and Earth science and its various sub-disciplines. But I believe you have or will be talking to Dan Crichton.
ZIERLER: I did, yes.
DOYLE: He has gone big-time into—
ZIERLER: Oh, early cancer detection and things like that, right.
DOYLE: —NIH/NCI and—yeah—biomarkers. That's a great success story but that's for him to tell. That's a great example of the power of these techniques to have great impact, and also illustrates the principle I mentioned earlier about the cross-cutting nature of these techniques. That if you get a powerful solution in one sector or one domain, with a little bit of good thinking and refactoring, you can transfer that capability into another domain. In that example, the same kind of imaging techniques that allowed us to find features on the surface of Mars might be used in histology, the staining for contrast that happens on slides in biology, and looking for whatever features that might be relevant to, say, cancer biomarkers. It's fundamentally the same imaging-type techniques, image analysis, and then finally the machine learning analysis of the data. That's very powerful and exciting. I personally haven't worked, say, in biology or chemistry, but there are all kinds of possibilities there.
ZIERLER: Moving our conversation closer to the present, now that we're in the rover era, from the late 1990s, early 2000s, given that you were so involved in the technology that allowed this to come to fruition, when it came time for actual launch and landing, what was your role as these events were unfolding?
DOYLE: In the case of Perseverance, by then I wasn't actively working on it.
ZIERLER: Is that simply because the work was done, that you had done what needed to be done?
DOYLE: My contributions were earlier when the technology was being developed, helping it get funded, et cetera. I had the great gratification of seeing it be successful, but I wasn't one of the hands-on people in the operations room at the moment of success. But that didn't make it any less gratifying. One thing I've learned in a 40-year career arc is delayed gratification. [laughs] Especially working in technology, it can take 20 years, and that was one example of that. But maybe that makes it even sweeter at the end, when you have that kind of success and that kind of impact.
ZIERLER: For the last 10, 15 years at JPL, what were some of the big things that you worked on?
DOYLE: I would say about 10 or 15 years ago is when the work started to shift from more focused on AI and machine learning to more of the data science, which of course are related. The Mars stuff continued, and then I got more involved with some of the Earth science applications. One of the more recent successes we had in the Earth science arena, which was quite exciting, is one of our data science activities worked with hurricane data. This was actually in collaboration with the National Hurricane Center. They were kind enough to provide some data, and one of our researchers was able to, with machine learning techniques, improve the prediction of, when tropical cyclones form, which can be the advent of hurricanes—the predictive ability in the early part of that life cycle on which storms were going to become severe and which ones were not. We applied machine learning techniques to historical data, and we were able to come up with—I could go back and get the exact, or even produce that paper—but it was on the order of upping the predictive capability from something like 40% accuracy to about 70% using these machine learning techniques. In collaboration with the National Hurricane Center. That was a pretty significant result. Because hurricanes, they seem to be only getting worse, right? And more frequent. It's a big concern, obviously. Being able to enhance the predictive power there is pretty important. That actually got attention all the way up to the Earth Science Division at NASA, which was gratifying, and the NHC people were very pleased. So, yes, that's bringing it back into a more recent Earth science application. Another one had to do with—this is often on people's minds these days, too—still in the Earth science arena— airborne flights looking for large methane emitters.
ZIERLER: Absolutely crucial for climate mitigation.
DOYLE: Again, we had a data science pilot which involved being able to work with the data while the airborne flight was underway. An airborne mission would happen over, say, a couple of weeks. We were able to, using a form of this cloud service, cloud techniques, analyze the data as it was being collected, and able to gain insights such that the path of the airborne mission could be modified to zero in on large emitters even while the flight was underway. In the past, all that would get done offline, so you'd have a two-week flight collecting data but no insight, and then you'd go offline, the flight was over, and then you'd find after the fact where, say, the big methane emitters were. We were able to streamline the processing of the data and the analysis of the data so that they could focus in on the big methane emitters efficiently. That got a lot of attention and a lot of kudos, too. As you just noted, that's a big area of interest right now.
ZIERLER: That's not even such delayed gratification. It's happening now.
DOYLE: Yes. That's literally for the whole planet, too, so that's very gratifying.
ZIERLER: Now that we've worked right up to the present, if I may, I want to ask an overarching, retrospective question about your career and then we'll end looking to the future. If I can put you in the mindset of graduate school when you were really starting to think about AI, if you can think back to then and imagine what career might unfold, what has surprised you the most, and what has more or less unfolded how you thought it would, with regard to what AI would be capable of contributing?
DOYLE: Whether it's AI people or computer science people, I think technologists in general are pretty poor at predicting which technologies are going to mature, at what pace, and when. If you roll it back 20 years and recall, "This was supposed to be just around the corner," and we're still working on it, and here's something that we thought was decades away and now it's already working really well. We're not very good at predicting that kind of thing. I can just give a couple of examples of where those things were surprising. I guess I have found it—surprising is one word; maybe even frustrating can apply—that it continues to be a tough go in a cultural sense to bring together those two viewpoints of engineering optimization and computer science generalization. I understand the reasons why JPL's deep culture and self-image lands more on the optimization side.
ZIERLER: Because of the systems engineering?
