Roarke Horstmeyer (PhD '16), Computational Microscopist and Biomedical Engineer
Over the past ten years, the breakneck speed in advances in machine learning has prompted a simple and important question: does this improve the lot of humanity? Roarke Horstmeyer's research, at the interface of optics, biomedical engineering, and artificial intelligence, offers a powerfully resounding answer in the affirmative. With his dual focus on developing cutting-edge instrumentation, and in optimizing these technologies with the precision and labor-saving capabilities of advanced algorithms, Horstmeyer's work is at the vanguard of a revolution in healthcare.
In the discussion below, Horstmeyer reflects on his interests in physics as an undergraduate at Duke, the value of his experience at MITRE and the view this gave him of on the world of science research and government contracting, and his initial graduate research at MIT's Media Lab and its culture of interdisciplinarity. Horstmeyer expresses gratitude that he had that MIT experience before his PhD work at Caltech, where he thrilled in a research culture that was so deeply focused on pure science. It was at Caltech that Horstmeyer became interested in biological questions, which led to an award-winning dissertation that investigated how computational advances could improve microscope resolution at the gigapixel level. This focus led Horstmeyer to conduct postdoctoral research in neuroscience at the Max Planck Institute before returning to the United States to join the faculty at Duke in Biomedical Engineering in 2018.
As a generational sign of the times, it was only as a new faculty member that Horstmeyer began to give serious consideration to the possibilities of artificial intelligence in biomedical engineering. With a solid foundation in optics, instrumentation, and applications in biology, the promise of AI was just starting to become relevant. Since then, Horstmeyer's approach has been to think broadly, and to develop technologies that could have wide applications. As both a hub for research, healthcare, and industry, Horstmeyer benefits from the multitude of collaborative opportunities available in the Research Triangle of North Carolina. And with a parallel approach that emphasizes improvements both to the instruments and to the algorithms, Horstmeyer's research future is bright, with ultimate benefit for patients and practitioners alike.
Interview Transcript
DAVID ZIERLER: This is David Zierler, Director of the Caltech Heritage Project. It is Tuesday, January 16, 2024. It is my great pleasure to be here with Professor Roarke Horstmeyer. Roarke, it is great to be with you. Thank you so much for joining me.
ROARKE HORSTMEYER: Thanks for inviting me.
ZIERLER: Roarke, to start, would you please tell me your title and institutional affiliation?
HORSTMEYER: My title is currently Assistant Professor of Biomedical Engineering and Electrical and Computer Engineering at Duke University.
ZIERLER: Is that two appointments in different departments or is that one overall title in one department?
HORSTMEYER: It's the former, two appointments in different departments.
ZIERLER: That leads me right to the big questions as they relate to your research. What is that point of contact for you between computation, biomedical engineering, and electrical engineering? How do you fit all of that together?
HORSTMEYER: I generally don't have a traditional home, and I think that's true for a lot of people in optics and optical engineering. That's really what I consider my core specialty or competency. Optics sometimes is in physics. Sometimes it's in electrical engineering. I'm primarily in biomedical engineering because I am currently really focused on making instruments for biosensing and biophotonics. Basically I make lots of different types of optical instruments, but I'm really excited about using them to study biology.
ZIERLER: How far back does that go in your career and your research? Have you always been interested in the intersection of biology and engineering?
HORSTMEYER: No. It goes back to my time at Caltech. Prior to Caltech, I didn't have so much experience working in the biological space or biotechnology space. I was making optical instruments or working on teams making optical instruments mostly to take images, starting with astronomy and then moving into imaging from above down to Earth. Then I got interested in small imagers for smartphones, before we had amazing cameras on smartphones. There was obviously a big push to make those better and do new and interesting things.
Optical Information and the Research Triangle
ZIERLER: Tell me about your work at the interface of optics and information. Are you using optics to optimize information, is it the other way around, or is it something else?
HORSTMEYER: Great question. Early on in my career, I joined a very small but now large group of people who work in the field of computational imaging. That is how it originally started. The field really tries to codesign the optics—the hardware that is taking the light and making it do things— and the software that is then processing the data after it has been collected. When you codesign or co-optimize those two things, you can really start to do new and interesting things with your instruments, your optical devices. A simple example of that is instead of having one camera, you could have two cameras, just like we have two eyes, and you can see things from slightly different perspectives and use that information with an algorithm to then create a 3D picture instead of just a standard two-dimensional picture. There are many other examples of doing things like that, that we work on.
ZIERLER: Is being in the Research Triangle—Chapel Hill and Durham and Raleigh—is that an asset for you? Do you have affiliations and partnerships beyond the Duke community?
HORSTMEYER: Absolutely, yeah! It's great here. I grew up in California, and so I know the Bay Area, I know the Southern California region. I've lived in Boston and D.C. I think the Triangle area is my favorite place so far. I really, really like it. I convinced my two brothers to move here. They're very happy here. So, yeah, we have a nice thing going, I think, down here in the Triangle! To answer your question about interacting more with the Triangle—yes. I have a startup company that is based in Durham. Through that, I've learned about people doing all sorts of other stuff in the industry side of things. There's lots of startup activity and interesting biotechnology commercial activity here. We also have collaborations at UNC and NC State, so there's also this research presence that goes beyond Duke.
ZIERLER: For the instruments that you build, what kind of interface do you have in a clinical or a medical environment? Do you work with doctors? Are you actually in the hospital working with patients?
HORSTMEYER: Great question. What is really nice about Duke Biomedical Engineering or just Duke in general is it is integrated with the Duke Medical Center. It's just right across the street. So, I work a lot with clinicians. Do I go in there and do the surgeries or look at the pathology specimens? No, but I watch people do it. I get invited to join in and see how things are working to try to help come up with better ideas for newer ways to take measurements, for example, or software that can improve their workflow. I work primarily with people in the pathology department, who are looking at specimen material from patients under microscopes, but also more and more so interacting with the people pathologists work with. The surgeons and others who are acquiring that specimen material, they also need a lot of optical instrumentation and software. They're looking towards AI-type algorithms to help them in that process. I also am beginning to work more and more with surgeons, specifically surgeons who use microscopes—it's called microsurgery—who really need better systems, better ways to see the body at high resolution to help them and help newer instruments that can be automatically controlled, or remotely controlled rather, achieve their surgical goals.