DOYLE: Because of systems engineering, deep in our core self-image. It's perfectly understandable, but it's still—how would I put this? I'm a believer in—whether you want to call it software, AI, machine learning, digitization, data science—that is the future. That's where the fundamental new capabilities are going to be coming from. It seems almost obvious to me that this applies even more so to the kinds of deep space missions that JPL is known for. As I mentioned before, you can't get the hardware back, but you can update the software, so we should be always thinking through that digital lens of how we will accomplish the future missions. But I find that we don't, not enough. We reference techniques that have worked well in the past, absolutely excellent systems engineering, and that notion of optimization, which come from accepting the first-of-a-kind challenge. So I understand why it's there, but I feel passionately that a shift does need to happen towards more of the software or the data and computer science, or the digital perspective, to continue to be a leader in this area. I mentioned the example of, wouldn't it be great if we can deploy general capability and then reconfigure it for new missions that we aim to do, as we gain insight into what those missions need to be, rather than start from step zero and plan a 30-year life cycle for that next mission. I think all these things are possible. But that has been a tough nut to crack, and it's more cultural than anything else. The technical challenges are there, and they're—
ZIERLER: It's still people, at the end of the day.
DOYLE: It's still people, at the end of the day. That's something that is still just sitting there, and needs work. I'll [laughs] continue to contribute, as I can, but others will pick it up. I do feel that JPL, NASA by extension, really has to get that right, to allow us to continue to keep pushing the frontiers of exploration in space. It's almost obvious to me, but it hasn't clicked yet.
ZIERLER: Looking to the future, a theme of our discussion of course has been all of the positive ways artificial intelligence and machine learning has pushed forward scientific discovery and all of the positive effects this had netted. As we look to the future, as the technology increases, to shift the perspective a little bit, what gives you pause? What are the things that we need to be cognizant or even careful of, as AI develops, both in a scientific realm and in a public policy realm?
DOYLE: AI is getting a lot of attention these days. I mentioned ChatGPT early on, because it's very topical right now.
ZIERLER: In the news every day.
DOYLE: It's kind of out there. Let me respond in terms of my own limited experience with ChatGPT. In my retirement phase of life, as you know I continue to stay engaged in certain ways, but I have some new endeavors going which have to do with creative writing. I'm very excited about science fiction, of course. I'm working with a collaborator, where we're working on scripts for a television limited series with a time travel theme. We got a little bit stuck on coming up with what Hollywood calls a tagline, which is this very pithy, eight-or-ten word sentence about why somebody should be interested in your TV series. What's the hook? What's cool about it? Because it's such a short sentence, it's hard to get it right. My collaborator and I, we were going back and forth. "That's not quite working. We don't have it yet." Then ChatGPT appeared. We said, "Let's see what ChatGPT has to say about it." So we gave it not a one-liner, but a short paragraph of what our TV series concept was about, and asked it to generate a tagline, which apparently is something it does. We got the usual output of ChatGPT to such queries — it gives you a list of 10 or 12. Some of them were just off, but a couple of them were interesting enough and worthy enough and surprising enough, but not that we would just grab them wholesale and say, "Aha, that's it. That's the one we were trying to find."
ZIERLER: It's a kernel.
DOYLE: It's a kernel, and it got us off—we were stuck, in a certain part of the space, and we couldn't climb out. We had gotten ourselves into a blind spot, and ChatGPT generated a couple ideas that were different enough that we said, "Ah. We didn't think of that." But then we ran with it. We didn't just take what it gave us, in terms of the text, but it shifted our thinking into a different part of the space. Then we got unstuck, and then we came up with a different tagline which we were happy with. My point is that ChatGPT proved to be useful in terms of that give-and-take interaction and nudging when we were experiencing a blind spot. That's one data point. I think it can have a worthy use like that. On the other hand, I have a sense of its limitations, and how people can attribute more capability to it than is deserved at this point. But for that kind of narrow use, where it can be part of a give and take, when you're stuck on something, especially something creative, where you're stuck cognitively and you just need a kick from a sideways direction, it worked in an interesting way, and maybe better than I might have expected. That doesn't mean we should just outsource or genuflect to it or be awed by it, because other outputs on that list were not very good at all. I don't know, it's perhaps in human nature, that we want to be more impressed or more trusting than maybe we should be. That's something to worry about, because that's an example of an AI capability that does certain things, but there's other things it doesn't do well. That in itself isn't either good or bad, but it's how it is presented and how we react to it. That's where it gets tricky.
ZIERLER: We would do well to remember that it's a tool, and a highly flawed tool.
DOYLE: Yeah, which can contribute in a context, but not where it's the authority.
ZIERLER: I want to thank you for spending this time with me. It has been a great conversation, wide-ranging, and really useful for our project on DDA, so I want to thank you so much.
DOYLE: I'm very glad to participate and contribute.
[END]
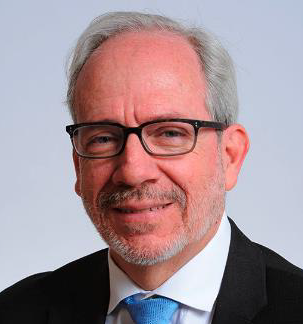
Interview Highlights
- Space Science Via Computer Science
- JPL Leadership in Artificial Intelligence
- Data Science as Interagency Connecting Point
- Machine Learning and the Deep Roots of AI
- Computer Science and Mars Exploration
- The Advent of Astroinformatics
- Bringing AI Across the Fundamental Sciences