Clinical Interface
ZIERLER: What are the kinds of human health ailments that you're working on, on the technological side of things?
HORSTMEYER: A lot. It really is widespread. We have projects looking at cancer. We have projects that are trying to enable better diagnostic and prognostic methods for cancer. We did a project with COVID-19. Obviously that was a big push recently. We look at infectious diseases, that aren't so much here but in other resource-limited areas, like malaria and TB. Those specimens aren't coming from the Duke clinic but are coming from elsewhere in the world, and then we get them here in our lab and image them to try to come up with better diagnostics. It is really a whole wide range.
ZIERLER: In terms of inspiration or getting a project going, are you developing technologies and then you see where they might be applied, or are you starting from the medical perspective and listening to what the clinicians are needing and you're responsive to that?
HORSTMEYER: I call it having a hammer and going to find nails to hit, versus finding the right nails beforehand. I think this is true for a lot of engineers—and I studied physics, so we're coming at it from the physics and engineering standpoint—you always end up falling into the former. You say, hey, here's an idea for a new instrument, or a new way to measure something, and then you think, well, where could that be useful? It's I think a natural way to approach problems when you are trained from a physics and engineering standpoint. You have to fight that. I'm trying more and more to come at it from the other direction, where you listen, you hear what people need, and you then try to think holistically, a technology-agnostic standpoint, about what's the best way to help them achieve that. Often times the answer is, "I can't help you. You should go do this, or use this. I've heard of this. This would be way better than some instrument I could make for you." But sometimes you do find that perfect fit, and that's when things get exciting.
ZIERLER: In dealing with medical devices, does that involve you in the FDA and the broader regulatory environment?
HORSTMEYER: A little bit, yeah. We're really just starting to enter into that space. A lot of times when we're dealing with clinical projects, we have been primarily just doing them from a research standpoint, where we don't need to involve the FDA at all, but we do need to really be considerate of privacy and patient—essentially going through the human subjects review process with the Institutional Review Board, all of that. We have in my startup been making microscopes that are beginning to seem like eventual products within the medical and diagnostic space, so we're starting to talk and learn more about that FDA approval process, because of course when you make a commercial system, then it really has to be fully FDA approved before it can be sold.
The Biomedical Embrace of AI
ZIERLER: Of course these days everyone is talking about machine learning and artificial intelligence. Are you a user of these technologies, or are you actually developing algorithms that are optimizing AI and machine learning?
HORSTMEYER: Great question. I would say at this point I am more of a user, myself and my lab. We're advanced users. But starting seven or eight years ago now—2014, 2015 was when I really started getting—I had studied machine learning at Caltech, but then a couple years later really was excited by some of the amazing results that were being demonstrated with convolutional neural networks. I started using and wrote some papers about using machine learning to optimize optical hardware and optical imaging methods. That's something we still work on and are still interested in. It's not really a core focus of our research right now, but we continue to help others and are involved in collaborations in that space. What we've really done or tried to do is take machine learning and AI methodologies and apply them not just to train computers to say what's in an image, but to also train them to make better imaging devices or make better measurement hardware. This is sometimes referred to as physics-informed machine learning, or there are a number of different terms. We call it learned sensing. I would say we work in that space, where research in machine learning is still ongoing, and we're developing new tools.
ZIERLER: I can wrap my head around the software side of things, but what does it mean for AI to actually improve hardware, physical devices?
HORSTMEYER: Great question. When you capture an image with your eye, it's optimized to make a really sharp, perfect image, and we can understand what we're looking at. That's a great way to capture image data for us because we look at all types of things in the world and need to navigate and be in many different scenarios. If you're making a camera—or an eye, so to speak; a lens with a digital image sensor—just to do one thing, let's say look at blood cells and say is there malaria in that blood cell or is there not—you don't necessarily need to make a perfect image. It can be a blurry image. It can look a little strange. Maybe the color is different in a way that really pulls out that signature of is there malaria or not. What sort of lens should you make? What sort of color pattern would be ideal? It's not so obvious. But what you can do is ask the computer to try to figure that out. How should I blur this image, how should I change the color or appearance of this image in a way that would be captured with the new microscope I'm going to make, such that I can optimally tell the difference between cells with a malaria parasite and cells without? Why would you want to do that? A, you can get better performance than a regular camera, and B, it turns out that you can really work within different design spaces. You can make microscopes that can see a much, much larger number of cells all at once, or really start to pull out new features of cells that you otherwise wouldn't think would be possible when you're asking the computer to establish that new type of hardware to use to then capture the data in a unique way.
ZIERLER: Let's go back and establish some personal history. You mentioned it was physics that you focused on at Duke as an undergrad?
HORSTMEYER: Yeah, I did a double major in physics and Japanese.
ZIERLER: Where does the Japanese come in?
HORSTMEYER: In high school I took Japanese, and then before I went to college I took a year off, I took like a gap year—which wasn't called a gap year at that time; I guess now it's called a gap year!—and I went to Japan and I went to high school. I did an extra year of high school but as an exchange student. I stayed with a host family and went to high school there with a bunch of other Japanese people. That was a great experience, and through that experience I just really enjoyed learning Japanese, obviously, because I then wanted to continue to keep up with it, which the second major helped me do.
ZIERLER: Operating in a high-tech world, has that been an asset to you, having those Japanese connections?
HORSTMEYER: Not really [laughs], to be honest. No. Of course I run into Japanese people through what I do, but they're often just surprised that I can speak Japanese. I rarely really start speaking with them in detail with it, because it's just a little bit surprising and different, I think, than what you're used to.
ZIERLER: On the physics side, I'm guessing you were more on the experimental side of things, or were you interested in theory also as an undergrad?
HORSTMEYER: I think I was always interested in instrumentation. You have all of these amazing ideas in physics, but how do you actually measure them? How do you actually go about designing an experiment and then the actual thing to try to measure it? I think that experimental aspect but specifically the thing you would make was really what always fascinated me. That started very early on. Even in high school, I was lucky enough to get an internship at NASA Ames—
ZIERLER: Oh, wow.
HORSTMEYER: —for a summer. I think that's really where some of my real interest in these instruments started. I was working with a scientist who was able to collect data from the Hubble telescope to look at planetary nebulae and understand the gases in them. I was just really fascinated about how that actually could happen. Here is this computer I'm sitting at down on Earth, and we're able to have these measurements that are just acquired from way up there. Who designed that, and how did they even achieve that? That just really sparked my interest in that whole thing. So, I think during my studies of physics, I was always drawn in that direction.
MITRE Before MIT
ZIERLER: When it came time to graduate, what were you thinking? What were your options at that point?
HORSTMEYER: While I was an undergrad at Duke, I was really interested in education. I spent some of my time all four years teaching science, very part-time. I would come into maybe four different classes once a week to do a one-hour science lesson, ranging from third grade up to high school. I really enjoyed that, and so I considered doing Teach for America right after graduating. I—in my opinion—luckily wasn't accepted into the [laughs] Teach for America program. Then I was drawn to applying to jobs that were more aligned with my major. I did some research while I was an undergrad with—no longer here, but you had mentioned him—David Brady, and really got interested in not just making big telescopes and things like that, which is where I was more fascinated about optics during my studies, but real instruments on an optical table, like something you could actually make yourself. I worked in his lab making some small cameras and then a spectrometer to help some of his research efforts. Through that experience, I then found a job at a company called MITRE, which is a defense government-type research company that is based in Washington, D.C., and Boston.
ZIERLER: When did you start to think about graduate school?
HORSTMEYER: I think even when I took that job at MITRE, it was under the pretenses of I would apply to graduate school. The person who hired me at MITRE was just recently a professor and left academia to go work at MITRE. He said he really wanted to hire people who would use this job as a small research experience to then go on to graduate studies. So, from the beginning, I had told him, "Yeah, I honestly probably want to go to graduate school at some point," and he said, "Great, that's exactly what I'm looking for. I would encourage that." That job, which I only worked at for a year and a half, I think, really served as almost like a master's degree, and that's where I got into the computational imaging community. Learning all about it was through that experience.
ZIERLER: MITRE, of course, as a government contractor, who were some of the users in government for this work?
HORSTMEYER: It's a funny space. MITRE did work with users from the government. One part of that government space, was talking to those government customers, but something else that MITRE does is it helps manage grants. Specifically, my job at MITRE was closely intertwined with what was going on at DARPA. When DARPA makes an award to some groups at universities, the projects are ongoing and they need to be evaluated, progress needs to be tracked, and MITRE would help with that process. As scientists and researchers, we were able to communicate and distill how different projects were going and provide input back and forth to different research teams. Through that experience, I really got to learn about all these different labs that were doing great research. I was tasked with following the cool research coming out of labs. So, when it came time to apply to graduate school a year later, I knew already all the labs I wanted to apply to. It was easy! I could say specifically, yeah, I would love to work on this thing or that thing. I already knew what they were doing. It was a big leg up for me.
ZIERLER: It sounds like everybody should go work at MITRE before they want to go to graduate school! [laughs]
HORSTMEYER: Totally. I highly encourage that to some of the undergrads here who I speak with who are a little bit uncertain but are kind of thinking about graduate school.
ZIERLER: For all the labs you were exposed to, what was most exciting? How did it really refine the kinds of programs, the kinds of professors you wanted to work with in grad school?
HORSTMEYER: That's a good question. My most exciting labs that I remember, that I was like, "I really want to join this lab," one of them I did join. It was Ramesh Raskar's lab at the MIT Media Lab. There was another really great lab who—I think maybe he's retired now, but Marc Levoy at Stanford University, who was designing or making light field cameras and light field microscopes. I was really interested and excited about that. There were a few labs at like UT Austin that were doing really, really neat spectroscopy, exciting spectral measurements that I thought was cool. Rice University had a big effort in several groups that were doing something called compressive sensing, which was also exciting at that time. It really was a bit diverse, I'd say. To be honest, I did not look at Caltech. I didn't know too many labs at Caltech at that time. That came later. After I moved on to graduate school, I got more exposed to some of the research at Caltech.
Science in the Media Lab
ZIERLER: Tell me about the MIT Media Lab and why that was compelling to you.
HORSTMEYER: Oh, it was just so exciting. Especially I guess at that time, there were so many neat things coming out—I guess, still; [laughs] it hasn't really changed—that are really almost like—inspirational research, I could maybe use the term? Research for the sake of inspiring new ideas and new creativity, really pushing the boundaries of what is possible or what you might even consider as a potential device or use or algorithm.
ZIERLER: What does "media" mean in this context?
HORSTMEYER: I think the Media Lab, the name almost doesn't mean anything at this point. [laughs] The Media Lab I think spun out of CSAIL at MIT, the Computer Science and Artificial Intelligence Department, because it was at the interface of new things that weren't traditional research. Some key examples that were told to me, and I think they tell to everyone, was early on, they were putting cameras on cars because one professor was really excited about filming everything to establish a map of Boston, before computers could really even accept all of that data. So, like 30 years before Google Street View, envisioning the need and desire for that. I would say likewise, new ways to interface with computers that were really forward-looking. I think the term "media" really refers to any primarily digital content that would be ingested by humans. Looking forward, how can we think about designing better, newer, more interesting, more useful ways not just to interface with digital data, but to collect it, have algorithms process it? It's that ecosystem between the human, the world, and the computer, and how do you make a virtuous cycle between those three areas. I think media undersells or perhaps is a little bit of an older term that maybe doesn't best describe what most labs at the Media Lab are working on.
ZIERLER: Is it an independent department? Is it an interdisciplinary thing where professors from other departments get together? Administratively, how does it work at MIT?
HORSTMEYER: It's a fully independent department. It's its own building. Now it's two buildings that are connected. Most professors are solely appointed in the Media Lab, not cross-appointed. It is very interdisciplinary, so it's an interesting space. There are professors really who I would say are closer to art, artists. There's neuroscientists next to that, a whole wet lab space where people are really doing neuroscience experiments. And there's everything in between. It's pretty neat and really diverse, which has its pros and cons. Certainly one pro is that there's always something interesting going on, and there's always new things to learn about, so it is a great environment for exploration. A con is that there really isn't a core focus, so if you do want to really narrow down and become excellent at a smaller subdiscipline, then you have to go find those resources. They are not right next door.
ZIERLER: Was it a terminal master's for you? Could you have stayed on for the PhD?
HORSTMEYER: I could have stayed on for the PhD, yes. I joined with the intention of getting a PhD at the Media Lab, then after about I'd say a year, I kind of was thinking, well, maybe—partially because of that downside of not having this really focused effort, or struggling to find that focus I'd say perhaps, I started thinking about applying to other places. At the same time, I also got really interested in microscopy; just microscopy, period, not even biology at that point. I was working more and more with another lab at MIT, with someone else who went to Caltech, George Barbastathis, who was also in Demetri Psaltis's lab like David Brady. George and his lab didn't really make microscopes that much, but a few of his lab members were beginning to make microscopes that could do some cool things. I was really friendly with those students, and we would talk research all the time. We were writing papers together. I would go to their lab meetings. Through that, I increasingly got really interested in specific methods in microscopy. Obviously knew about Changhuei Yang and all of his research through that.
ZIERLER: Caltech pretty soon got on your radar. That was a place for you to go?
HORSTMEYER: Absolutely. At that point when I was like, I think I'm going to apply to some other programs for my PhD degree just to see if I get in anywhere else, because perhaps they might be a better fit, Caltech was top of the list.
ZIERLER: Looking back, was it valuable, do you think, to have that duality of that shorter time at MIT before going on for the PhD, or would you have been better served doing one PhD the whole way through at Caltech?
HORSTMEYER: No, it was incredibly helpful for me to have gone to the Media Lab and then Caltech, I think the main reason being at the Media Lab I learned, through mostly seeing and having all of these colleagues in other labs, that doing research isn't necessarily just about focusing and doing an experiment but you have to be able to communicate your ideas well, to not just other peers but ideally the broader world, and that you can be very creative in your research. Research really is an opportunity not to just focus more from a physicist's viewpoint on really getting a result or something, but it's really about exploring, coming up with new ideas that might sound kind of crazy at the beginning, but if you do that enough, one of them, or two of them if you're lucky, might hit and stick. I think if I hadn't seen all of the people at the Media Lab and learned about that kind of process through my experience there, I would have not had such a great time at Caltech—or I would have had a great time, but not as great a time as I did. I think it really opened my eyes a bit as to what I could achieve.
Caltech Before Medical Engineering
ZIERLER: The Department of Medical Engineering must have been either relatively new or not yet started. What was its status by the time you came to Pasadena?
HORSTMEYER: It didn't exist when I first came. If it did exist, I didn't know about it until maybe two years in, and it was just getting started. I think Yang had been appointed perhaps as a member of the Medical Engineering Department, but we didn't interact much with other members. Perhaps we did interact with other members of the Medical Engineering Department, but it wasn't under the pretenses of being in the Medical Engineering Department.
ZIERLER: Did you come to Caltech knowing who your thesis advisor would be, coming more as sort of an advanced student than somebody straight from undergrad?
HORSTMEYER: Oh, yeah, I came directly to Yang's lab. I applied to his lab, and then I flew out to meet him and see the lab. I wouldn't have gone to Caltech if he said, "No, you can't come," I don't think.
ZIERLER: What was Yang working on at that point? What was exciting?
HORSTMEYER: I think everything Yang does is exciting! But there were two or three projects that I thought were really cool at the time. One was this—optofluidics I guess is the term, or fluidic, let's say, is the easier way to explain it—having fluidic devices, microfluidics, integrated on an image sensor, so that you can flow and pattern whatever you want into these microfluidic chambers and image them directly and see what you're doing at high resolution. I thought that was great because it was this proposed low-cost, simple, but pretty powerful platform to see whatever you need to see, whatever will be helpful to see, while you're manipulating your experiments in these optofluidic chambers. The second was the ePetri device had just come out, and they were really trying to make progress on it. This was again a sensor without any lenses. You would put your specimen on and then use a LED array to shine light from different angles and capture a bunch of uniquely illuminated images to then process and get better resolution of or 3D information from. The third was focusing light deep in tissue. People call it different things now—wavefront shaping, or optical phase conjugation—but it was patterning a laser beam to then have it focus itself deep in some disordered scattering medium like human tissue or tissue in general.
ZIERLER: I'm always interested in the MIT and Caltech comparisons. Obviously it's apples and oranges given the uniqueness of the Media Lab, but what were your impressions of the differences in the research culture at both institutions?
HORSTMEYER: They're very different, especially the Media Lab. To put it simply, Caltech is really focused on science, pure science. A big win I think for any lab at Caltech or any graduate student at Caltech—a huge win, the biggest—is a paper in Nature or Science. In absence of that, which is not to put too much emphasis on publications in these certain journals, a big win at Caltech is an achievement that is viewed by the scientific community as a breakthrough by their peers across all of people doing science. That really, it seems like to me, during my time at Caltech, let's say 90 percent of the focus is really on that. It's really important to heavily weigh that goal or to have that goal, hopefully. At MIT, specifically, certainly the Media Lab, that generally was not a goal. A few people had that goal, but not the majority of people. The majority of people at the Media Lab really had the goal of capturing the attention of the world, not just scientists but everyone, and showcasing how technology can really be transformative, and showing how it can lead to these paradigm shifts, not just in science but across the board. I would say that also outside of the Media Lab, really that balance between science focus and world focus was a little bit different.
What manifests itself in that difference is at MIT—it is a much larger school, so it's sort of unfair to compare, but there's a bigger emphasis on commercialization, spinning out different technologies. It might not be the coolest science, but hey, it might lead to a great startup, and so you should really, really focus your attention on that, and here, go talk to the business school because they're going to help you do that, et cetera. That's a big difference, I would say.
LEDs and the ePetri Project
ZIERLER: Did you come in with a good idea of how you would slot in, in the lab, and ultimately what your dissertation research would be?
HORSTMEYER: It changed pretty quickly. I came in and was assigned by Yang to help with those projects I mentioned, to pick and find a way to push forward. I started off making a digital optical phase conjugation setup, which was very challenging. [laughs] I did it once then and it took me a long time, and then I ended up doing it a second time during my postdoc, and it was much faster but still very time consuming. Through that experience, I really got great training in hardcore optics aligning and precisely doing experiments. During though that initial time, we came up with an idea to not just focus light in tissue—or rather, when you shine light in tissue, it really scrambles light and makes an interference pattern that looks like a kind of speckly appearance. People call it speckle. We came up with an idea to use that speckle for cryptography, for purposes of information security. So, after just maybe six months of being there, my project trajectory shifted towards that. We got really excited about that. So, it kind of quickly diverged from what I was originally signing up in the lab to work on, to a new project area.
At the same time I was working in that scattering space, I was also helping with this ePetri project, shining light from different LEDs to capture different images. Guoan Zheng, who now is a professor at Connecticut, and I worked a lot together, informally. He and I really shared this "Hey, let's just try it" mentality of doing weird and crazy experiments just to see what happens. We were both almost obsessed with making a picture with a billion pixels, a gigapixel. We were using the ePetri to try to help do that, and through that whole process we came upon a new technology, Fourier ptychography, which then became the second half of my PhD or almost the majority of my PhD. I came in with certain projects in mind, but thanks to Yang and the team and everyone there, I was given enough flexibility to explore and come up with new, interesting ideas, try new things out, and that led to a couple things that defined my thesis.
ZIERLER: You mentioned it was really at Caltech where your interests in biology blossomed. Caltech obviously does not have a hospital, and it kind of celebrates that. It's sort of liberating. That's the idea at Caltech—we don't have a hospital; we don't need to deal with that. How do you square that circle? How does that make sense, to not have any of that clinical interface but to do things that are so bioengineering-forward?
HORSTMEYER: With Yang, we would collaborate with the City of Hope, which wasn't too far. We would drive out there. It takes 30 minutes or something. Apart from the clinical aspect or clinical focus use cases, we were just focused on biological use cases, even for research, researchers just down the hall or in the Biology Department who needed better tools. We would go over and say, "Hey, how are you imaging your cells? What sort of workflow?" Not that they cared about the instrument; they just knew they could capture pictures of their cells and then get the data they needed on cell growth or death. We went to them and said, "Hey, we can probably do that, but we can do it x times faster," or, "You don't have to stain your cells and look at them after they're dead; we can do it while they're living, using the innate contrast of the cells itself." At Caltech, we were targeting our design and exploration around the needs of biologists in research, not the clinic.
ZIERLER: When did your dissertation feel done? When did you feel like you had reached a point of conclusion or completion?
HORSTMEYER: That's a good question.
ZIERLER: Did your advisor tell you it was time to move on? Is it as easy as that?
HORSTMEYER: [laughs] No. It was more that my wife—we weren't married at the time—we were ready to move on to the next thing. It wasn't like Yang said, "Hey, you gotta get out of here, move on." I think he was happy having me. I think generally, as an assistant professor now, the longer you can keep students, the better [laughs], because they're trained, and they're great, and they're doing excellent research by the time they're in their later years as compared to the beginning. So, I didn't feel the pressure to leave at all, but I did feel like, okay, it's time. I had been in grad school I think six years or something at this point. It seemed like time to move on. My wife now and I were really excited about traveling. Right around the time I think I said "Hey, I think I'm ready to graduate" and started really putting my thesis together, I had gotten a job offer from a former postdoc in Yang's lab, Benjamin Judkewitz, to go to Berlin. I think looking back, probably the sequence of events was getting an email from Benjamin saying, "Hey, you have a job now in Berlin. We got this funding for you. You have to come soon." Then I said, "Okay, well, now I have to write my thesis and move on." [laughs]
ZIERLER: I'm curious how your time at Caltech informs your approach to collaborations. Not having formal training in biology but working so deeply in that field, when is it right for you to hit the books and learn the material yourself, and when do you outsource that through collaborations, where you accept that you're not the expert and you need to work with people who are specifically trained in that particular area?
HORSTMEYER: For biology and clinical work, I always work with experts. I never claim to be the expert. I think it's so important to do that, not just to make sure you're doing good science, but also to really learn and keep exploring and find new and exciting things to work on. The more collaborations and people you can talk to who are in biology but not experts at, let's say, the data science and information collection aspects of what they're doing, or the hardware instrumentation side, who need that input, it's really a perfect marriage, I think, so I really am open to that. When I do work with biology, biological specimens, it's almost always as a proof of concept. It's to say, hey, look at this, we tried this out and this is what we see. Is this enough to get some actual science started, some real experiments started, with someone who is an expert at that?
Neuroscience in Berlin
ZIERLER: I don't know what the chronology is here—the Chen Institute for Neuroscience at Caltech, was that up and running? Did you have any partnerships there? Were you interested in neuroscience during your time at Caltech?
HORSTMEYER: I was, and no, it wasn't. For my postdoc—Benjamin is a neuroscientist, so his lab in Berlin where I did my postdoc was a neuroscience department. At Caltech, I was slowly drawn into the neuroscience space—and honestly knew very little at that time—through some collaborations Yang had where, A, we were getting neuronal cultures, just on glass, to image with our Fourier ptychography microscopes, and then B, where we were trying to focus light into the brain of model organisms, so with that phase conjugation type system, which Benjamin was working on heavily. I worked with Benjamin just collaborating with him in the lab, but we were more like friends, so it was really through the friendship with Benjamin and a few of the others that I learned about neuroscience and how important imaging was for neuroscience. I really learned the bulk of what I know about that space, which is still very little, in my postdoc later.
ZIERLER: What was your home institution in Berlin?
HORSTMEYER: In Germany, things are very complicated in terms of institutions. They are all interconnected. I was officially in the Charité medical school. It is the biggest hospital in Berlin and the biggest medical school in Berlin, one of the bigger ones in Germany. Charité has a Max Planck inside of it, or next to it, I guess. Physically, the Charité complex has a Max Planck Institute there, and what's called the NeuroCure, which is a neuroscience-focused institution with a number of labs. The complexity comes in that my affiliation as a researcher was through Humboldt University, which is a very big university in Berlin that simply sponsored my postdoc fellowship. I really didn't have any other interaction much with Humboldt University. I would say I technically was a member of Humboldt University but in all practical senses worked at the Charité medical school.
ZIERLER: Was neuroscience your main focus during your postdoc?
HORSTMEYER: Pretty much, yeah. During that time, we were focused on two main projects technically, and then—well, kind of three, but two that were very neuroscience focused, which I can explain in a second. Similarly at that time was when I was really beginning to use deep learning methods and apply those to design, or try to design new imaging acquisition methods or hardware, using the machine learning algorithm to say, "Hey, you should use your microscope like this, or illuminate the specimen like this, because that will yield better results." I think indirectly that interest in machine learning and deep learning connected with—or I was connecting with—a lot of the theoretical neuroscience students who knew about neural networks and how they worked. So, I would say holistically, yes, I worked on neuroscience. Some of it was actually experimental neuroscience, and some of it was more neural network science, learning how those worked. The neuroscience imaging projects were twofold. One was to, again, work on this effort of focusing light deep in tissue, but now with the goal of scanning a beam around to form an image in a living organism—which was really hard, not surprisingly, to do, but not just myself, primarily other students in the lab, really came up with some great ideas with, and I was there to help with that. The second goal, which we still are working on today, was to image neutral activity in freely moving organisms. The main organism that we're focused on is the zebrafish, but we've also worked with fruit flies.
ZIERLER: What is freely moving? What's not a freely moving organism?
HORSTMEYER: Something that is anesthetized or constrained or paralyzed. Ninety-eight percent of neuroscience imaging experiments, or experiments in general, are under those three buckets.
ZIERLER: The value there is to interact with a specimen in its quote-unquote natural state?
HORSTMEYER: Natural state is important. You can study new things like social interactions or motor behavior. Interesting behaviors in the zebrafish that we're trying to look at are like hunting, exploration, and what are the neural signatures of that. It's really difficult to figure that out when they're constrained, anesthetized, or paralyzed.
ZIERLER: Did you enjoy your time in Berlin?
HORSTMEYER: Yeah, I loved it. It was great. It's a really fun city, and it was really nice to live there for a couple years in a new country, new place. I recently went back. I go back once in a while. I hadn't gone back during the pandemic, and I went back about a month ago. Even though it was the dead of winter and really dark and kind of dreary the whole time, I still was like, ‘Oh, wow, this is a great city." I like it there.
ZIERLER: To go back to the beginning of our conversation where you were explaining your research agenda, which is quite a bit more expansive than neuroscience, did you have that perspective when you went on the job market? Did you not want to focus narrowly on neuroscience for the kind of research you would do as a professor?
HORSTMEYER: Yes. I didn't feel like an expert in neuroscience at all. I still don't. It's such a challenging discipline. I have a huge amount of respect for people who work in neuroscience. It's really pushing the frontiers of what we know, and there's so much that we don't. To make scientific progress in that context, with the challenges that biology always presents experimentally, it takes a lot of brilliance, patience, and hard work. I certainly was not trained at all as a neuroscientist and didn't want to focus my research solely in that direction, but I knew, from my time as a postdoc primarily, how useful some of the tools we were making could be for neuroscience. I really wanted to continue that. I saw a very clear pathway to helping experimental neuroscience out or trying to really push what was possible in current neuroscience experiments.
ZIERLER: What about your appreciation of the utility of machine learning as a biological tool in general, beyond neuroscience? That was a major focus of your time in Berlin. Was that part of the faculty vision that you had in job talks and things like that, thinking about AI more broadly in biological engineering?
HORSTMEYER: No, it was not, but it should have been!
ZIERLER: [laughs]
HORSTMEYER: If I had applied later. I was in a funny scenario, which I think is kind of uncommon [laughs]. The chronology of that time is a little fuzzy, but when I was graduating from Caltech, I like I said was motivated to write my PhD and graduate to then go to Germany and live in Berlin and enjoy that experience. I was definitely dead-set on that. But I was invited to apply to a couple faculty positions. I think the timing was let's say I actually finished my thesis in October and was hoping to go to Berlin in December or January or something, and that's right when all of those job applications are due for faculty positions. I was invited by several colleagues, too, who I really respected and didn't feel comfortable saying no to, I guess. Like it would have been rude to say, "Hey, I'm not going to apply," after being invited by someone I really thought was a smart and important person. So I said, "Okay, I'll apply." Then I had to put all of that material together. It's not a light ask to [laughs] put together an application for faculty positions.
Because I spent many hours writing all of those essays and making my CV all pretty and everything else, I said, "Well, I'll apply to a few other places." I ended up applying I think to like six programs, which I think usually people apply to a lot, but I said, "Okay, I'm just going to apply to places I really would move to, I really want to go." Or my wife and I, rather, we collectively picked those places. I didn't just say, "Hey, we're going to move here." One of the places that made that list was this area, Durham and Chapel Hill, because my wife and I met here—we were both undergrads at Duke—so we knew it and we liked it, and we said, "Yeah, we would definitely move back there."
I applied to both UNC and Duke. Lo and behold, I think right before moving to Germany they said, "Hey, we want to interview you." So, really early on, before I had even started by postdoc, I came to interview both at UNC and Duke. Then we went to Germany and then I got asked back for a second round of interviews. They said, "Hey, you have to come back." I had to fly back and interview at Duke. Then they said, "Hey, you got the job." So, right when I was beginning my postdoc, I got the job offer at Duke. I was not even doing the neuroscience or machine learning experiments yet. The research I was proposing, I don't even remember, to be honest. It was not anything I'm doing now, I'm pretty sure. It then required me to say, "Hey, I'm not going to take this job unless I can spend two years here in Germany. I want to delay things to really enjoy that time and be able to do great research here." They said "yes," luckily. Duke said, "Sure." I was able to really enjoy my time there, because I wasn't worried about a job afterwards. I had already gotten this job here at Duke and could just focus on the research there as opposed to having this nagging, oh, I've got to apply, and come up with all this new material and stuff.
Early Days in Machine Learning and Image Analysis
ZIERLER: Given that your memory is fuzzy on what you proposed to do, how quickly did you pivot once you joined the faculty at Duke to what you're doing now, essentially?
HORSTMEYER: Immediately. Right when I came to Duke, I said, "Hey, I'm working on machine learning. I want to teach a machine learning course. I think it's great—machine learning for image analysis." Duke said that would be a great course. I hired students to help work on some of the machine learning projects I was pursuing. At the same time, these microcamera array microscopes that my lab is working with a lot, that the startup here is developing, that was immediate as well. I said, "Hey, this is also going to be something that I'm going to be doing for my research here at Duke," with some collaborators that I already knew before actually coming here physically.
ZIERLER: Tell me about getting your research program up and running. What was most important for you?
HORSTMEYER: Just getting it up and running period [laughs], that was important.
ZIERLER: Building the lab, funding sources, graduate students?
HORSTMEYER: In hindsight, I got lucky, but recruiting good students and postdocs and just a good team, and coming up with a really solid dynamic lab culture, is great. It's really important. I think I did focus on that early on, and it definitely paid off. I think I got lucky in finding the people I did find to hire who have done really great work. Then also trying to maintain a happy lab culture and having people feel the ability or find ways to be productive in that space. Funding was something I also had to do and start to apply to. It wasn't a big focus of mine at the beginning but kind of became more of a focus. Honestly, I think you can be too much focused on funding. It's sort of a never-ending hamster wheel. If you bring in a lot of funding, then you need to keep bringing in a lot of funding to keep everyone and grow, et cetera. It kind of never ends. I've tried to come up with a really healthy and gradual, slow and steady I'd say, funding application process. I think actually starting and getting research out the door for what I do is a lot easier than other people in biomedical engineering. In other words, if I had a lab that was doing CRISPR on new cell lines and new techniques like that, it takes a long time to create the infrastructure to start to do experiments, to then get those first papers and results out the door. Building microscopes is much quicker. Writing software could be much quicker, more flexible. So, I didn't struggle at all in getting research started.
ZIERLER: Your graduate students, what kinds of disciplines do they come from? Is it as eclectic as all the things that you work on? Do you have chemistry, physics, biology, CS undergraduates, the whole range?
HORSTMEYER: Yeah, it's pretty diverse. We have a lot of physics, electrical engineering. I have two—actually, we were talking about this recently at my lab meeting—two of my PhD students studied statistics in undergraduate and then went on to get a master's degree in something else, but that really informed their experience in machine learning and machine learning method development. A little bit of biology. Like I said, we're not experts at the biological aspect—we find that through collaboration—but I do have one biology student in the lab to answer all of those tough questions.
ZIERLER: When you were surveying all of the areas of human health that your lab is focused on, which is so extraordinarily wide-ranging—from virology to cancer to neuroscience—did you appreciate that at the time? Did that all become apparent quickly, or was it more a slow process of building collaborations and you realized that your lab had these capacities that could be applied in all of these different areas?
HORSTMEYER: It's really the latter. We settle on these projects that have really wide-ranging clinical, diagnostic, prognostic, or even scientific applications, through that process of finding those perfect fits. I speak to people at the medical center all the time. I speak to other researchers as much as I can, to hear their problems, to hear the scenarios they're struggling in. I've learned that many times you just have to say, "No, I'm sorry, this isn't a great fit," but when there is a good fit, that's when you really say, "We have to pursue this. This seems like something we should at least test out or try out." It's through those ongoing conversations, constantly talking and hearing from others what's needed, that I have established a diverse application space. From a technology perspective, there is a common core. There is this central expertise in measurement, software, process that we work on, that is helpful in those diverse spaces.
Attacking COVID with Algorithms
ZIERLER: When COVID hit, besides the attraction obviously of being able to keep your lab open—I assume there was that exemption for you—what was your point of entrée in virology? How could you contribute to COVID-19 research?
HORSTMEYER: Again, it was this kind of crazy experimental thing, that we said, "Hey, let's just try it." I already had collaborations with several pathologists and one hematologist at Duke. I think we were working on some project. We had been working on looking at some blood specimens with them to help with a few diagnostic questions, se were already working with blood specimens from these clinicians. When COVID hit, here at Duke at least, very early on—and it didn't come out until later I think—but very early on here at Duke, the people I was working with were convinced about the importance of blood in the disease. This now is well recognized, that COVID virus particles are highly prevalent in platelets. They would attach themselves to platelets. A lot of long COVID symptoms are connected to blood disorders and things like this. We said, hey, can we use machine learning to try to figure out something about COVID-19 in the blood? Because we really have no idea what's going on.
We came up with a simple hypothesis to set out to answer, almost as like a fun project. Fun in a technical point; it was of course not a fun thing in the context of COVID-19 being a terrible disease. We said, hey, can we establish if a patient has COVID simply from looking at their blood? Nothing biochemical about the blood itself, but just its morphology. Can we see if the blood cells are actually changing in appearance somehow due to COVID-19? It was pretty easy to test that question. After getting an IRB, of course, we said, hey, let's take images of patients' blood who have later on—we verified—had COVID-19, and then ones who later on were verified to not have COVID-19. Let's then see if a machine learning algorithm, just from those images, can tell those two cohorts apart. Given a set of images of some blood cells, can the algorithm say, yes, this person does have COVID-19, and no, it does not? If the algorithm is able to do that, then we would guess that there is some morphological change in the blood. If not, then, oh, well, we tried, but the hypothesis we can say is probably negated.
We did that, and it worked! [laughs] The algorithm was pretty good at figuring out if a patient did have COVID or not, just from pictures of the blood cells. We were really surprised by that. The next question was, what is changing? What exactly is happening in the blood? That being the real central goal, because then if we were able to establish that, we were able to use artificial intelligence to do something that a human couldn't get, because no one in the medical community really knew which type of white blood cell is being impacted more or less by COVID, or morphologically what is changing in the cells due to COVID. Is the nucleus getting bigger? Are some specific white blood cells getting eaten up and the cell wall is deteriorating, becoming smaller, or whatever it is? We worked really hard to try to answer that question, and we did not succeed.
It would be interesting to return to that question now, given how much AI methods, machine learning methods have advanced, but at that time, the methodology to use machine learning to transparently inform us of what is important in the data were pretty in their infancy, so it didn't do a great job. The best we could do was having the algorithm—and it worked really robustly—tell us which type of blood cell is more or less important for the diagnostic. Those blood cells—at that time, right when we were doing this—were coming out in the literature as, "Yes, these are the important blood cells. These are the cells that are being impacted." So, our results did corroborate with what was being clinically suggested at the time, which was exciting, too.
Better Microscopes and Better Diagnostics
ZIERLER: We'll bring the conversation right to the present. What are you working on these days? What is exciting to you in the field?
HORSTMEYER: We continue to work on better machine learning and microscope methods for pathology. What is exciting, I think, is this push to move pathology and diagnostic towards intersurgical—into the operating room, into the OR suite. What are newer imaging and software methods that could be used while a patient is on the table to better inform what the doctor should do next? I think that's a really exciting space. It's in its infancy, and there are a lot of challenges obviously involved with coming up with new hardware that actually fits in a clinician's workflow, a doctor's workflow, but we're excited about that. We have a couple projects in that space.
I would say at the same time that that's happening, there is this other interest in the medical community in automated robotic methods for surgery and all sorts of other operations. As you envision the medical suite of the future, having imaging as part of it and robotic systems as part of it, those need to talk to each other. There's no way around that. So, how do you think of this from a more holistic standpoint, of how can we begin to think of imaging, and with these robotic systems in mind, create an environment that is as useful and efficient for all the surgeons and the other clinicians to improve patient care? That space I think is really new, really exciting, and we are just starting to make progress in coming up with ideas and thinking about that.
ZIERLER: Now that we've reached the present, for the last part of our talk I'd like to ask a retrospective question about your career, and then we'll end looking to the future. Of course what brings us together is Caltech. What has stayed with you from your Caltech days? Your approach to the science and the engineering, collaborating, knowing to ask the most important questions, being able to figure out the most relevant technologies to answer those questions.
HORSTMEYER: First and foremost I think what I loved about Caltech was that point I was saying that was different than MIT. It was really focusing on science for science's sake. I think something I've noticed that is different also at Duke here is not necessarily having that central tenet so instilled across everyone's research goals and trajectories, throughout at least the departments I'm in. I learned that there is such a value in at least—not just for everyone, but also just to make progress in research—in defining a hypothesis, setting up a way to test that, and just proceeding, and doing that kind of like clockwork. Having that be instilled in your approach to research, that has served me very well. I focus on that in training my PhD students and postdocs and undergraduates. It's really a focus on that scientific process that allows you—it's not just there because someone said it's a good idea; it's there because it actually is a great way to slowly make progress in a way that's quantifiable and tractable and you can turn back and look and all these other things. I think that is something that I love Caltech for. I don't know any other place that I could have learned that better, and I'm very happy about that.
Specifically in Yang's lab, I learned also how to be the technologist at this interface of biology, to serve almost as if the biologists are customers. Because they need help. It's really true. There are so many important challenges in the clinic but also the modern biology lab, and to really figure out where you can help that process is a skill set. It's not just, "Hey, take this technology and use it because I say it's good." It's more about a conversation. I really learned that from Yang and his team, and that served me well.
ZIERLER: As you chart the rest of your career, because you're so focused on human health and health outcomes, all of the technologies that you utilize, all of the different sicknesses that you're focused on, do you have an amalgam or a unifying vision for how you want to contribute to better patient outcomes regardless of the particular technology or the particular illness? Is there some overarching goal that puts it all together for you?
HORSTMEYER: Yeah. Honestly, for me, it is mildly technically driven, but I just see the benefits of automation and the assistance that software can bring to analyzing large complex datasets, which really are the foundation of the cutting edge of clinical and biological work today. I think people say, oh, there's this artificial intelligence effort and machine learning that is going to be evil and be this thing we need to consider as a separate entity. I think of it as an extension of us, and a tool we're able to use, but only when it is helpful to us. I am motivated by how we can create better methods and tools to leverage these amazing gains in algorithms that can handle the huge amounts of data and measurements that clinicians are expected to obtain and use, and that scientists are expected to obtain and use, to gain new insights. It's too much, and they really need help with that. What are ways we can streamline that process? What are ways that we can help that interface to improve their lives? Not just the patients' lives but the clinicians' lives, the scientists' lives, and then improve human health in the process, because that's what those people are working on.
ZIERLER: Well, what can I say? Good luck! We're all counting on it, right? [laughs]
HORSTMEYER: [laughs] Thanks, thanks. We're trying!
ZIERLER: Roarke, this has been a wonderful conversation. I want to thank you so much for spending this time with me.
HORSTMEYER: Yes, thanks to you!
[END]
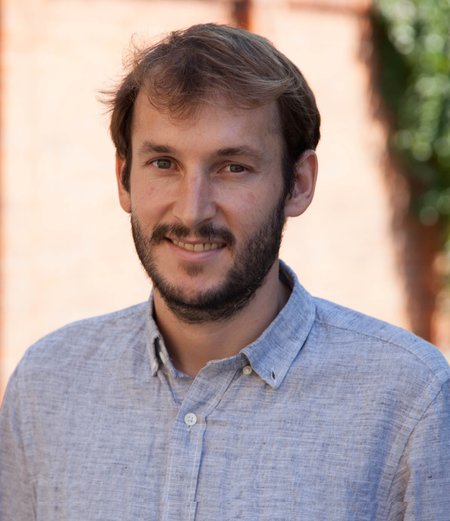
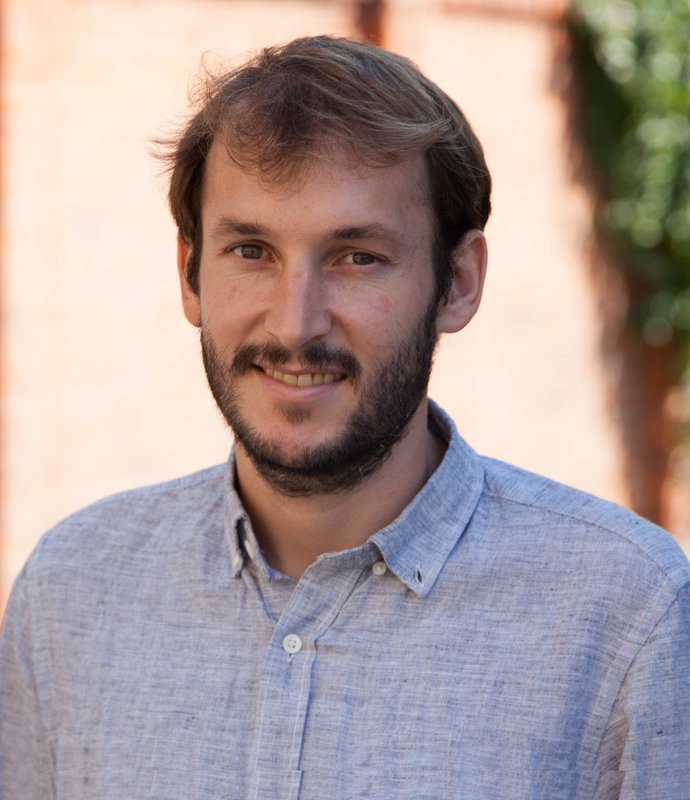
Interview Highlights
- Optical Information and the Research Triangle
- Clinical Interface
- The Biomedical Embrace of AI
- MITRE Before MIT
- Science in the Media Lab
- Caltech Before Medical Engineering
- LEDs and the ePetri Project
- Neuroscience in Berlin
- Early Days in Machine Learning and Image Analysis
- Attacking COVID with Algorithms
- Better Microscopes and Better Diagnostics